matlab中Npop = size(population,3)是什么意思
时间: 2024-05-31 12:10:16 浏览: 14
在MATLAB中,`size`函数可以用于获取数组的维度大小。`population`是一个三维数组,`Npop`是一个表示第三维大小的变量。具体地说,`Npop`的值等于`population`数组的第三个维度的大小,也就是该数组中包含的矩阵数量。换句话说,如果`population`是一个`m`行、`n`列、`k`个矩阵的三维数组,则`Npop`的值为`k`。
相关问题
matlab中改进粒子群算法引入交叉变异应该加哪些代码
改进粒子群算法(Improved Particle Swarm Optimization, IPSON)通常不需要引入交叉操作,因为它已经融合了遗传算法的思想。但是,可以考虑加入变异操作来增加算法的多样性和搜索能力。
在 MATLAB 中实现变异操作通常需要以下几个步骤:
1. 随机选择种群中的一个个体作为变异个体。
2. 随机选择该个体的某个维度进行变异,例如将该维度的值随机增加或减少一定的量。
3. 将变异后的个体与原个体进行比较,选择更优的一个作为变异后的结果。
具体的代码实现可以参考以下示例:
```matlab
function newpop = mutation(pop, pm)
% pop: population matrix (nPop x nVar)
% pm: mutation probability
% newpop: new population matrix (nPop x nVar)
[nPop, nVar] = size(pop);
newpop = zeros(nPop, nVar);
for i = 1:nPop
if rand() <= pm
% select a random dimension for mutation
dim = randi(nVar);
% mutate the selected dimension
newpop(i, dim) = pop(i, dim) + randn() * 0.1;
else
newpop(i, :) = pop(i, :);
end
end
end
```
其中,`pm` 表示变异概率,`randi(nVar)` 用于随机选择变异个体的一个维度,`randn()` 用于生成符合正态分布的随机数。此处的变异操作是对每个个体的某个维度进行随机扰动。
下面这个代码报错了,应该怎么改: %%Matlab Genetic Algorithm for Sin Prediction clear; clc; %population size Npop=50; %create the population Pop=rand(Npop,1)*2*pi; %define fitness fit=@(x) sin(x); %fitness score score=fit(Pop); %maximum number of generations maxgen=100; %weights w=0.7; %probability p_crossover=0.9; p_mutation=0.2; %loop for number of generations for gen=1:maxgen %ranking %rank the population in descending order [~,rank]=sort(score); %rank the population in ascending order rank=flipud(rank); %normalised rank NormalisedRank=rank/sum(rank); %selection %cumulative sum of the normalised rank cumulativeSum=cumsum(NormalisedRank); %randomly select the two parents %from the populations based on their %normalised rank randnum=rand; parent1=find(cumulativeSum>randnum,1); randnum=rand; parent2=find(cumulativeSum>randnum,1); %crossover %randomly select the crossover point pc=randi([1 Npop-1]); %create the offsprings offspring1=[Pop(parent1,1:pc) Pop(parent2,pc+1:end)]; offspring2=[Pop(parent2,1:pc) Pop(parent1,pc+1:end)]; %perform crossover with a probability if(rand<p_crossover) Pop=[Pop; offspring1; offspring2]; end %mutation %randomly select the point of mutation pm=randi([1 Npop]); %mutate the value under the chosen point Pop(pm)=rand*2*pi; %perform mutation with a probability if (rand<p_mutation) Pop(pm)=rand*2*pi; end %evaluate new population score=fit(Pop); %elitism %sort the population in ascending order %of their fitness score [score,rank]=sort(score); elite=Pop(rank(1),:); Pop(rank(Npop),:)=elite; %replace old population Pop=Pop(1:Npop,:); end %print the best solution disp('Best Solution: '); disp(elite);
I'm sorry, I don't understand your question. Can you please provide more information or clarify what you're asking?
相关推荐
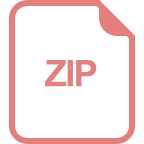
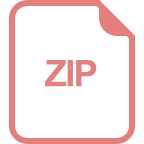
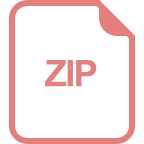












