python variance-covariance matrix怎么算
时间: 2024-05-05 19:17:39 浏览: 18
对于您的问题,可以使用 NumPy 库函数 numpy.cov 来计算 Python 的方差-协方差矩阵。具体实现方式如下:
首先,将含有数据的 NumPy 数组作为参数传入 numpy.cov 函数中:
```python
import numpy as np
data = np.array([[1, 2, 3], [4, 5, 6], [7, 8, 9]])
covariance_matrix = np.cov(data)
```
其中,data 表示包含数据的 NumPy 数组,covariance_matrix 变量存储有计算后的方差-协方差矩阵。
如果需要计算行的方差-协方差矩阵,可以选择使用 rowvar 参数。将其设置为 True,即可完成行的方差-协方差矩阵的计算:
```python
import numpy as np
data = np.array([[1, 2, 3], [4, 5, 6], [7, 8, 9]])
covariance_matrix = np.cov(data, rowvar=True)
```
希望对您有所帮助。
相关问题
variance-covariance matrix怎么计算
方差-协方差矩阵(variance-covariance matrix)用于描述多维随机变量之间的方差和协方差关系,常用于多元统计分析和金融风险分析等领域。
对于 $n$ 维随机变量 $X = (X_1, X_2, ..., X_n)$,其方差-协方差矩阵 $S$ 的元素 $S_{i,j}$ 可以计算如下:
$S_{i,j} = cov(X_i, X_j) = E[(X_i - E(X_i))(X_j - E(X_j))]$
其中,$E(\cdot)$ 表示数学期望,$cov(\cdot)$ 表示协方差。
同时,我们可以使用矩阵的形式来表示方差-协方差矩阵 $S$,即:
$S = \begin{bmatrix}
cov(X_1, X_1) & cov(X_1, X_2) & \cdots & cov(X_1, X_n) \\
cov(X_2, X_1) & cov(X_2, X_2) & \cdots & cov(X_2, X_n) \\
\vdots & \vdots & \ddots & \vdots \\
cov(X_n, X_1) & cov(X_n, X_2) & \cdots & cov(X_n, X_n)
\end{bmatrix}$
其中,对角线上的元素是方差,非对角线上的元素是协方差。
在Rstudio中使用dataframe计算variance-covariance matrix
可以使用R语言中的var和cov函数来计算variance-covariance matrix。假设你的数据框名字为df,代码如下:
```R
# 计算变量的方差
variance <- var(df)
# 计算变量之间的协方差
covariance <- cov(df)
# 输出variance-covariance matrix
var_cov_matrix <- cbind(variance, covariance)
print(var_cov_matrix)
```
其中,var函数用于计算每个变量的方差,cov函数用于计算变量之间的协方差。最后用cbind函数将两个矩阵合并为一个variance-covariance matrix。
相关推荐
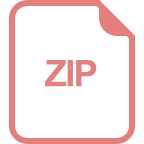
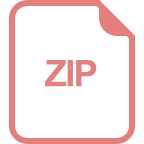












