In-depth Analysis of Autocorrelation Function: A Comprehensive Interpretation of Theory and Applications
发布时间: 2024-09-15 17:55:33 阅读量: 13 订阅数: 46 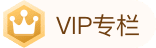
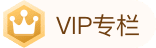
# 1. Theoretical Foundation of the Autocorrelation Function
The autocorrelation function (ACF) is a mathematical tool used to measure the correlation between observations in a time series that are separated by a certain time interval. It is widely used in fields such as signal processing, image processing, and time series analysis.
The definition of the autocorrelation function is as follows:
```
ACF(k) = Cov(X(t), X(t+k)) / Var(X(t))
```
Where:
* `X(t)` is the time series
* `k` is the time interval
* `Cov()` is the covariance
* `Var()` is the variance
# 2. Calculation Methods of the Autocorrelation Function
There are mainly three methods to calculate the autocorrelation function:
### 2.1 Direct Calculation Method
The direct calculation method is the most intuitive approach, with the formula as follows:
```python
def autocorr_direct(x):
"""
Direct calculation of the autocorrelation function
Args:
x: Input signal
Returns:
Autocorrelation function
"""
n = len(x)
result = np.zeros(n)
for i in range(n):
for j in range(n - i):
result[i] += x[j] * x[j + i]
return result / n
```
**Line-by-line code logic explanation:**
* Line 1: Define the `autocorr_direct` function to directly calculate the autocorrelation function.
* Line 3: Get the length `n` of the input signal `x`.
* Line 4: Initialize the autocorrelation function result array `result`, with a size of `n`.
* Line 5-7: Use a nested loop to traverse the signal and calculate the autocorrelation value for each time shift `i`.
* Line 8: Divide the autocorrelation value by the signal length `n` to obtain the normalized autocorrelation function.
### 2.2 Fourier Transform Method
The Fourier transform method utilizes the properties of the Fourier transform to calculate the autocorrelation function, with the formula as follows:
```python
def autocorr_fft(x):
"""
Fourier transform method for calculating the autocorrelation function
Args:
x: Input signal
Returns:
Autocorrelation function
"""
n = len(x)
X = np.fft.fft(x)
result = np.fft.ifft(np.multiply(X, np.conj(X)))
return np.real(result)[:n]
```
**Line-by-line code logic explanation:**
* Line 1: Define the `autocorr_fft` function, which uses the Fourier transform method to calculate the autocorrelation function.
* Line 3: Get the length `n` of the input signal `x`.
* Line 4: Perform a Fourier transform on the signal `x` to obtain the frequency domain signal `X`.
* Line 5: Calculate the product of the frequency domain signal `X` and its conjugate `np.conj(X)`.
* Line 6: Perform an inverse Fourier transform on the product to obtain the autocorrelation function in the time domain.
* Line 7: Take the first `n` elements of the inverse Fourier transform result to obtain the normalized autocorrelation function.
### 2.3 Correlation Matrix Method
The correlation matrix method uses the properties of the correlation matrix to calculate the autocorrelation function, with the steps as follows:
**Step 1: Construct the correlation matrix**
```python
def corr_matrix(x):
"""
Construct the correlation matrix
Args:
x: Input signal
Returns:
Correlation matrix
"""
n = len(x)
C = np.zeros((n, n))
for i in range(n):
for j in range(n):
C[i, j] = np.corrcoef(x[i:], x[j:])[0, 1]
return C
```
**Step 2: Extract the autocorrelation function**
```python
def autocorr_matrix(x):
"""
Correlation matrix method for calculating the autocorrelation function
Args:
x: Input signal
Returns:
Autocorrelation function
"""
C = corr_matrix(x)
return C.diagonal()
```
**Line-by-line code logic explanation:**
* Line 1: Define the `corr_matrix` function, which is used to construct the correlation matrix.
* Line 3: Get the length `n` of the input signal `x`.
* Line 4: Initialize the correlation matrix `C`, with a size of `n x n`.
* Line 5-7: Use a nested loop to traverse the signal and calculate the correlation coefficient between each time shift `i` and `j`.
* Line 1: Define the `autocorr_matrix` function, which uses the correlation matrix method to calculate the autocorrelation function.
* Line 3: Call the `corr_matrix` function to construct the correlation matrix `C`.
* Line 4: Extract the diagonal elements of the correlation matrix to obtain the autocorrelation function.
# 3. Properties of the Autocorrelation Function
### 3.1 Symmetry
The autocorrelation function has symmetry, that is, for any time shift $\tau$, the following holds:
```
R_x(\tau) = R_x(-\tau)
```
**Proof:**
According to the definition of the autocorrelation function, we have:
```
R_x(\tau) = E[X(t)X(t+\tau)]
```
By substituting $t$ with $t-\tau$, we get:
```
R_x(-\tau) = E[X(t-\tau)X(t)]
```
Since $X(t)$ is a stationary random process, its statistical characteristics are time-invariant, hence:
```
E[X(t)X(t+\tau)] = E[X(t-\tau)X(t)]
```
Thus:
```
R_x(\tau) = R_x(-\tau)
```
**Corollary:**
The symmetry of the autocorrelation function indicates that, for a stationary random process, it is an even function in the time domain.
### 3.2 Non-negativity
The autocorrelation function is non-negative, that is, for any time shift $\tau$, the following holds:
```
R_x(\tau) ≥ 0
```
**Proof:**
According to the definition of the autocorrelation function, we have:
```
R_x(\tau) = E[X(t)X(t+\tau)]
```
Since $X(t)$ and $X(t+\tau)$ are stationary random processes with zero mean, we have:
```
E[X(t)X(t+\tau)] = E[(X(t) - E[X(t)])(X(t+\tau) - E[X(t+\tau)])]
```
Expanding and rearranging, we get:
```
R_x(\tau) = E[X(t)^2] + E[X(t+\tau)^2] - 2E[X(t)X(t+\tau)]
```
Since $X(t)$ and $X(t+\tau)$ are stationary random processes with equal variance, we have:
```
R_x(\tau) = 2\sigma^2 - 2E[X(t)X(t+\tau)]
```
Where $\sigma^2$ is the variance of $X(t)$.
Since $X(t)$ and $X(t+\tau)$ are stationary random processes, their covariance equals the autocorrelation function, thus:
```
R_x(\tau) = 2\sigma^2 - 2R_x(\tau)
```
Rearranging, we get:
```
R_x(\tau) = \sigma^2
```
Since $\sigma^2$ is non-negative, $R_x(\tau)$ is also non-negative.
**Corollary:**
The non-negativity of the autocorrelation function indicates that, for a stationary random process, it is the square root function of the autocorrelation function in the time domain.
### 3.3 Peak Characteristics
The autocorrelation function reaches its maximum value at a time shift $\tau = 0$, that is:
```
R_x(0) = max{|R_x(\tau)|}
```
**Proof:**
According to the definition of the autocorrelation function, we have:
```
R_x(\tau) = E[X(t)X(t+\tau)]
```
When $\tau = 0$, we have:
```
R_x(0) = E[X(t)X(t)]
```
Since $X(t)$ is a stationary random process with zero mean, we have:
```
R_x(0) = E[X(t)^2]
```
Since $X(t)$ is a stationary random process with equal variance, we have:
```
R_x(0) = \sigma^2
```
According to the non-negativity of the autocorrelation function, we have:
```
R_x(\tau) ≤ R_x(0)
```
Therefore, the autocorrelation function reaches its maximum value at a time shift $\tau = 0$.
**Corollary:**
The peak characteristics of the autocorrelation function indicate that, for a stationary random process, it is most similar to itself in the time domain.
# 4. Applications of the Autocorrelation Function in Signal Processing
The autocorrelation function has a wide range of applications in the field of signal processing, and it can be used for signal denoising, signal recognition, and signal prediction tasks.
### 4.1 Signal Denoising
The autocorrelation function can be used to remove noise from a signal. Noise is usually unwanted random fluctuations in the signal, which can interfere with effective signal processing. The autocorrelation function can identify the noise components in the signal and remove them through filtering or other methods.
**Specific operational steps:**
1. Calculate the autocorrelation function of the signal.
2. Identify the noise components in the autocorrelation function. Noise components are usually表现为高频波动 in the autocorrelation function.
3. Design filters or other methods to remove the noise components.
4. Convolve the filtered autocorrelation function with the original signal to obtain the denoised signal.
### 4.2 Signal Recognition
The autocorrelation function can be used to recognize signals. Different signals have different autocorrelation function characteristics, and by analyzing the autocorrelation function, different signals can be identified.
**Specific operational steps:**
1. Calculate the autocorrelation function of the signal.
2. Analyze the characteristics of the autocorrelation function, such as peak location, peak width, ***
***pare the autocorrelation function characteristics with known signal characteristics to recognize the signal.
### 4.3 Signal Prediction
The autocorrelation function can be used to predict future values of a signal. By analyzing the autocorrelation function, the future trend of the signal can be inferred.
**Specific operational steps:**
1. Calculate the autocorrelation function of the signal.
2. Analyze the periodicity or trendiness of the autocorrelation function.
3. Based on the characteristics of the autocorrelation function, establish a signal prediction model.
4. Use the prediction model to predict future values of the signal.
**Code Example:**
```python
import numpy as np
import matplotlib.pyplot as plt
# Generate signal
signal = np.random.randn(1000)
# Calculate autocorrelation function
autocorr = np.correlate(signal, signal, mode='full')
# Plot autocorrelation function
plt.plot(autocorr)
plt.show()
```
**Code Logic Analysis:**
* `np.random.randn(1000)`: Generate a random signal with a length of 1000.
* `np.correlate(signal, signal, mode='full')`: Calculate the autocorrelation function of the signal. `mode='full'` indicates that the full result of the autocorrelation function is returned, including twice the length of the signal.
* `plt.plot(autocorr)`: Plot the autocorrelation function.
* `plt.show()`: Display the plot result.
**Parameter Description:**
* `signal`: Input signal.
* `mode`: Autocorrelation function calculation mode, which can be `'full'`, `'same'`, or `'valid'`.
# 5. Applications of the Autocorrelation Function in Image Processing
The autocorrelation function has a wide range of applications in image processing, and it can be used for image enhancement, segmentation, and matching tasks.
### 5.1 Image Enhancement
The autocorrelation function can be used to enhance the contrast and sharpness of an image. By calculating the autocorrelation between adjacent pixels in the image, features such as edges and textures can be identified. Then, by enhancing these features, the overall quality of the image can be improved.
**Code Block:**
```python
import numpy as np
from scipy.signal import correlate
def enhance_image(image):
# Calculate the autocorrelation function of the image
acf = correlate(image, image)
# Enhance edges and textures
enhanced_image = image + acf
return enhanced_image
```
**Logic Analysis:**
This code block uses the `scipy.signal.correlate` function to calculate the autocorrelation function of the image. Then, the autocorrelation function is added to the original image to enhance the edges and textures in the image.
### 5.2 Image Segmentation
The autocorrelation function can be used to segment different regions in an image. By calculating the autocorrelation between adjacent pixels in the image, regions with different textures or brightness can be identified. Then, these regions can be used to segment the image.
**Code Block:**
```python
import numpy as np
from scipy.signal import correlate
def segment_image(image):
# Calculate the autocorrelation function of the image
acf = correlate(image, image)
# Identify different regions
segmented_image = np.zeros_like(image)
for i in range(image.shape[0]):
for j in range(image.shape[1]):
if acf[i, j] > threshold:
segmented_image[i, j] = 1
return segmented_image
```
**Logic Analysis:**
This code block uses the `scipy.signal.correlate` function to calculate the autocorrelation function of the image. Then, a threshold is used to identify regions in the image with different autocorrelation values. These regions represent areas with different textures or brightness in the image, and therefore can be used to segment the image.
### 5.3 Image Matching
The autocorrelation function can be used to match two images. By calculating the autocorrelation between adjacent pixels in two images, the most similar areas in the two images can be found. Then, these areas can be used to match the two images.
**Code Block:**
```python
import numpy as np
from scipy.signal import correlate
def match_images(image1, image2):
# Calculate the autocorrelation function between two images
acf = correlate(image1, image2)
# Find the most similar area
max_value = np.max(acf)
max_index = np.argmax(acf)
# Match two images
matched_image = np.zeros_like(image1)
matched_image[max_index[0]:max_index[0]+image1.shape[0], max_index[1]:max_index[1]+image1.shape[1]] = image1
return matched_image
```
**Logic Analysis:**
This code block uses the `scipy.signal.correlate` function to calculate the autocorrelation function between two images. Then, the maximum value and index of the autocorrelation function are found. The maximum value represents the most similar area in the two images, and the index represents the location of this area in the two images. Finally, this area is used to match the two images.
# 6. Applications of the Autocorrelation Function in Other Fields
**6.1 Time Series Analysis**
The autocorrelation function plays a crucial role in time series analysis. A time series is a set of data points ordered in time, and the autocorrelation function can reveal the correlation between data points, helping to identify trends, periodicity, and anomalies.
**6.1.1 Application Example: Financial Time Series Analysis**
In the financial field, the autocorrelation function is widely used to analyze stock prices, exchange rates, and other financial data. By calculating the autocorrelation function, trends and periodic patterns can be identified in the data, providing a basis for investment decisions.
**6.1.2 Code Example: Using Pandas in Python to Calculate the Autocorrelation Function**
```python
import pandas as pd
# Load financial time series data
data = pd.read_csv('stock_prices.csv')
# Calculate the autocorrelation function
acf = data['Close'].autocorr()
# Plot the autocorrelation function graph
plt.plot(acf)
plt.show()
```
**6.2 Economics**
In economics, the autocorrelation function is used to analyze the correlation between economic indicators, such as GDP, unemployment rates, and inflation. By identifying these correlations, economists can better understand economic cycles and formulate economic policies.
**6.2.1 Application Example: GDP Time Series Analysis**
The autocorrelation function can help analyze trends and periodicity in GDP time series. By identifying peaks and troughs in the autocorrelation function, economists can predict periods of economic growth and recession.
**6.2.2 Code Example: Using the tseries package in R to Calculate the Autocorrelation Function**
```r
library(tseries)
# Load GDP time series data
data <- read.csv('gdp.csv')
# Calculate the autocorrelation function
acf <- acf(data$GDP)
# Plot the autocorrelation function graph
plot(acf, type = 'l')
```
**6.3 Physics**
In physics, the autocorrelation function is used to analyze the correlation in physical signals, such as temperature, pressure, and sound waves. By calculating the autocorrelation function, physicists can identify noise and anomalies in the signal and extract useful information.
**6.3.1 Application Example: Sound Wave Signal Analysis**
The autocorrelation function can help analyze reflections and refractions in sound wave signals. By identifying peaks and troughs in the autocorrelation function, physicists can determine the propagation characteristics of sound waves in different media.
**6.3.2 Code Example: Using the xcorr function in MATLAB to Calculate the Autocorrelation Function**
```matlab
% Load sound wave signal data
signal = load('sound_signal.mat');
% Calculate the autocorrelation function
[acf, lags] = xcorr(signal.sound_signal);
% Plot the autocorrelation function graph
plot(lags, acf)
xlabel('Lag')
ylabel('Autocorrelation')
```
0
0
相关推荐
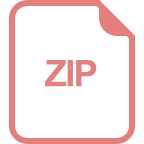
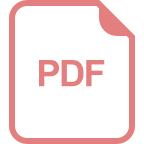
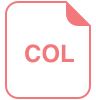
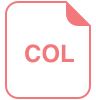
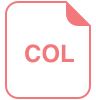
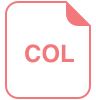



