Common Issues and Solutions for Matlab Autocorrelation Function: Parameter Selection and Results Interpretation
发布时间: 2024-09-15 18:06:47 阅读量: 34 订阅数: 31 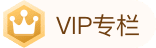
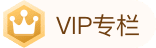
# Common Issues and Solutions for Autocorrelation Function in Matlab: Parameter Selection and Result Interpretation
## 1. Basic Concepts of Autocorrelation Function
### 1.1 Definition of Autocorrelation Function
The autocorrelation function (ACF) measures the correlation of a time series with itself at various lag times. For a time series {X_t}, its autocorrelation function is defined as:
```
ACF(k) = Corr(X_t, X_{t+k})
```
where k is the lag time and Corr() is the correlation coefficient function.
### 1.2 Properties of Autocorrelation Function
The autocorrelation function has the following properties:
* Symmetry: ACF(k) = ACF(-k)
* Normalization: ACF(0) = 1
* Non-negativity: ACF(k) ≥ 0
## 2. Parameter Selection for Autocorrelation Function
The choice of parameters for the autocorrelation function is crucial for the accuracy of the results. This chapter will discuss two key parameters: lag order and window function.
### 2.1 Selection of Lag Order
Lag order represents the lag steps considered in the autocorrelation function. Choosing the appropriate lag order is essential for capturing the correlation of the data.
#### 2.1.1 Empirical Rule
The empirical rule is a simple method to determine the lag order. It suggests setting the lag order to 10% to 20% of the data length. For example, for a sequence with 100 data points, the lag order can be set to 10 to 20.
#### ***
***mon information criteria include the Akaike Information Criterion (AIC) and the Bayesian Information Criterion (BIC). These criteria consider the goodness of fit of the autocorrelation function and the complexity of the model.
### 2.2 Selection of Window Function
The window function is used to weight the data to reduce edge effects. Choosing the appropriate window function can improve the accuracy of the autocorrelation function.
#### 2.2.1 Rectangular Window
The rectangular window is the simplest window function, which assigns the same weight to all data points. It is suitable for stationary time series, but for non-stationary sequences, it may cause edge effects.
#### 2.2.2 Hamming Window
The Hamming window is a smooth window function that assigns smaller weights at the beginning and end of the data sequence. It can reduce the edge effects of the rectangular window and is suitable for most time series.
#### 2.2.3 Hanning Window
The Hanning window is a smoother window function that assigns even smaller weights at the start and end of the data sequence. It has stronger edge effect suppression than the Hamming window and is suitable for non-stationary time series.
**Code Block:**
```
% Load data
data = load('data.mat');
% Choose lag order
lag = 10;
% Choose window function
window = 'hamming';
% Calculate autocorrelation function
acf = autocorr(data, lag, window);
% Plot autocorrelation function
figure;
plot(acf);
xlabel('Lag');
ylabel('Autocorrelation');
title('Autocorrelation Function');
```
**Logical Analysis:**
This code demonstrates the parameter selection for the autocorrelation function. It loads data, specifies the lag order and window function, then calculates and plots the autocorrelation function.
**Parameter Explanation:**
* `data`: The data series to calculate the autocorrelation function.
* `lag`: Lag order.
* `window`: Type of window function, which can be 'rectangular', 'hamming', or 'hanning'.
## 3. Interpretation of Autocorrelation Function Results
### 3.1 Meaning of Autocorrelation Coefficients
The range of values for the autocorrelation function is [-1, 1]. The value of the autocorrelation coefficient indicates the correlation of the time s
0
0
相关推荐








