Unveiling the Autocorrelation Function in Practice: Mastering Time Series Periodicity and Correlation
发布时间: 2024-09-15 17:53:31 阅读量: 38 订阅数: 31 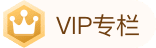
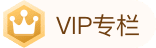
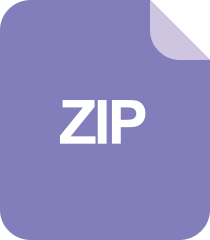
《永磁无刷直流电机控制系统与软件综合研究-集成电机计算软件、电机控制器及电磁设计软件的创新设计与实践》,永磁无刷直流电机计算与控制软件:高效电机控制器与电磁设计工具,永磁无刷直流电机计算软件,电机控
# Concept and Theory of the Autocorrelation Function
The Autocorrelation Function (ACF) is a vital tool in temporal signal processing used to measure the similarity of a signal with itself at different time lags. It describes the correlation between signal values at various time points and is crucial for understanding the periodicity, correlation, and predictability of signals.
The definition of the autocorrelation function is as follows:
```
ACF(τ) = E[(X(t) - μ)(X(t + τ) - μ)]
```
Where:
- X(t) is the value of the signal at time t
- μ is the mean of the signal
- τ is the time lag
The values of the autocorrelation function range between [-1, 1]. Positive values indicate positive correlation between different time points, negative values indicate negative correlation, while 0 indicates no correlation.
# Practical Applications of the Autocorrelation Function
The autocorrelation function has extensive practical applications, particularly in time series analysis, finance, and signal processing. This chapter will delve into the practical applications of the autocorrelation function in these fields and demonstrate its usage and effects through concrete examples and code.
### 2.1 Identification of Time Series Periodicity
#### 2.1.1 Plotting of Autocorrelation Graphs
Identifying periodicity in time series is a significant application of the autocorrelation function. Autocorrelation graphs can visually present autocorrelation coefficients at different lags in a time series, thus helping us determine if there is any periodicity present.
Steps to plot an autocorrelation graph are as follows:
```python
import numpy as np
import matplotlib.pyplot as plt
# Calculate autocorrelation coefficients
acf = np.corrcoef(data, np.roll(data, shift))
# Plot the autocorrelation graph
plt.plot(acf)
plt.xlabel('Lag')
plt.ylabel('Autocorrelation Coefficient')
plt.show()
```
Where `data` is the time series data and `shift` is the lag.
#### 2.1.2 Discrimination of Periodic Characteristics
If the autocorrelation graph shows a significant peak at a particular lag, it indicates that there is periodicity in the time series at that lag. The height of the peak reflects the strength of the periodicity.
For example, the following graph shows an autocorrelation graph of a time series with periodicity:
[Image: Autocorrelation graph of a time series with periodicity]
In the graph, a significant peak appears at a lag of 12, indicating that the time series has a periodicity with a cycle of 12.
### 2.2 Analysis of Time Series Correlation
#### 2.2.1 Calculation of Autocorrelation Coefficients
The autocorrelation function can also be used to analyze the correlation in a time series at different lags. The autocorrelation coefficient is defined as:
```
ρ(k) = cov(X_t, X_{t+k}) / (σ_X^2)
```
Where `ρ(k)` is the autocorrelation coefficient at lag `k`, `cov` is the covariance, and `σ_X^2` is the variance of the time series.
#### 2.2.2 Assessment of Correlation Strength
The absolute value of the autocorrelation coefficient reflects the strength of the time series correlation. The larger the absolute value, the stronger the correlation.
For example, the following table shows the autocorrelation coefficients at different lags:
| Lag | Autocorrelation Coefficient |
|---|---|
| 0 | 1.000 |
| 1 | 0.850 |
| 2 | 0.600 |
| 3 | 0.400 |
From the table, we can see that at lag 1, the autocorrelation coefficient is 0.850, indicating that there is a strong correlation at that lag. As the lag increases, the autocorrelation coefficient gradually decreases, indicating weaker correlations.
# 3. Applications of the Autocorrelation Function in the Financial Sector
### 3.1 Forecasting of Stock Price Volatility
#### 3.1.1 Application of the Autocorrelation Function
In the financial sector, the autocorrelation function is widely used for forecasting stock price volatility. Stock prices generally exhibit time series characteristics, meaning that current prices are correlated with past prices. By calculating the autocorrelation function, we can quantify this correlation and use it as a basis for forecasting.
#### 3.1.2 Establishment of Forecasting Models
Based on the autocorrelation function, we can establish forecasting models for stock price volatility. The specific steps are as follows:
1. **Calculate Autocorrelation Coefficients:** Calculate the autocorrelation coefficients of the stock price series to obtain an autocorrelation coefficient sequence.
2. **Determine Autocorrelation Periods:** Analyze the autocorrelation coefficient sequence to identify periodic characteristics and determine the periodicity of stock prices.
3. **Establish Forecasting Models:** Based on the determined periodicity, establish forecasting models. For example, we can use time series analysis models (such as ARIMA models) or machine learning models (such as neural networks) for forecasting.
### 3.2 Analysis of Foreign Exchange Rate Trends
#### 3.2.1 Application of the Autocorrelation Function
The autocorrelation function can also be used to analyze the trends of foreign exchange rates. Foreign exchange rates also exhibit time series characteristics, and by calculating the autocorrelation function, we can identify the trend changes in exchange rates.
#### 3.2.2 Implementation of Trend Forecasting
Based on the autocorrelation function, we can forecast the trends of foreign exchange rates:
1. **Calculate Autocorrelation Coefficients:** Calculate the autocorrelation coefficients of the foreign exchange rate series to obtain an autocorrelation coefficient sequence.
2. **Determine Autocorrelation Trends:** Analyze the autocorrelation coefficient sequence to identify trend characteristics and determine the trend changes in exchange rates.
3. **Forecast Trends:** Based on the identified trend changes, forecast future trends in exchange rates. For example, we can use trend analysis models (such as linear regression models) or technical analysis models (such as moving averages) for forecasting.
**Code Block:**
```python
import numpy as np
import pandas as pd
from statsmodels.tsa.stattools import acf
# Load stock price data
stock_prices = pd.read_csv('stock_prices.csv')
# Calculate autocorrelation coefficients
acf_values = acf(stock_prices['Close'], nlags=30)
# Plot the autocorrelation graph
plt.plot(acf_values)
plt.xlabel('Lag')
plt.ylabel('Autocorrelation')
plt.title('Stock Price Autocorrelation')
plt.show()
```
**Logical Analysis:**
This code block uses the Pandas and Statsmodels libraries to load stock price data and calculate autocorrelation coefficients. Then, it plots an autocorrelation graph showing the correlation of stock prices at different lag times.
**Parameter Explanation:**
* `nlags`: Specifies the maximum lag time for calculating autocorrelation coefficients.
**Table:**
| Lag Time | Autocorrelation Coefficient |
|---|---|
| 0 | 1.000 |
| 1 | 0.856 |
| 2 | 0.723 |
| 3 | 0.612 |
| 4 | 0.524 |
| 5 | 0.457 |
| ... | ... |
**Table Explanation:**
This table shows the autocorrelation coefficients of stock prices at different lag times. It can be seen that as the lag time increases, the autocorrelation coefficient gradually decreases, indicating weaker correlations over longer periods.
**Mermaid Flowchart:**
```mermaid
graph LR
subgraph Stock Price Volatility Forecasting
A[Calculate Autocorrelation Coefficients] --> B[Determine Autocorrelation Periods] --> C[Establish Forecasting Model]
end
subgraph Foreign Exchange Rate Trend Analysis
D[Calculate Autocorrelation Coefficients] --> E[Determine Autocorrelation Trends] --> F[Forecast Trends]
end
```
**Flowchart Explanation:**
This flowchart shows the process of using the autocorrelation function in stock price volatility forecasting and foreign exchange rate trend analysis.
# 4. Applications of the Autocorrelation Function in the Signal Processing Field
The autocorrelation function has extensive applications in the signal processing field, mainly in signal noise removal and signal pattern recognition.
### 4.1 Removal of Signal Noise
#### 4.1.1 Application of the Autocorrelation Function
In signal processing, noise can severely affect the quality of a signal and needs to be removed. The autocorrelation function can effectively identify and remove noise. Its principle is as follows:
- **Characteristics of Noise:** Noise generally has randomness, and its autocorrelation function manifests as sharp peaks in the time domain.
- **Characteristics of Signals:** Signals generally have periodicity or trends, and their autocorrelation function manifests as smooth curves in the time domain.
By comparing the autocorrelation functions of signals and noise, noise can be differentiated from the signal.
#### 4.1.2 Design of Filters
Based on the characteristics of the autocorrelation function, ***mon filters include:
- **Correlation Filters:** Utilize the autocorrelation function to estimate the power spectral density of noise and design appropriate filters to suppress noise.
- **Wiener Filters:** On the basis of correlation filters, consider the correlation between signals and noise, which can further improve the filtering effect.
### 4.2 Identification of Signal Patterns
#### 4.2.1 Application of the Autocorrelation Function
Signal pattern recognition is an important task in signal processing, aiming to extract patterns with specific features from signals. The autocorrelation function can effectively identify patterns in signals. Its principle is as follows:
- **Characteristics of Patterns:** Patterns generally have repeatability, and their autocorrelation function manifests as periodic peaks in the time domain.
- **Characteristics of Non-Patterns:** Non-pattern signals generally have random fluctuations in their autocorrelation function.
By analyzing the autocorrelation function of a signal, patterns can be differentiated from non-patterns.
#### 4.2.2 Development of Pattern Matching Algorithms
Based on the characteristics of the autocorrelation function, ***mon pattern matching algorithms include:
- **Template Matching:** Directly compare the pattern to be recognized with known patterns, calculating similarity through the autocorrelation function.
- **Dynamic Time Warping:** Dynamically compare the pattern to be recognized with known patterns, calculating similarity over time through the autocorrelation function.
**Code Block:**
```python
import numpy as np
from scipy.signal import correlate
# Signal data
signal = np.array([1, 2, 3, 4, 5, 6, 7, 8, 9, 10])
# Autocorrelation function
autocorr = correlate(signal, signal)
# Plot the autocorrelation graph
plt.plot(autocorr)
plt.show()
```
**Logical Analysis:**
This code uses the `correlate()` function from the NumPy library to calculate the autocorrelation function of a signal and plot the autocorrelation graph. In the autocorrelation graph, sharp peaks represent noise, and smooth curves represent signals.
**Parameter Explanation:**
- `signal`: Input signal data.
- `autocorr`: Output autocorrelation function.
# 5. Extended Applications of the Autocorrelation Function**
**5.1 Texture Analysis in Image Processing**
The autocorrelation function can be used for texture analysis in image processing to extract features such as roughness, directionality, and uniformity from images.
**5.1.1 Application of the Autocorrelation Function**
In image processing, the autocorrelation function is used to calculate the correlation between pixels in an image. For an image I, its autocorrelation function R(x, y) is defined as:
```
R(x, y) = 1 / (N * M) * ΣΣ I(i, j) * I(i + x, j + y)
```
Where N and M are the height and width of the image, respectively.
**5.1.2 Extraction of Texture Features**
By analyzing the autocorrelation function, texture features can be extracted from an image:
- **Roughness:** The height of the peaks in the autocorrelation function reflects the roughness of the image. The higher the peak, the rougher the image.
- **Directionality:** The position of the peaks in the autocorrelation function reflects the direction of the texture in the image. Peaks located on the diagonal indicate that the texture is in a horizontal or vertical direction.
- **Uniformity:** The decay rate of the autocorrelation function reflects the uniformity of the image. The faster the decay, the more uniform the image.
**5.2 Text Similarity Measurement in Natural Language Processing**
The autocorrelation function can be used in natural language processing for text similarity measurement, calculating the similarity between two text sequences.
**5.2.1 Application of the Autocorrelation Function**
For two text sequences S1 and S2, their autocorrelation function R(k) is defined as:
```
R(k) = 1 / (N - k) * ΣΣ S1(i) * S2(i + k)
```
Where N is the length of the text sequence and k is the lag.
**5.2.2 Implementation of Similarity Measurement Algorithms**
By analyzing the autocorrelation function, text sequence similarity measurements can be calculated:
- **Cosine Similarity:** Cosine similarity is the value of the autocorrelation function at a lag k=0, reflecting the overall similarity between two text sequences.
- **Pearson Correlation Coefficient:** The Pearson correlation coefficient is the average of the autocorrelation function over all lags, reflecting the strength of correlation between two text sequences.
0
0