Autocorrelation Function and Cross-correlation Function: Revealing the Correlations in Different Time Series
发布时间: 2024-09-15 18:04:39 阅读量: 16 订阅数: 46 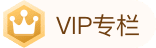
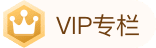
# Autocorrelation Function and Cross-correlation Function: Unveiling the Association Between Different Time Series
## 1. Concepts and Theoretical Foundations of Autocorrelation and Cross-correlation Functions
### 1.1 Autocorrelation Function
The Autocorrelation Function (ACF) measures the correlation between observations at specific time intervals within a time series. It is defined as:
```
ACF(k) = Cov(X_t, X_{t+k}) / Var(X_t)
```
where:
- `X_t` is the observation of the time series at time `t`
- `k` is the time interval
- `Cov()` is the covariance
- `Var()` is the variance
The range of values for the ACF is [-1, 1]. Positive values indicate positive correlation, negative values indicate negative correlation, and 0 indicates no correlation.
## 2. Calculation Methods for Autocorrelation and Cross-correlation Functions
### 2.1 Calculation of Autocorrelation Function
**Definition:**
The Autocorrelation Function (ACF) measures the correlation between an observation and itself at different time lags within a time series. It is defined as:
```
ρ(k) = Cov(X_t, X_{t+k}) / Var(X_t)
```
where:
- ρ(k) is the autocorrelation coefficient at lag k
- X_t is the observation of the time series at time t
- Cov(X_t, X_{t+k}) is the covariance between X_t and X_{t+k}
- Var(X_t) is the variance of X_t
**Calculation Method:**
The autocorrelation function can be calculated using the following steps:
1. Calculate the mean of the time series: μ = (1/N) ΣX_t
2. Calculate the variance of the time series: σ^2 = (1/N) Σ(X_t - μ)^2
3. Calculate the covariance at different lags k: Cov(X_t, X_{t+k}) = (1/N) Σ(X_t - μ)(X_{t+k} - μ)
4. Calculate the autocorrelation coefficient: ρ(k) = Cov(X_t, X_{t+k}) / σ^2
**Code Block:**
```python
import numpy as np
def autocorr(x, k):
"""Calculate the autocorrelation function.
Parameters:
x: Time series
k: Lag
Returns:
The autocorrelation coefficient at lag k
"""
mean = np.mean(x)
var = np.var(x)
cov = np.cov(x, np.roll(x, k))[0, 1]
return cov / var
```
**Logical Analysis:**
This code block implements the calculation of the autocorrelation function. It first calculates the mean and variance of the time series. Then, it uses the `np.cov()` function to calculate the covariance of the time series with itself at lag k. Finally, it divides the covariance by the variance to obtain the autocorrelation coefficient.
### 2.2 Calculation of Cross-correlation Function
**Definition:**
The Cross-correlation Function (CCF) measures the correlation between observations in two time series at different time lags. It is defined as:
```
ρ_{XY}(k) = Cov(X_t, Y_{t+k}) / (σ_X σ_Y)
```
where:
- ρ_{XY}(k) is the cross-correlation coefficient at lag k
- X_t is the observation of time series X at time t
- Y_{t+k} is the observation of time series Y at time t+k
- Cov(X_t, Y_{t+k}) is the covariance between X_t and Y_{t+k}
- σ_X is the standard deviation of time series X
- σ_Y is the standard deviation of time series Y
**Calculation Method:**
The cross-correlation function can be calculated using the following steps:
1. Calculate the means of the two time series: μ_X = (1/N) ΣX_t, μ_Y = (1/N) ΣY_t
2. Calculate the standard deviations of the two time series: σ_X = (1/N) Σ(X_t - μ_X)^2, σ_Y = (1/N) Σ(Y_t - μ_Y)^2
3. Calculate the covariance at different lags k: Cov(X_t, Y_{t+k}) = (1/N) Σ(X_t - μ_X)(Y_{t+k} - μ_Y)
4. Calculate the cross-correlation coefficient: ρ_{XY}(k) = Cov(X_t, Y_{t+k}) / (σ_X σ_Y)
**Code Block:**
```python
import numpy as np
def crosscorr(x, y, k):
"""Calculate the cross-correlation function.
Parameters:
x: Time series X
y: Time series Y
k: Lag
Returns:
The cross-correlation coefficient at lag k
"""
mean_x = np.mean(x)
mean_y = np.mean(y)
std_x = np.std(x)
std_y = np.std(y)
cov = np.cov(x, np.roll(y, k))[0, 1]
return cov / (std_x * std_y)
```
**Logical Analysis:**
This code block implements the calculation of the cross-correlation function. I
0
0
相关推荐
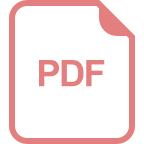
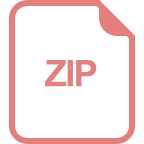






