int sum=0; for (int i=0;i<101;i++) { sum = sum + i; } return View();
时间: 2024-02-14 18:24:53 浏览: 29
根据您提供的代码,这是一个C#语言中的for循环求和示例。在每次迭代中,变量`i`从0递增到100,将当前的`i`值加到`sum`变量中。最后,将求和结果存储在变量`sum`中。
然而,代码中的`return View();`语句看起来是ASP.NET MVC框架中的视图返回语句。如果您正在开发一个MVC应用程序,并希望在视图中显示求和结果,您可以将求和结果传递给视图并在视图中进行显示。
以下是一个简单的示例,展示如何在ASP.NET MVC中将求和结果传递给视图:
在控制器中:
```csharp
public ActionResult Index()
{
int sum = 0;
for (int i = 0; i <= 100; i++)
{
sum += i;
}
ViewBag.Sum = sum;
return View();
}
```
在视图中(例如Index.cshtml):
```html
<h1>Sum: @ViewBag.Sum</h1>
```
这样,在视图中就可以显示求和结果。
相关问题
void C312105010501View::OnJunzhi(){ C312105010501Doc* pDoc = GetDocument(); ASSERT_VALID(pDoc); if (pDoc->m_pDib == NULL) { AfxMessageBox("未打开图像"); return; } int mcols = pDoc->m_pDib->m_nCols; int mrows = pDoc->m_pDib->m_nRows; unsigned char* m_pData = NULL; m_pData = pDoc->m_pDib->pImgData; unsigned char* m_pDataOldPos = m_pData; BYTE* ptmp = new BYTE[mcols * mrows]; memcpy(ptmp, m_pData, mcols * mrows); int Template[9] = { 1,1,1,1,1,1,1,1,1 }; int mask = 3; int irows, jcols; for (jcols = mask / 2; jcols < mcols - mask / 2; jcols++) { for (irows = mask / 2; irows < mrows - mask / 2; irows++) { int sum = 0, m, n; for (m = -mask / 2; m <= mask / 2; m++) { for (n = -mask / 2; n <= mask / 2; n++) { sum += ptmp[(irows + m) * mcols + jcols + n] * Template[(m + mask / 2) * mask + n + mask / 2]; } } sum = sum / 9; if (sum > 255) sum = 255; m_pData[irows * mcols + jcols] = sum; m_pData = m_pDataOldPos; } m_pData = m_pDataOldPos; } pDoc->UpdateAllViews(NULL);}仿照以上代码写一个3*5均值滤波处理和5*5均值滤波处理和7-7均值滤波处理
以下是分别实现3*5、5*5和7*7均值滤波的代码:
3*5均值滤波:
```c++
void CMyView::OnJunzhi35()
{
CMyDoc* pDoc = GetDocument();
ASSERT_VALID(pDoc);
if (pDoc->m_pDib == NULL)
{
AfxMessageBox("未打开图像");
return;
}
int mcols = pDoc->m_pDib->m_nCols;
int mrows = pDoc->m_pDib->m_nRows;
unsigned char* m_pData = pDoc->m_pDib->pImgData;
unsigned char* m_pDataOldPos = m_pData;
// 备份原始数据
BYTE* ptmp = new BYTE[mcols * mrows];
memcpy(ptmp, m_pData, mcols * mrows);
// 定义模板和掩码大小
int Template[15] = { 1, 1, 1, 1, 1,
1, 1, 1, 1, 1,
1, 1, 1, 1, 1 };
int maskCol = 2;
int maskRow = 1;
// 对每个像素进行处理
for (int j = maskCol; j < mcols - maskCol; j++)
{
for (int i = maskRow; i < mrows - maskRow; i++)
{
int sum = 0;
for (int m = -maskRow; m <= maskRow; m++)
{
for (int n = -maskCol; n <= maskCol; n++)
{
sum += ptmp[(i + m) * mcols + j + n] * Template[(m + maskRow) * (2 * maskCol + 1) + n + maskCol];
}
}
sum /= 15;
if (sum > 255)
{
sum = 255;
}
m_pData[i * mcols + j] = sum;
}
}
// 释放内存
delete[] ptmp;
pDoc->UpdateAllViews(NULL);
}
```
5*5均值滤波:
```c++
void CMyView::OnJunzhi55()
{
CMyDoc* pDoc = GetDocument();
ASSERT_VALID(pDoc);
if (pDoc->m_pDib == NULL)
{
AfxMessageBox("未打开图像");
return;
}
int mcols = pDoc->m_pDib->m_nCols;
int mrows = pDoc->m_pDib->m_nRows;
unsigned char* m_pData = pDoc->m_pDib->pImgData;
unsigned char* m_pDataOldPos = m_pData;
// 备份原始数据
BYTE* ptmp = new BYTE[mcols * mrows];
memcpy(ptmp, m_pData, mcols * mrows);
// 定义模板和掩码大小
int Template[25] = { 1, 1, 1, 1, 1,
1, 1, 1, 1, 1,
1, 1, 1, 1, 1,
1, 1, 1, 1, 1,
1, 1, 1, 1, 1 };
int maskCol = 2;
int maskRow = 2;
// 对每个像素进行处理
for (int j = maskCol; j < mcols - maskCol; j++)
{
for (int i = maskRow; i < mrows - maskRow; i++)
{
int sum = 0;
for (int m = -maskRow; m <= maskRow; m++)
{
for (int n = -maskCol; n <= maskCol; n++)
{
sum += ptmp[(i + m) * mcols + j + n] * Template[(m + maskRow) * (2 * maskCol + 1) + n + maskCol];
}
}
sum /= 25;
if (sum > 255)
{
sum = 255;
}
m_pData[i * mcols + j] = sum;
}
}
// 释放内存
delete[] ptmp;
pDoc->UpdateAllViews(NULL);
}
```
7*7均值滤波:
```c++
void CMyView::OnJunzhi77()
{
CMyDoc* pDoc = GetDocument();
ASSERT_VALID(pDoc);
if (pDoc->m_pDib == NULL)
{
AfxMessageBox("未打开图像");
return;
}
int mcols = pDoc->m_pDib->m_nCols;
int mrows = pDoc->m_pDib->m_nRows;
unsigned char* m_pData = pDoc->m_pDib->pImgData;
unsigned char* m_pDataOldPos = m_pData;
// 备份原始数据
BYTE* ptmp = new BYTE[mcols * mrows];
memcpy(ptmp, m_pData, mcols * mrows);
// 定义模板和掩码大小
int Template[49] = { 1, 1, 1, 1, 1, 1, 1,
1, 1, 1, 1, 1, 1, 1,
1, 1, 1, 1, 1, 1, 1,
1, 1, 1, 1, 1, 1, 1,
1, 1, 1, 1, 1, 1, 1,
1, 1, 1, 1, 1, 1, 1,
1, 1, 1, 1, 1, 1, 1 };
int maskCol = 3;
int maskRow = 3;
// 对每个像素进行处理
for (int j = maskCol; j < mcols - maskCol; j++)
{
for (int i = maskRow; i < mrows - maskRow; i++)
{
int sum = 0;
for (int m = -maskRow; m <= maskRow; m++)
{
for (int n = -maskCol; n <= maskCol; n++)
{
sum += ptmp[(i + m) * mcols + j + n] * Template[(m + maskRow) * (2 * maskCol + 1) + n + maskCol];
}
}
sum /= 49;
if (sum > 255)
{
sum = 255;
}
m_pData[i * mcols + j] = sum;
}
}
// 释放内存
delete[] ptmp;
pDoc->UpdateAllViews(NULL);
}
```
给这段代码添加中文注释:#include<stdio.h> #include<conio.h> #include<windows.h> struct student { char code[10]; float s1,s2,s3; float score; char grade; }temp; void set(int *choice) { printf(" ******* 1、从文件读取数据 *******\n"); printf(" ******* 2、计算并显示成绩 *******\n"); printf(" ******* 3、按等级查询 *******\n"); printf(" ******* 4、按总评成绩排序 *******\n"); printf(" ******* 5、按学号查询 *******\n"); printf(" ******* 6、查询所有等级 *******\n"); printf(" ******* 7、保存到文件 *******\n"); printf(" ******* 0、退出系统 *******\n\n"); printf("请选择您所需要操作的功能:"); *choice=getch(); } void dispinfo() { system("cls"); printf("\n\n"); printf(" *********************** 欢迎成绩核算系统 **********************\n\n"); printf(" *********************** **************** **********************\n\n"); } int duqu(struct student *list) { int n = 0; FILE fp = fopen("C:\Users\dell\Desktop\软件设计-源文件\A3\note.txt", "r"); if (fp == NULL) { printf("无法打开文件"); return n; } char line[100]; fgets(line, 100, fp); sscanf(line, "%d", &n); printf("\n"); // 空一行 printf(" 学号 平时成绩 期中成绩 期末成绩 \n"); // 输出表头 for (int i = 0; i < n; i++) { fgets(line, 100, fp); sscanf(line, "%s %f %f %f", list[i].code, &list[i].s1, &list[i].s2, &list[i].s3); printf("\t %s %5.1lf %5.1lf %5.1lf \n", list[i].code, list[i].s1, list[i].s2, list[i].s3); } printf("\n\n\n ****** 读取文件成功! 按任意键继续! ****** \n"); fclose(fp); while (getchar() != '\n'); // 等待用户按下回车键继续 return n; } void view(struct student list, int amount) { float sum = 0, average = 0; printf("\n 学号 总评 等级 \n"); for (int i = 0; i < amount; i++) { sum += list[i].score; printf("%10s %6.1f %c \n", list[i].code, list[i].score, list[i].grade); } average = sum / amount; printf(" 本班总人数 %d 本班平均成绩 %.1f\n", amount, average); printf("\n\n\n\n\n ***** 摁任意键继续! ****** \n"); getch(); } void paixu(struct student list, int amount) { for (int i = 0; i < amount; i++) { for (int j = i + 1; j < amount; j++) { if (list[j].score > list[i].score) { struct student temp = list[j]; list[j] = list[i]; list[i] = temp; } } } view(list, amount); } void calculate(struct student list[], int amount) { for(int i = 0; i < amount; i++) { float score = list[i].s10.3 + list[i].s20.3 + list[i].s30.4; list[i].score = score; if(score >= 90) { list[i].grade = 'A'; } else if(score >= 80) { list[i].grade = 'B'; } else if(score >= 70) { list[i].grade = 'C'; } else if(score >= 60) { list[i].grade = 'D'; } else { list[i].grade = 'E'; } } dispinfo(); view(list, amount); }
// 引入头文件
#include<stdio.h>
#include<conio.h>
#include<windows.h>
// 定义学生结构体
struct student {
char code[10]; // 学号
float s1,s2,s3; // 平时成绩,期中成绩,期末成绩
float score; // 总评成绩
char grade; // 成绩等级
}temp;
// 设置函数,用于选择功能
void set(int *choice) {
printf(" ******* 1、从文件读取数据 *******\n");
printf(" ******* 2、计算并显示成绩 *******\n");
printf(" ******* 3、按等级查询 *******\n");
printf(" ******* 4、按总评成绩排序 *******\n");
printf(" ******* 5、按学号查询 *******\n");
printf(" ******* 6、查询所有等级 *******\n");
printf(" ******* 7、保存到文件 *******\n");
printf(" ******* 0、退出系统 *******\n\n");
printf("请选择您所需要操作的功能:");
*choice=getch();
}
// 显示信息函数
void dispinfo() {
system("cls"); // 清屏
printf("\n\n");
printf(" *********************** 欢迎成绩核算系统 **********************\n\n");
printf(" *********************** **************** **********************\n\n");
}
// 读取文件函数
int duqu(struct student *list) {
int n = 0;
FILE fp = fopen("C:\Users\dell\Desktop\软件设计-源文件\A3\note.txt", "r"); // 打开文件
if (fp == NULL) { // 判断文件是否能够打开
printf("无法打开文件");
return n;
}
char line[100]; // 定义一个字符数组
fgets(line, 100, fp); // 读取一行
sscanf(line, "%d", &n); // 从字符串中读取一个整数
printf("\n"); // 空一行
printf(" 学号 平时成绩 期中成绩 期末成绩 \n"); // 输出表头
for (int i = 0; i < n; i++) { // 循环读取每个学生的信息
fgets(line, 100, fp); // 读取一行
sscanf(line, "%s %f %f %f", list[i].code, &list[i].s1, &list[i].s2, &list[i].s3); // 从字符串中读取学生的学号和成绩
printf("\t %s %5.1lf %5.1lf %5.1lf \n", list[i].code, list[i].s1, list[i].s2, list[i].s3); // 输出学生的成绩
}
printf("\n\n\n ****** 读取文件成功! 按任意键继续! ****** \n");
fclose(fp); // 关闭文件
while (getchar() != '\n'); // 等待用户按下回车键继续
return n;
}
// 显示学生成绩函数
void view(struct student list, int amount) {
float sum = 0, average = 0;
printf("\n 学号 总评 等级 \n");
for (int i = 0; i < amount; i++) {
sum += list[i].score;
printf("%10s %6.1f %c \n", list[i].code, list[i].score, list[i].grade);
}
average = sum / amount; // 计算平均成绩
printf(" 本班总人数 %d 本班平均成绩 %.1f\n", amount, average);
printf("\n\n\n\n\n ***** 摁任意键继续! ****** \n");
getch();
}
// 按总评成绩排序函数
void paixu(struct student list, int amount) {
for (int i = 0; i < amount; i++) {
for (int j = i + 1; j < amount; j++) {
if (list[j].score > list[i].score) {
struct student temp = list[j];
list[j] = list[i];
list[i] = temp;
}
}
}
view(list, amount); // 显示学生成绩
}
// 计算并显示成绩函数
void calculate(struct student list[], int amount) {
for(int i = 0; i < amount; i++) {
float score = list[i].s10.3 + list[i].s20.3 + list[i].s30.4; // 计算总评成绩
list[i].score = score; // 将总评成绩赋值给结构体中的score变量
if(score >= 90) { // 判断成绩等级
list[i].grade = 'A';
} else if(score >= 80) {
list[i].grade = 'B';
} else if(score >= 70) {
list[i].grade = 'C';
} else if(score >= 60) {
list[i].grade = 'D';
} else {
list[i].grade = 'E';
}
}
dispinfo(); // 显示信息
view(list, amount); // 显示学生成绩
}
相关推荐
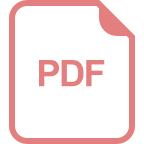
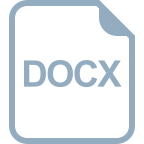
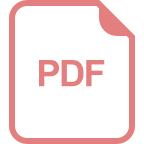












