bbox=mask.getbbox()
时间: 2024-05-28 09:11:34 浏览: 25
这行代码是用于获取一个二值化掩码图像中的最小边界框(bounding box)。在计算机视觉领域中,我们经常需要从一个二值化的图像中找到一个物体的轮廓,并将其包含在一个最小的矩形框中。这个矩形框就是最小边界框,它可以用来描述物体的位置和大小。在这个代码中,mask是一个二值化的图像,getbbox()函数会返回这个图像中的最小边界框,也就是包含所有像素的最小矩形框。这个边界框的坐标可以用来定位和裁剪出物体。
相关问题
var bbox = text.node().getBBox()
这段代码是用于获取SVG元素中文本节点的边界框(bounding box)信息。具体来说,它会返回一个包含四个属性的对象,分别是x、y、width和height,分别表示文本框的左上角坐标和宽高。这个对象可以帮助我们计算文本节点的位置和大小,以便进行后续的操作,比如对齐、布局等。需要注意的是,这段代码需要在文本节点被渲染到页面上后才能调用,否则可能会返回不准确的信息。
bbox = text.bbox("1.0", "end")出错
### 回答1:
这个错误可能是因为你在调用`text.bbox`方法时没有正确地传入参数。`bbox`方法的两个参数分别是起始位置和结束位置,用于指定要计算边界框的文本范围。通常,起始位置为 "1.0"(表示文本的第一行第一列),结束位置为 "end"(表示文本的最后一行最后一列)。如果你的文本框为空,也会导致这个错误。请检查一下你的代码,确保这两个问题都没有出现。
### 回答2:
当出现bbox = text.bbox("1.0", "end")出错时,可能有几个原因导致:
1. 错误的变量名或对象:首先需要确认是否正确初始化了"text"对象。如果没有正确创建一个名为"text"的文本对象,则可能会出现此错误。
2. 没有文本内容:如果"text"对象中没有任何文本内容,也会出现此错误。在使用bbox方法之前,需要确保文本对象中有一些文本内容。
3. 未正确导入模块:如果没有正确导入所需的模块,也会引发错误。在使用bbox方法之前,请确保正确导入了相应的模块。例如,对于Tkinter模块,需要导入相应的模块:from tkinter import *
4. 错误的调用方式:如果在调用bbox方法时传递了错误的参数,也会导致错误。bbox方法接受两个参数,表示文本的起始位置和结束位置。例如,"1.0"表示第一行第一个字符,"end"表示文本的末尾位置。
解决问题的方法可以是:
1. 确认是否已正确初始化"text"对象,并添加了一些文本内容。
2. 检查是否正确导入了所需的模块。
3. 确认bbox方法调用时传递了正确的参数,表示文本的起始位置和结束位置。
通过排除以上可能的原因,即可解决bbox = text.bbox("1.0", "end")出错的问题。
相关推荐
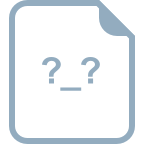
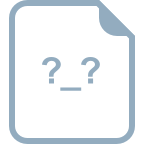
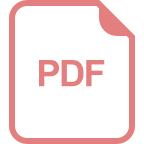













