centroids = np.zeros((K,n))
时间: 2023-11-29 15:31:12 浏览: 34
这行代码是在初始化 K 个聚类中心点的坐标为 0。其中,K 表示聚类中心点的数量,n 表示每个聚类中心点的坐标数。这里使用了 NumPy 库中的 zeros 函数,它会创建一个数组,该数组的元素全部为 0。对于这个聚类算法来说,每个聚类中心点都是一个 n 维坐标,因此使用了二维数组来存储所有聚类中心点的坐标。
相关问题
def findClosestCentroids(X, centroids): #定义函数findClosestCentroids """ Returns the closest centroids in idx for a dataset X where each row is a single example. """ K = centroids.shape[0] #获得数组centroids的行数并赋值给K idx = np.zeros((X.shape[0],1)) #定义idx为X.shape[0]行1列的零数组 temp = np.zeros((centroids.shape[0],1)) #定义temp为centroids.shape[0]行1列的数组 for i in range(X.shape[0]): #i遍历循环X.shape[0] for j in range(K): #j遍历循环K dist = X[i,:] - centroids[j,:] # length = np.sum(dist**2) temp[j] = length idx[i] = np.argmin(temp)+1 return idx 给这段代码注释
# 定义函数findClosestCentroids,它接受两个参数:数据集X和聚类中心centroids
# 函数的作用是为数据集中的每个样本找到距离它最近的聚类中心,并将其对应的聚类中心下标存储在idx中
# 获取聚类中心的数量K
K = centroids.shape[0]
# 初始化idx为X.shape[0]行1列的零数组
idx = np.zeros((X.shape[0],1))
# 初始化temp为centroids.shape[0]行1列的数组
temp = np.zeros((centroids.shape[0],1))
# 遍历数据集X中的每个样本
for i in range(X.shape[0]):
# 遍历每个聚类中心
for j in range(K):
# 计算当前样本到聚类中心的距离
dist = X[i,:] - centroids[j,:]
# 将距离的平方和存储在temp数组中
length = np.sum(dist**2)
temp[j] = length
# 找到距离当前样本最近的聚类中心下标,并将其加1存储在idx中
idx[i] = np.argmin(temp)+1
# 返回存储聚类中心下标的idx
return idx
def __init__(self, n_inputs, n_rules, learning_rate=0.01): self.n = n_inputs self.m = n_rules self.lr = learning_rate # Initialize MF parameters using k-means clustering kmeans = KMeans(n_clusters=1) x0 = [i for i in np.zeros(self.n)] kmeans.fit([x0]) centroids = kmeans.cluster_centers_.flatten() sigmas = np.ones(self.m) * (kmeans.inertia_ / self.m) ** 0.5 self.params = { "mf_params": np.random.rand(self.n*self.m*2), "out_params": np.random.rand((self.n+1)*self.m,) }修正错误
根据您提供的代码,可以将其修正如下:
```python
def __init__(self, n_inputs, n_rules, learning_rate=0.01):
self.n = n_inputs
self.m = n_rules
self.lr = learning_rate
# Initialize MF parameters using k-means clustering
kmeans = KMeans(n_clusters=self.m)
x0 = np.random.rand(100, self.n) # 用于聚类的样本点
kmeans.fit(x0)
centroids = kmeans.cluster_centers_ # 获取聚类中心
sigmas = np.ones(self.m) * (kmeans.inertia_ / self.m) ** 0.5 # 计算标准差
self.params = {
"mf_params": np.concatenate([centroids.flatten(), sigmas.flatten()]),
"out_params": np.random.rand((self.n+1)*self.m,)
}
```
在修正后的代码中,我们将k-means聚类的聚类数设置为`self.m`,并使用随机生成的样本点`x0`进行聚类。然后,我们通过`kmeans.cluster_centers_`获取每个聚类的中心点,并通过计算`sigmas`来确定高斯隶属度函数的标准差。最后,我们将所有的高斯隶属度函数参数连接起来,构建成一个包含所有参数的`self.params["mf_params"]`数组。
相关推荐
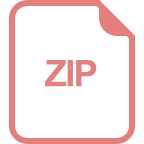
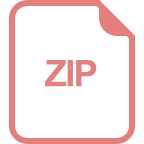













