如何处理UndefinedMetricWarning: Precision and F-score are ill-defined and being set to 0.0 in labels with no predicted samples. Use zero_division parameter to control this behavior.这一问题?请给出代码
时间: 2024-02-24 07:54:33 浏览: 199
在sklearn中,可以使用`classification_report`函数来计算分类指标,例如精度、召回率和F1分数。如果某个标签在预测结果中没有出现,就会出现上述警告信息。为了解决这个问题,可以使用`zero_division`参数将警告信息关闭,或者手动处理未预测标签的情况。
下面是一个例子,展示了如何使用`classification_report`函数,并将`zero_division`参数设置为1:
```python
from sklearn.metrics import classification_report
# y_true是真实标签,y_pred是预测标签
report = classification_report(y_true, y_pred, zero_division=1)
print(report)
```
如果你想手动处理未预测标签的情况,可以按照如下方式实现:
```python
from sklearn.metrics import precision_score, recall_score, f1_score
# y_true是真实标签,y_pred是预测标签
precision = precision_score(y_true, y_pred, labels=labels, average='macro', zero_division=1)
recall = recall_score(y_true, y_pred, labels=labels, average='macro', zero_division=1)
f1 = f1_score(y_true, y_pred, labels=labels, average='macro', zero_division=1)
# 处理未预测标签的情况
for label in labels:
if label not in y_pred:
precision[label] = 1
recall[label] = 1
f1[label] = 1
```
其中,`labels`是所有可能的标签列表,可以使用`np.unique`函数或手动指定。`average`参数可以设置为`macro`、`micro`或`weighted`,具体含义可以参考sklearn文档。
阅读全文
相关推荐

















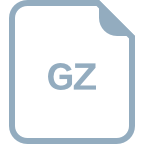
