data.drop('_id',axis=1, inplace=True)
时间: 2024-01-10 13:02:52 浏览: 101
你好!你的第一个问题是关于对数据进行处理的。根据你的要求,你想要去除数据中的"_id"列。为了实现这个目标,你可以使用`drop`函数,并设置`axis=1`来指定删除列。最后,使用`inplace=True`参数可以直接在原始数据上进行修改。以下是示例代码:
```python
data.drop('_id', axis=1, inplace=True)
```
请注意,这只是一个示例代码,你需要根据实际情况调整变量名和数据框名。如果你有任何其他问题,请随时提问!
相关问题
#setting index data = df.sort_index(ascending=True, axis=0) new_data = data[['trade_date', 'close']] new_data.index = new_data['trade_date'] new_data = new_data.drop('trade_date', axis=1).copy() #new_data.drop('trade_date', axis=1, inplace=True) new_data.head()仔细解读每行代码
好的,我来帮你解读每行代码:
1. `data = df.sort_index(ascending=True, axis=0)`:这一行代码是将原始数据按照索引(即时间)排序,且按照升序排列。
2. `new_data = data[['trade_date', 'close']]`:这一行代码是将排序后的数据中的"trade_date"和"close"这两列提取出来,赋值给一个新的DataFrame对象new_data。
3. `new_data.index = new_data['trade_date']`:这一行代码是将new_data的索引设置为"trade_date"列的值,即将时间作为索引。
4. `new_data = new_data.drop('trade_date', axis=1).copy()`:这一行代码是将"trade_date"这一列删除,因为已经将其作为索引,再保留这一列就会出现重复。同时,由于.drop()方法默认是不对原DataFrame进行修改的,因此这里使用.copy()方法来创建一个新的DataFrame对象,从而避免对原数据的影响。
5. `new_data.head()`:这一行代码是输出新的DataFrame对象new_data的前5行数据,以供查看。
优化代码 def cluster_format(self, start_time, end_time, save_on=True, data_clean=False, data_name=None): """ local format function is to format data from beihang. :param start_time: :param end_time: :return: """ # 户用簇级数据清洗 if data_clean: unused_index_col = [i for i in self.df.columns if 'Unnamed' in i] self.df.drop(columns=unused_index_col, inplace=True) self.df.drop_duplicates(inplace=True, ignore_index=True) self.df.reset_index(drop=True, inplace=True) dupli_header_lines = np.where(self.df['sendtime'] == 'sendtime')[0] self.df.drop(index=dupli_header_lines, inplace=True) self.df = self.df.apply(pd.to_numeric, errors='ignore') self.df['sendtime'] = pd.to_datetime(self.df['sendtime']) self.df.sort_values(by='sendtime', inplace=True, ignore_index=True) self.df.to_csv(data_name, index=False) # 调用基本格式化处理 self.df = super().format(start_time, end_time) module_number_register = np.unique(self.df['bat_module_num']) # if registered m_num is 0 and not changed, there is no module data if not np.any(module_number_register): logger.logger.warning("No module data!") sys.exit() if 'bat_module_voltage_00' in self.df.columns: volt_ref = 'bat_module_voltage_00' elif 'bat_module_voltage_01' in self.df.columns: volt_ref = 'bat_module_voltage_01' elif 'bat_module_voltage_02' in self.df.columns: volt_ref = 'bat_module_voltage_02' else: logger.logger.warning("No module data!") sys.exit() self.df.dropna(axis=0, subset=[volt_ref], inplace=True) self.df.reset_index(drop=True, inplace=True) self.headers = list(self.df.columns) # time duration of a cluster self.length = len(self.df) if self.length == 0: logger.logger.warning("After cluster data clean, no effective data!") raise ValueError("No effective data after cluster data clean.") self.cluster_stats(save_on) for m in range(self.mod_num): print(self.clusterid, self.mod_num) self.module_list.append(np.unique(self.df[f'bat_module_sn_{str(m).zfill(2)}'].dropna())[0])
Here are some possible optimizations for the given code:
1. Instead of using a list comprehension to find columns with 'Unnamed' in their names, you can use the `filter()` function along with a lambda function to achieve the same result in a more concise way:
```
unused_index_col = list(filter(lambda x: 'Unnamed' in x, self.df.columns))
```
2. Instead of dropping duplicates and resetting the index separately, you can use the `drop_duplicates()` function with the `ignore_index` parameter set to `True` to achieve both in one step:
```
self.df.drop_duplicates(inplace=True, ignore_index=True)
```
3. Instead of using `sys.exit()` to terminate the program when there is no module data, you can raise a `ValueError` with an appropriate error message:
```
raise ValueError("No module data!")
```
4. Instead of using a series of `if` statements to find the voltage reference column, you can use the `loc` accessor with a boolean mask to select the first column that starts with 'bat_module_voltage':
```
volt_ref_col = self.df.columns[self.df.columns.str.startswith('bat_module_voltage')][0]
```
5. Instead of using a loop to append a single item to a list, you can use the `append()` method directly:
```
self.module_list.append(np.unique(self.df[f'bat_module_sn_{str(m).zfill(2)}'].dropna())[0])
```
By applying these optimizations, the code can become more concise and efficient.
阅读全文
相关推荐
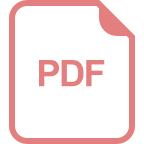
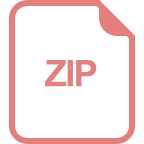
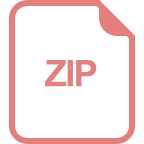














