x = F.threshold(-x, -1, -1)
时间: 2023-09-14 20:05:54 浏览: 37
这行代码使用了 PyTorch 中的阈值函数,将输入张量 x 中小于 -1 的值设置为 -1,大于等于 -1 的值保持不变。具体而言,函数的定义如下:
```
torch.threshold(input, threshold, value, inplace=False) -> Tensor
```
其中,
- input:输入张量
- threshold:阈值
- value:小于阈值的元素设置为该值
- inplace:是否原地操作,即是否把操作结果直接存储到输入张量中。默认为 False。
因此,该行代码的作用是将 x 中小于 -1 的元素替换为 -1。
相关问题
public Point2d RefineSubPixel(Mat image, Point2d lower, Point2d upper) { // 提取感兴趣区域 Rect roiRect = new Rect((int)lower.X, (int)lower.Y, (int)(upper.X - lower.X), (int)(upper.Y - lower.Y)); Mat roi = new Mat(image, roiRect); // 初始化初始点 Point2d refinedPoint = new Point2d(roi.Cols / 2.0, roi.Rows / 2.0); // 定义优化终止标准 var termCriteria = new TermCriteria(CriteriaTypes.MaxIter | CriteriaTypes.Eps, 20, 0.03); // 执行优化迭代 if (roi.Width > 1 && roi.Height > 1) { // 预处理 var grayRoi = new Mat(); Cv2.PyrMeanShiftFiltering(roi, roi, 2, 2); Cv2.CvtColor(roi, grayRoi, ColorConversionCodes.BGR2GRAY); Cv2.Threshold(grayRoi, grayRoi, 0, 255, ThresholdTypes.Binary | ThresholdTypes.Otsu); // 迭代更新点坐标 var delta = new Point2d(); var point = new Point2d(refinedPoint.X, refinedPoint.Y); var bestPoint = new Point2d(refinedPoint.X, refinedPoint.Y); var width = image.Cols; var height = image.Rows; var targetGray = grayRoi.At<byte>((int)point.Y, (int)point.X); var minError = double.MaxValue; var precision = 1e-6; for (int i = 0; i < termCriteria.MaxCount; i++) { int x = (int)Math.Round(point.X); int y = (int)Math.Round(point.Y); if (x <= 0 || y <= 0 || x >= grayRoi.Cols - 1 || y >= grayRoi.Rows - 1) { break; } // 计算当前点周围的梯度信息 var derivX = (grayRoi.At<byte>(y, x + 1) - grayRoi.At<byte>(y, x - 1)) / 2.0; var derivY = (grayRoi.At<byte>(y + 1, x) - grayRoi.At<byte>(y - 1, x)) / 2.0; var hessian = new Mat(2, 2, MatType.CV_64F); hessian.Set<double>(0, 0, grayRoi.At<byte>(y, x + 1) + grayRoi.At<byte>(y, x - 1) - 2 * grayRoi.At<byte>(y, x)); hessian.Set<double>(0, 1, (grayRoi.At<byte>(y + 1, x + 1) - grayRoi.At<byte>(y + 1, x - 1) - grayRoi.At<byte>(y - 1, x + 1) + grayRoi.At<byte>(y - 1, x - 1)) / 4.0); hessian.Set<double>(1, 0, hessian.At<double>(0, 1)); hessian.Set<double>(1, 1, grayRoi.At<byte请完善代码
>(y + 1, x) + grayRoi.At<byte>(y - 1, x) - 2 * grayRoi.At<byte>(y, x)); // 求解线性方程组,更新点坐标 var deltaMat = new Mat(2, 1, MatType.CV_64F); deltaMat.Set<double>(0, 0, derivX); deltaMat.Set<double>(1, 0, derivY); var hessianInv = hessian.Inv(); var deltaPoint = hessianInv * deltaMat; delta.X = deltaPoint.At<double>(0, 0); delta.Y = deltaPoint.At<double>(1, 0); point -= delta; point.X = Math.Max(Math.Min(point.X, roi.Cols - 1), 0); point.Y = Math.Max(Math.Min(point.Y, roi.Rows - 1), 0); // 判断是否收敛 var currentGray = grayRoi.At<byte>((int)point.Y, (int)point.X); if (Math.Abs(currentGray - targetGray) < minError) { minError = Math.Abs(currentGray - targetGray); bestPoint = new Point2d(point.X, point.Y); } if (Math.Sqrt(delta.X * delta.X + delta.Y * delta.Y) < precision) { break; } } refinedPoint = bestPoint + new Point2d(lower.X, lower.Y); } return refinedPoint; }
这段代码是用于对图像中某个区域内的点进行亚像素级别的精确定位。具体实现过程是通过迭代优化,计算当前点周围的梯度信息和Hessian矩阵,然后求解线性方程组并更新点坐标,直到达到优化终止标准为止。
其中,先通过PyrMeanShiftFiltering函数对感兴趣区域进行预处理,然后再用CvtColor函数将其转换为灰度图像,接着用Threshold函数对其进行二值化处理。在迭代过程中,还需要判断当前点是否在图像边界内,以及判断是否达到优化终止标准。最后返回经过优化后的精确点坐标。
###function approximation f(x)=sin(x) ###2018.08.14 ###激活函数用的是sigmoid import numpy as np import math import matplotlib.pyplot as plt x = np.linspace(-3, 3, 600) # print(x) # print(x[1]) x_size = x.size y = np.zeros((x_size, 1)) # print(y.size) for i in range(x_size): y[i] = math.sin(2*math.pi*0.4*x[i])+ math.sin(2*math.pi*0.1*x[i]) + math.sin(2*math.pi*0.9*x[i]) # print(y) hidesize = 10 W1 = np.random.random((hidesize, 1)) # 输入层与隐层之间的权重 B1 = np.random.random((hidesize, 1)) # 隐含层神经元的阈值 W2 = np.random.random((1, hidesize)) # 隐含层与输出层之间的权重 B2 = np.random.random((1, 1)) # 输出层神经元的阈值 threshold = 0.005 max_steps = 1001 def sigmoid(x_): y_ = 1 / (1 + math.exp(-x_)) return y_ E = np.zeros((max_steps, 1)) # 误差随迭代次数的变化 Y = np.zeros((x_size, 1)) # 模型的输出结果 for k in range(max_steps): temp = 0 for i in range(x_size): hide_in = np.dot(x[i], W1) - B1 # 隐含层输入数据 # print(x[i]) hide_out = np.zeros((hidesize, 1)) # 隐含层的输出数据 for j in range(hidesize): # print("第{}个的值是{}".format(j,hide_in[j])) # print(j,sigmoid(j)) hide_out[j] = sigmoid(hide_in[j]) # print("第{}个的值是{}".format(j, hide_out[j])) # print(hide_out[3]) y_out = np.dot(W2, hide_out) - B2 # 模型输出 # print(y_out) Y[i] = y_out # print(i,Y[i]) e = y_out - y[i] # 模型输出减去实际结果。得出误差 ##反馈,修改参数 dB2 = -1 * threshold * e dW2 = e * threshold * np.transpose(hide_out) dB1 = np.zeros((hidesize, 1)) for j in range(hidesize): dB1[j] = np.dot(np.dot(W2[0][j], sigmoid(hide_in[j])), (1 - sigmoid(hide_in[j])) * (-1) * e * threshold) dW1 = np.zeros((hidesize, 1)) for j in range(hidesize): dW1[j] = np.dot(np.dot(W2[0][j], sigmoid(hide_in[j])), (1 - sigmoid(hide_in[j])) * x[i] * e * threshold) W1 = W1 - dW1 B1 = B1 - dB1 W2 = W2 - dW2 B2 = B2 - dB2 temp = temp + abs(e) E[k] = temp if k % 100 == 0: print(k) plt.figure() plt.plot(x, Y) plt.plot(x, Y, color='red', linestyle='--') plt.show()这个程序如何每迭代100次就输出一次图片
要每迭代100次输出一次图片,你需要在代码中添加相应的逻辑。以下是修改后的代码示例:
```python
import numpy as np
import math
import matplotlib.pyplot as plt
x = np.linspace(-3, 3, 600)
x_size = x.size
y = np.zeros((x_size, 1))
for i in range(x_size):
y[i] = math.sin(2*math.pi*0.4*x[i]) + math.sin(2*math.pi*0.1*x[i]) + math.sin(2*math.pi*0.9*x[i])
hidesize = 10
W1 = np.random.random((hidesize, 1))
B1 = np.random.random((hidesize, 1))
W2 = np.random.random((1, hidesize))
B2 = np.random.random((1, 1))
threshold = 0.005
max_steps = 1001
def sigmoid(x_):
y_ = 1 / (1 + math.exp(-x_))
return y_
E = np.zeros((max_steps, 1))
Y = np.zeros((x_size, 1))
for k in range(max_steps):
temp = 0
for i in range(x_size):
hide_in = np.dot(x[i], W1) - B1
hide_out = np.zeros((hidesize, 1))
for j in range(hidesize):
hide_out[j] = sigmoid(hide_in[j])
y_out = np.dot(W2, hide_out) - B2
Y[i] = y_out
e = y_out - y[i]
dB2 = -1 * threshold * e
dW2 = e * threshold * np.transpose(hide_out)
dB1 = np.zeros((hidesize, 1))
for j in range(hidesize):
dB1[j] = np.dot(np.dot(W2[0][j], sigmoid(hide_in[j])), (1 - sigmoid(hide_in[j])) * (-1) * e * threshold)
dW1 = np.zeros((hidesize, 1))
for j in range(hidesize):
dW1[j] = np.dot(np.dot(W2[0][j], sigmoid(hide_in[j])), (1 - sigmoid(hide_in[j])) * x[i] * e * threshold)
W1 = W1 - dW1
B1 = B1 - dB1
W2 = W2 - dW2
B2 = B2 - dB2
temp = temp + abs(e)
E[k] = temp
if k % 100 == 0:
plt.figure()
plt.plot(x, Y)
plt.plot(x, Y, color='red', linestyle='--')
plt.savefig(f'iteration_{k}.png')
plt.close()
plt.figure()
plt.plot(x, Y)
plt.plot(x, Y, color='red', linestyle='--')
plt.show()
```
在上述示例中,我添加了一个条件判断语句`if k % 100 == 0`来判断是否达到每100次迭代的条件。如果满足条件,则在该迭代结束后绘制并保存图片,文件名为`iteration_k.png`,其中`k`表示当前迭代次数。
请根据你的具体需求和编程环境进行相应的调整。
相关推荐
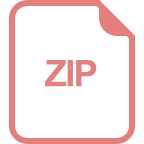
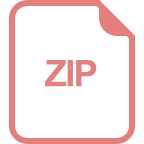
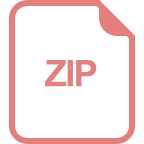












