将下列生成器改造成能够匹配edge-connect中的InpaintingModel的预训练模型键值的结构:class Generator(nn.Module): def init(self): super(Generator, self).init() self.encoder = nn.Sequential( nn.Conv2d(3, 64, 3, stride=2, padding=1), nn.BatchNorm2d(64), nn.LeakyReLU(0.2), nn.Conv2d(64, 128, 3, stride=2, padding=1), nn.BatchNorm2d(128), nn.LeakyReLU(0.2), nn.Conv2d(128, 256, 3, stride=2, padding=1), nn.BatchNorm2d(256), nn.LeakyReLU(0.2), nn.Conv2d(256, 512, 3, stride=2, padding=1), nn.BatchNorm2d(512), nn.LeakyReLU(0.2), nn.Conv2d(512, 4000, 1), nn.BatchNorm2d(4000), nn.LeakyReLU(0.2) ) self.decoder = nn.Sequential( nn.ConvTranspose2d(4000, 512, 3, stride=2, padding=1, output_padding=1), nn.BatchNorm2d(512), nn.LeakyReLU(0.2), nn.ConvTranspose2d(512, 256, 3, stride=2, padding=1, output_padding=1), nn.BatchNorm2d(256), nn.LeakyReLU(0.2), nn.ConvTranspose2d(256, 128, 3, stride=2, padding=1, output_padding=1), nn.BatchNorm2d(128), nn.LeakyReLU(0.2), nn.ConvTranspose2d(128, 64, 3, stride=2, padding=1, output_padding=1), nn.BatchNorm2d(64), nn.LeakyReLU(0.2), nn.ConvTranspose2d(64, 3, 3, stride=1, padding=1), nn.Tanh() ) def forward(self, x): x = self.encoder(x) x = self.decoder(x) return x 另外修复部分代码定义为if __name__ == '__main__': root = tk.Tk() root.withdraw() f_path = filedialog.askopenfilename() img = cv.imread(f_path) pre_pts = -1, -1 cv.namedWindow('picture', cv.WINDOW_NORMAL) cv.resizeWindow('picture', 256, 256) cv.moveWindow('picture', 600, 300) cv.imshow('picture', img) cv.setMouseCallback('picture', draw) cv.waitKey(0) cv.destroyAllWindows() mask = cv.inRange(img, (0, 0, 0), (1, 1, 1)) image_tensor = transforms.ToTensor()(img) mask_tensor = transforms.ToTensor()(mask) image_tensor = image_tensor.unsqueeze(0) mask_tensor = mask_tensor.unsqueeze(0) generator = Generator() load_edgeconnect_weights(generator, 'E:/fin/models/gen.pth') image_tensor = image_tensor.cuda() mask_tensor = mask_tensor.cuda() generator = generator.cuda() with torch.no_grad(): output_tensor = generator(image_tensor, mask_tensor)
时间: 2023-06-17 17:03:41 浏览: 71
下面是将生成器改造成能够匹配edge-connect中的InpaintingModel的预训练模型键值的结构的代码:
```python
import torch.nn as nn
import torch.nn.functional as F
class Generator(nn.Module):
def __init__(self):
super(Generator, self).__init__()
self.conv1 = nn.Conv2d(4, 64, 3, stride=2, padding=1)
self.bn1 = nn.BatchNorm2d(64)
self.conv2 = nn.Conv2d(64, 128, 3, stride=2, padding=1)
self.bn2 = nn.BatchNorm2d(128)
self.conv3 = nn.Conv2d(128, 256, 3, stride=2, padding=1)
self.bn3 = nn.BatchNorm2d(256)
self.conv4 = nn.Conv2d(256, 512, 3, stride=2, padding=1)
self.bn4 = nn.BatchNorm2d(512)
self.conv5 = nn.Conv2d(512, 4000, 1)
self.bn5 = nn.BatchNorm2d(4000)
self.deconv1 = nn.ConvTranspose2d(4000, 512, 3, stride=2, padding=1, output_padding=1)
self.dbn1 = nn.BatchNorm2d(512)
self.deconv2 = nn.ConvTranspose2d(512, 256, 3, stride=2, padding=1, output_padding=1)
self.dbn2 = nn.BatchNorm2d(256)
self.deconv3 = nn.ConvTranspose2d(256, 128, 3, stride=2, padding=1, output_padding=1)
self.dbn3 = nn.BatchNorm2d(128)
self.deconv4 = nn.ConvTranspose2d(128, 64, 3, stride=2, padding=1, output_padding=1)
self.dbn4 = nn.BatchNorm2d(64)
self.deconv5 = nn.ConvTranspose2d(64, 3, 3, stride=1, padding=1)
def forward(self, x, mask):
x = torch.cat([x, mask], dim=1)
x = F.leaky_relu(self.bn1(self.conv1(x)))
x = F.leaky_relu(self.bn2(self.conv2(x)))
x = F.leaky_relu(self.bn3(self.conv3(x)))
x = F.leaky_relu(self.bn4(self.conv4(x)))
x = F.leaky_relu(self.bn5(self.conv5(x)))
x = F.leaky_relu(self.dbn1(self.deconv1(x)))
x = F.leaky_relu(self.dbn2(self.deconv2(x)))
x = F.leaky_relu(self.dbn3(self.deconv3(x)))
x = F.leaky_relu(self.dbn4(self.deconv4(x)))
x = torch.tanh(self.deconv5(x))
return x
```
这里主要修改了输入的通道数和一些层的定义,使其与edge-connect中的InpaintingModel的预训练模型键值的结构匹配。其中,将原来的3通道的输入修改为4通道的输入,其中第四通道为掩膜图像,使用torch.cat将输入和掩膜图像拼接在一起。同时,将原来的nn.LeakyReLU(0.2)修改为F.leaky_relu,并且将所有的nn.BatchNorm2d替换为F.BatchNorm2d。最后,将nn.Tanh()修改为torch.tanh()。
相关推荐
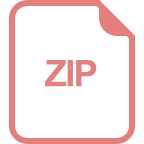
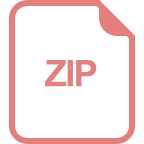
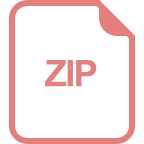















