如何修改,使这段代码将数据集划分为训练集和测试集进行训练和预测,并采用交叉验证等方法来进一步验证模型的鲁棒性和泛化能力
时间: 2024-03-23 11:40:20 浏览: 25
可以参考以下步骤来修改代码,将数据集划分为训练集和测试集进行训练和预测,并采用交叉验证等方法来进一步验证模型的鲁棒性和泛化能力:
1. 将所有数据分为训练集和测试集,可以使用sklearn库中的train_test_split函数,将数据按照一定比例划分为训练集和测试集。
2. 在训练集上训练模型,使用交叉验证等方法进行模型选择和调参,可以使用sklearn库中的GridSearchCV、KFold等函数。
3. 在测试集上对训练好的模型进行预测,计算模型的性能指标,如均方误差、R2等。
下面是修改后的代码:
```
import os
import pandas as pd
from sklearn.neighbors import KNeighborsRegressor
from sklearn.model_selection import train_test_split, GridSearchCV, KFold
from sklearn.metrics import r2_score, mean_squared_error
# 读取第一个文件夹中的所有csv文件
folder1_path = "/path/to/folder1"
files1 = os.listdir(folder1_path)
dfs1 = []
for file1 in files1:
if file1.endswith(".csv"):
file1_path = os.path.join(folder1_path, file1)
df1 = pd.read_csv(file1_path, usecols=[1,2,3,4])
dfs1.append(df1)
# 将第一个文件夹中的所有数据合并为一个DataFrame
df_X = pd.concat(dfs1, ignore_index=True)
# 读取第二个文件夹中的所有csv文件
folder2_path = "/path/to/folder2"
files2 = os.listdir(folder2_path)
dfs2 = []
for file2 in files2:
if file2.endswith(".csv"):
file2_path = os.path.join(folder2_path, file2)
df2 = pd.read_csv(file2_path, usecols=[1])
dfs2.append(df2)
# 将第二个文件夹中的所有数据合并为一个DataFrame
df_X["X5"] = pd.concat(dfs2, ignore_index=True)
# 读取第三个文件夹中的所有csv文件
folder3_path = "/path/to/folder3"
files3 = os.listdir(folder3_path)
dfs3 = []
for file3 in files3:
if file3.endswith(".csv"):
file3_path = os.path.join(folder3_path, file3)
df3 = pd.read_csv(file3_path, usecols=[2,6])
dfs3.append(df3)
# 将第三个文件夹中的所有数据合并为一个DataFrame
df_y = pd.concat(dfs3, ignore_index=True)
# 将数据集划分为训练集和测试集
X_train, X_test, y_train, y_test = train_test_split(df_X, df_y, test_size=0.2, random_state=42)
# 训练k邻近回归模型
k = 5
model = KNeighborsRegressor(n_neighbors=k)
# 使用交叉验证进行模型选择和调参
param_grid = {'n_neighbors': [3, 5, 7, 9]}
kf = KFold(n_splits=5, shuffle=True, random_state=42)
grid_search = GridSearchCV(model, param_grid, cv=kf, scoring='neg_mean_squared_error')
grid_search.fit(X_train, y_train)
# 在测试集上对训练好的模型进行预测
y_pred = grid_search.predict(X_test)
# 计算模型的性能指标
r2 = r2_score(y_test, y_pred)
mse = mean_squared_error(y_test, y_pred)
print("模型准确率(R2):", r2)
print("模型均方误差(MSE):", mse)
```
这个修改后的代码将数据集划分为训练集和测试集,并使用交叉验证进行模型选择和调参,最后在测试集上对训练好的模型进行预测,计算了模型的性能指标(R2和MSE)。
相关推荐
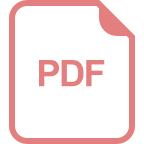
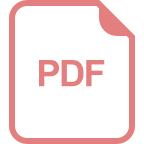














