解释probability prediction at means和the average of probability prediction的差别
时间: 2024-06-03 22:10:12 浏览: 109
在自然语言处理中,probability prediction at means指的是在给定一组训练数据的情况下,预测模型对于某个输入句子所预测出来的所有可能性的平均分布,这个平均分布可以用来确定每个可能性的概率。而the average of probability prediction则是指在给定一个大量的输入句子的情况下,预测模型对于所有句子的可能性分布的平均值,这个平均值可以用来判断整体的预测表现如何。两者的区别在于前者是基于单个输入句子的预测结果,后者则是对于多个句子进行整体分析得出的结论。
相关问题
prediction interval coverage probability
Prediction interval coverage probability refers to the probability that a prediction interval will contain the true value of the dependent variable for a new observation. In other words, if we were to repeat the process of estimating a prediction interval for a new observation many times, the coverage probability would be the proportion of those intervals that actually contain the true value of the dependent variable. Ideally, the coverage probability should be close to the nominal level of the prediction interval, which is typically set at 95% or 99%. If the coverage probability is lower than the nominal level, it suggests that the prediction interval is too narrow and does not account for all sources of variability in the data. On the other hand, if the coverage probability is higher than the nominal level, it suggests that the prediction interval is too wide and may not be very useful for making predictions.
将Excel文件转换为csv文件,并将表头的id,lable,probability更改为uuid,prediction,proba
要将Excel文件转换为CSV文件并重命名列标题,你可以使用Python的`pandas`库。首先确保已安装了`openpyxl`(用于读取Excel)和`pandas`(用于数据处理)。以下是一段示例代码:
```python
import pandas as pd
from uuid import uuid4
# 读取Excel文件
xls = pd.read_excel('input.xlsx', engine='openpyxl') # 替换 'input.xlsx' 为你实际的Excel文件路径
# 重命名列标题
xls = xls.rename(columns={'id': 'uuid', 'lable': 'prediction', 'probability': 'proba'})
# 将DataFrame保存为CSV文件
xls.to_csv('output.csv', index=False) # 替换 'output.csv' 为你希望输出的CSV文件路径
# 如果你想创建UUID列而不是简单地重命名id,可以在rename之前生成一个新的列:
# xls['uuid'] = xls['id'].apply(lambda x: str(uuid4()))
```
这段代码做了以下几件事:
1. 使用`read_excel`函数从Excel文件加载数据到一个`pandas` DataFrame。
2. 利用`rename`函数更改列名,注意这里用到了字典形式的参数,键是原列名,值是新列名。
3. 最后使用`to_csv`函数将更新后的DataFrame保存为CSV文件。
如果你需要的是在每个行的'id'列上生成新的UUID,记得在`rename`之前添加一行生成新的'uuid'列的代码。
相关推荐
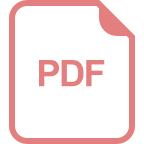
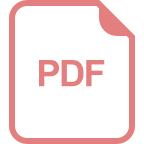
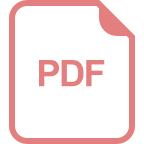












