image_t.unsqueeze_(0)
时间: 2024-05-17 20:19:01 浏览: 157
这行代码的作用是在 Tensor 类型的图像数据中增加一个维度,作为 batch_size。具体来说,输入的 Tensor 类型的图像数据可能是三维的,例如 shape 为 (3, 224, 224),其中 3 表示通道数,224 表示图像的高度和宽度。
而深度学习模型的输入通常是四维的,例如 shape 为 (1, 3, 224, 224),其中第一个维度表示 batch_size,也就是输入的图像数量。因此,需要将输入的 Tensor 类型的图像数据扩展一个维度作为 batch_size,这样才能符合模型的输入要求。
在这里,使用了 unsqueeze_() 函数来在原有 Tensor 类型的图像数据的第一个维度上增加一个维度。由于 unsqueeze_() 函数直接在原有 Tensor 上操作,并返回了修改后的 Tensor,因此使用了 in-place 操作符 _,即 unsqueeze_()。
最终,Tensor 类型的图像数据的 shape 变成了 (1, 3, 224, 224),它可以作为一个 batch 中的一个样本输入到深度学习模型中进行计算。
相关问题
from skimage.segmentation import slic, mark_boundaries import torchvision.transforms as transforms import numpy as np from PIL import Image import matplotlib.pyplot as plt import torch.nn as nn import torch # 定义超像素池化层 class SuperpixelPooling(nn.Module): def init(self, n_segments): super(SuperpixelPooling, self).init() self.n_segments = n_segments def forward(self, x): # 使用 SLIC 算法生成超像素标记图 segments = slic(x.numpy().transpose(1, 2, 0), n_segments=self.n_segments, compactness=10) # 将超像素标记图转换为张量 segments_tensor = torch.from_numpy(segments).unsqueeze(0).unsqueeze(0) # 将张量 x 与超像素标记图张量 segments_tensor 进行逐元素相乘 pooled = x * segments_tensor.float() # 在超像素维度上进行最大池化 pooled = nn.AdaptiveMaxPool2d((self.n_segments, 1))(pooled) # 压缩超像素维度 pooled = pooled.squeeze(3) # 返回池化后的特征图 return pooled # 加载图像 image = Image.open('3.jpg') # 转换为 PyTorch 张量 transform = transforms.ToTensor() img_tensor = transform(image).unsqueeze(0) # 将 PyTorch 张量转换为 Numpy 数组 img_np = img_tensor.numpy().transpose(0, 2, 3, 1)[0] # 使用 SLIC 算法生成超像素标记图 segments = slic(img_np, n_segments=60, compactness=10) # 将超像素标记图转换为张量 segments_tensor = torch.from_numpy(segments).unsqueeze(0).float() # 将超像素索引映射可视化 plt.imshow(segments, cmap='gray') plt.show() # 将 Numpy 数组转换为 PIL 图像 segment_img = Image.fromarray((mark_boundaries(img_np, segments) * 255).astype(np.uint8)) # 保存超像素索引映射可视化 segment_img.save('segment_map.jpg') # 使用超像素池化层进行池化 pooling_layer = SuperpixelPooling(n_segments=60) pooled_tensor = pooling_layer(img_tensor) # 将超像素池化后的特征图可视化 plt.imshow(pooled_tensor.squeeze().numpy().transpose(1, 0), cmap='gray') plt.show() ,上述代码出现问题:segments = slic(x.numpy().transpose(1, 2, 0), n_segments=self.n_segments, compactness=10) ValueError: axes don't match array,如何修改
这个错误通常是由于输入的张量维度不匹配导致的。在这里,可能是由于输入张量的维度不是按照预期的顺序排列导致的。建议检查一下输入张量 x 的维度是否为 (batch_size, channels, height, width),如果不是,可以使用 PyTorch 的 permute 函数重新排列维度。您可以尝试将这一行代码修改为:
```
segments = slic(x.permute(0, 2, 3, 1).numpy(), n_segments=self.n_segments, compactness=10)
```
这样可以将维度从 (batch_size, channels, height, width) 转换为 (batch_size, height, width, channels),以适应 SLIC 函数的输入要求。
Traceback (most recent call last): File "E:\xiangmu\measure\未命名1.py", line 66, in <module> test_image = transform(test_image).unsqueeze(0) File "E:\anaconda\lib\site-packages\torchvision\transforms\transforms.py", line 95, in __call__ img = t(img) File "E:\anaconda\lib\site-packages\torch\nn\modules\module.py", line 1501, in _call_impl return forward_call(*args, **kwargs) File "E:\anaconda\lib\site-packages\torchvision\transforms\transforms.py", line 361, in forward return F.resize(img, self.size, self.interpolation, self.max_size, self.antialias) File "E:\anaconda\lib\site-packages\torchvision\transforms\functional.py", line 476, in resize _, image_height, image_width = get_dimensions(img) File "E:\anaconda\lib\site-packages\torchvision\transforms\functional.py", line 78, in get_dimensions return F_pil.get_dimensions(img) File "E:\anaconda\lib\site-packages\torchvision\transforms\_functional_pil.py", line 31, in get_dimensions raise TypeError(f"Unexpected type {type(img)}") TypeError: Unexpected type <class 'str'>
这个错误是因为 `transform` 函数期望的输入是一个 PIL 图像对象,但你给它传递了一个字符串类型的参数。
要解决这个问题,你需要先将字符串路径转换为 PIL 图像对象,然后再将其传递给 `transform` 函数。可以使用 `PIL.Image` 模块中的 `open` 函数来打开图像文件并转换为 PIL 图像对象。
以下是一个示例代码:
```python
from PIL import Image
from torchvision import transforms
# 定义图像转换
transform = transforms.Compose([
transforms.Resize((224, 224)), # 调整图像大小为 224x224
transforms.ToTensor() # 将图像转换为张量
])
# 打开图像文件并转换为 PIL 图像对象
image_path = "path/to/image.jpg"
image = Image.open(image_path)
# 对图像进行转换
transformed_image = transform(image).unsqueeze(0)
```
请根据你的实际需求修改代码,并确保通过 `Image.open` 函数将图像路径转换为 PIL 图像对象。如果问题仍然存在,请提供更多详细信息,以便我能够提供更具体的帮助。
阅读全文
相关推荐
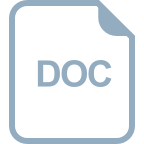
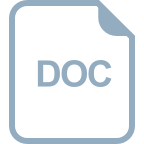
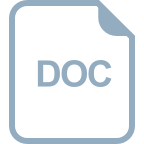



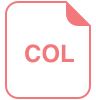
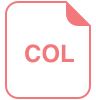
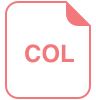







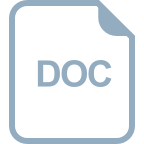