Dense(32, activation = 'relu',kernel_regularizer = l2(1e-5))用pytorch怎么写
时间: 2023-12-14 09:39:04 浏览: 24
在 PyTorch 中,可以使用 `nn.Sequential` 以及 `nn.Linear` 模块来实现 Dense 层。同时,可以在 `nn.Linear` 模块中设置激活函数和正则化项。
以下是一个示例代码:
```
import torch
import torch.nn as nn
model = nn.Sequential(
nn.Linear(in_features=输入特征数, out_features=32),
nn.ReLU(),
nn.Linear(in_features=32, out_features=输出特征数),
nn.Dropout(0.5),
)
# 添加正则化项
model[0].weight_regularizer = nn.L2Loss()
# 打印模型结构
print(model)
```
其中,`输入特征数` 和 `输出特征数` 分别为输入和输出张量的形状。在第一个 `nn.Linear` 模块中,输入特征数为输入张量的形状,而输出特征数为 32。在第二个 `nn.Linear` 模块中,输入特征数为 32,而输出特征数为输出张量的形状。`nn.Dropout` 模块用于随机失活,避免过拟合。
`nn.Linear` 模块中的 `weight_regularizer` 属性可以设置正则化项,这里使用的是 L2 正则化,正则化系数为 1e-5。在 PyTorch 中,L2 正则化可以使用 `nn.L2Loss()` 实现,也可以使用 `torch.norm()` 函数计算 L2 范数。
相关问题
x = Dense(32, activation = 'relu',kernel_regularizer = l2(1e-5))(x) x = Dense(16, activation = 'relu',kernel_regularizer = l2(1e-5))(x) x = Dense(8, activation = 'relu',kernel_regularizer = l2(1e-5))用pytorch咋写
这段代码在 PyTorch 中对应的实现可以如下:
```python
import torch.nn as nn
x = nn.Linear(in_features, 32)(x)
x = nn.ReLU()(x)
x = nn.Linear(32, 16)(x)
x = nn.ReLU()(x)
x = nn.Linear(16, 8)(x)
x = nn.ReLU()(x)
x = nn.Linear(8, out_features)(x)
```
其中,`in_features` 和 `out_features` 分别是输入和输出的特征数,可以根据具体需要进行修改。`nn.Linear` 对应 Keras 中的 `Dense` 层,`nn.ReLU` 对应 Keras 中的 `activation='relu'` 参数,`kernel_regularizer` 对应 PyTorch 中的正则化,可以通过添加 `nn.Dropout` 层实现 dropout 正则化。
model = densenet121(pretrained=True) num_ftrs = model.classifier.in_features model.classifier = nn.Linear(num_ftrs, 4)与K.set_learning_phase(0) base_model = DenseNet121(weights='imagenet', include_top=False, input_shape=(224, 224, 3), ) for layer in base_model.layers: layer.trainable=False K.set_learning_phase(1) x = base_model.output x = layers.GlobalMaxPooling2D()(x) # let's add a fully-connected layer x = layers.Dense(512, activation='relu', kernel_regularizer=regularizers.l2(0.0001))(x) x = layers.Dense(128, activation='relu', kernel_regularizer=regularizers.l2(0.0001))(x) # and a logistic l # ayer -- let's say we have 200 classes predictions = layers.Dense(4, activation='softmax')(x) model = Model(inputs=base_model.input, outputs=predictions) model.summary()
这是两个不同的深度学习模型的代码。第一个是使用PyTorch实现的DenseNet121模型,将其预训练的分类器替换为一个具有4个输出类的线性层。第二个是使用Keras实现的DenseNet121模型,该模型在ImageNet数据集上进行了预训练,但将其顶部的分类层移除,并添加了几个全连接层和一个softmax层,以适应另一个具有4个输出类的任务。这两个模型采用不同的框架和实现方式,但都使用了DenseNet121作为基础模型,并且都是用于分类任务。
相关推荐
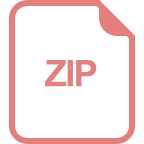
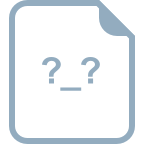
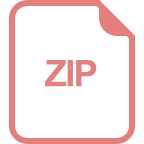












