base_model = tf.keras.applications.MobileNet(weights = "imagenet", include_top = False, input_shape = input_shape) base_model.trainable = False inputs = keras.Input(shape = input_shape) x = base_model(inputs, training = False) x = tf.keras.layers.GlobalAveragePooling2D()(x) x = tf.keras.layers.Dropout(0.2)(x) x = tf.keras.layers.Dense(len(categories), activation="softmax")(x) model = keras.Model(inputs = inputs, outputs = x, name="LeafDisease_MobileNet") weight_path = os.path.join(base_dir, 'checkpoints', 'my_checkpoint') model.load_weights(weight_path) img = plt.imread(img_path) img = img / 255. img = cv2.resize(img, (224, 224)) img = img.reshape(-1, 224, 224, 3) img.astype('float32') result = model.predict(img) cate_result = categories[np.argmax(result, axis=1)[0]] return cate_result可以详细解释一下每行代码的作用吗
时间: 2023-11-09 14:05:30 浏览: 258
当然可以。
1. `base_model = tf.keras.applications.MobileNet(weights="imagenet", include_top=False, input_shape=input_shape)`: 加载MobileNet模型,其中`weights="imagenet"`表示使用ImageNet预训练权重,`include_top=False`表示不包括顶层全连接层,`input_shape`表示输入图像的大小。
2. `base_model.trainable = False`: 将MobileNet模型的参数冻结,不参与训练。
3. `inputs = keras.Input(shape=input_shape)`: 定义输入张量。
4. `x = base_model(inputs, training=False)`: 将输入张量通过MobileNet模型得到特征张量。
5. `x = tf.keras.layers.GlobalAveragePooling2D()(x)`: 对特征张量进行全局平均池化操作。
6. `x = tf.keras.layers.Dropout(0.2)(x)`: 对全局平均池化后的特征张量进行Dropout操作。
7. `x = tf.keras.layers.Dense(len(categories), activation="softmax")(x)`: 添加一个全连接层,输出类别概率。
8. `model = keras.Model(inputs=inputs, outputs=x, name="LeafDisease_MobileNet")`: 将输入张量和输出张量封装成一个模型。
9. `weight_path = os.path.join(base_dir, 'checkpoints', 'my_checkpoint')`: 定义权重文件路径。
10. `model.load_weights(weight_path)`: 加载预训练好的权重。
11. `img = plt.imread(img_path)`: 读取待分类的图像。
12. `img = img / 255.`: 将图像像素值从[0,255]归一化到[0,1]。
13. `img = cv2.resize(img, (224, 224))`: 将图像缩放到MobileNet模型能够接受的大小。
14. `img = img.reshape(-1, 224, 224, 3)`: 将图像变形为模型需要的4维张量。
15. `img.astype('float32')`: 将图像数据类型转换为float32类型。
16. `result = model.predict(img)`: 对图像进行预测,得到类别概率。
17. `cate_result = categories[np.argmax(result, axis=1)[0]]`: 取最大概率对应的类别,返回类别名称。其中`np.argmax(result, axis=1)`表示取每个样本预测概率最大的下标,`[0]`表示取第一个样本。
相关推荐
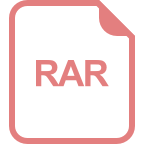
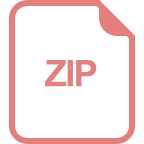
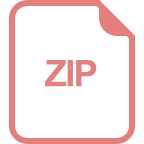














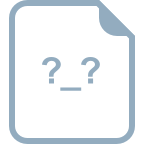