clf = LR(random_state = 0).fit(X_train, y_train) clf.predict(X_train.iloc[:2, :])#X_train是数据框类型,需要加iloc clf.predict(X_test) clf.score(X_test,y_test) clf.predict_proba(X_test)#第一列为等于0的概率,第二列为等于1的概率 clf.coef_ clf.intercept_
时间: 2023-11-06 15:27:29 浏览: 40
这是一段 Python 代码,使用了逻辑回归模型(LR)来对数据进行分类预测。
- 第一行代码定义了一个逻辑回归模型,其中参数 random_state = 0 表示每次随机的结果都相同,以保证模型的可重复性。
- 第二行代码使用训练集 X_train 和 y_train 对模型进行训练,并对训练集中的前两个样本进行预测。
- 第三行代码对测试集 X_test 进行预测,并返回模型的预测准确率(score)。
- 第四行代码返回测试集 X_test 中每个样本属于类别 0 或类别 1 的概率。
- 第五行代码返回模型训练得到的特征系数(coef_)。
- 第六行代码返回模型训练得到的截距(intercept_)。
需要注意的是,X_train 是一个数据框类型,因此需要使用 iloc 方法来进行切片操作。另外,这段代码并没有给出 X_train 和 y_train 的赋值代码,因此无法确定它们的具体内容和数据类型,需要根据实际情况进行设置。
相关问题
def return_value(): import pandas as pd data = pd.read_excel('2.xlsx') x_train=data.iloc[:20,1:4] y_train=data.iloc[:20,4] x_test=data.iloc[20:,1:4] from sklearn.linear_model import LogisticRegression clf = LR() clf.fit(x_train,y_train) R=clf.predict(x_test) return R解释每个步骤
这段代码是一个 Python 函数,函数名为 `return_value`。下面逐行解释每个步骤的含义:
```
import pandas as pd
```
导入 Pandas 库,用于数据处理和分析。
```
data = pd.read_excel('2.xlsx')
```
使用 Pandas 的 `read_excel` 函数读取名为 `2.xlsx` 的 Excel 文件,并将其存储在 `data` 变量中。
```
x_train=data.iloc[:20,1:4]
y_train=data.iloc[:20,4]
x_test=data.iloc[20:,1:4]
```
使用 Pandas 的 `iloc` 函数,将 `data` 数据集中的前 20 行(不含第 20 行)的第 1 列至第 3 列的数据提取出来,赋值给 `x_train`。将 `data` 数据集中的前 20 行(不含第 20 行)的第 5 列数据提取出来,赋值给 `y_train`。将 `data` 数据集中的第 21 行(含第 21 行)至最后一行的第 1 列至第 3 列的数据提取出来,赋值给 `x_test`。
```
from sklearn.linear_model import LogisticRegression
clf = LR()
clf.fit(x_train,y_train)
R=clf.predict(x_test)
```
导入 Scikit-learn 库中的 `LogisticRegression` 模块,实例化一个名为 `clf` 的逻辑回归分类器,并使用 `fit` 方法将 `x_train` 和 `y_train` 作为参数输入到分类器中进行训练。然后使用 `predict` 方法将 `x_test` 作为参数输入到分类器中进行预测,将结果存储在 `R` 变量中。
```
return R
```
将预测结果 `R` 作为函数的返回值。
def return_values(): import pandas as pd import numpy as np from sklearn.neural_network import MLPRegressor as MP data = pd.read_excel('4.xlsx') x_train=data.iloc[:,1:4] y_train=data.iloc[:,4:6] clf = MP(solver='lbfgs', alpha=1e-5,hidden_layer_sizes=8, random_state=1) clf.fit(x_train, y_train); a=np.array([[73.39,3.9635,0.9880],[75.55,4.0975,1.0268]]) Y=clf.predict(a) return Y 解释代码
这段代码主要是进行神经网络回归预测,以下是代码的详细解释:
1. 导入需要的库:
```python
import pandas as pd
import numpy as np
from sklearn.neural_network import MLPRegressor as MP
```
2. 读取Excel文件数据:
```python
data = pd.read_excel('4.xlsx')
```
3. 提取训练数据集的特征和标签:
```python
x_train=data.iloc[:,1:4] #提取第2-4列作为特征
y_train=data.iloc[:,4:6] #提取第5-6列作为标签
```
4. 初始化神经网络模型:
```python
clf = MP(solver='lbfgs', alpha=1e-5,hidden_layer_sizes=8, random_state=1)
```
其中,`solver`是优化器,`alpha`是L2正则化系数,`hidden_layer_sizes`是隐藏层神经元个数,`random_state`是随机种子,用于保证每次运行结果一致。
5. 训练神经网络模型:
```python
clf.fit(x_train, y_train)
```
6. 预测新数据:
```python
a=np.array([[73.39,3.9635,0.9880],[75.55,4.0975,1.0268]])
Y=clf.predict(a)
```
其中,`a`是待预测的新数据,`Y`是预测结果。
7. 返回预测结果:
```python
return Y
```
相关推荐
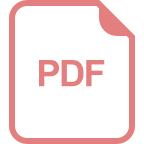













