inference in Bayes
时间: 2023-10-14 11:07:23 浏览: 39
In Bayesian inference, we start with a prior probability distribution that represents our beliefs about the probability of different events or parameters before we observe any data. We then update this prior distribution with the observed data using Bayes' theorem to obtain a posterior distribution that reflects our updated beliefs about the probability of events or parameters given the data.
The posterior distribution is obtained by multiplying the prior distribution by the likelihood of the observed data given the events or parameters, and then normalizing to obtain a probability distribution. This allows us to make probabilistic inferences about the events or parameters, such as estimating their means or variances, or making predictions about future outcomes.
Bayesian inference is a powerful framework for making inferences in a wide range of applications, including machine learning, statistics, and decision making. It allows us to incorporate prior knowledge and uncertainty into our models, and update our beliefs as new data becomes available.
相关推荐
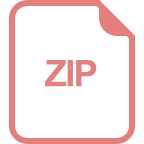
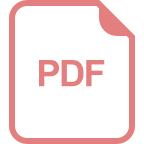
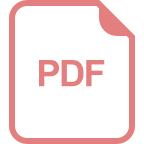














