Accelerated Development: Open Source Libraries for Monte Carlo Simulation in MATLAB
发布时间: 2024-09-15 10:21:05 阅读量: 23 订阅数: 23 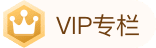
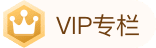
# 1. Fundamentals of Monte Carlo Simulation**
Monte Carlo simulation is a numerical method based on probability and random sampling to solve problems involving complex systems and stochastic processes. Its core idea is to estimate the expected value or probability distribution of a target quantity by generating a large number of random samples and analyzing their statistical characteristics.
The advantages of Monte Carlo simulation include:
- **Applicability to complex systems:** It can handle systems with non-linearity, high dimensionality, or uncertainty, which can be challenging for traditional methods.
- **High computational efficiency:** For certain problems, Monte Carlo simulation can be more efficient than deterministic methods, especially when dealing with large sample sizes.
- **Probability distribution estimation:** It provides the probability distribution of the target quantity, not just a single estimate value, which is crucial for risk analysis and uncertainty quantification.
# 2. Monte Carlo Simulation Libraries in MATLAB
### 2.1 Metropolis-Hastings Algorithm Library
**2.1.1 Installation and Usage**
The Metropolis-Hastings algorithm library in MATLAB can be installed via the Statistics and Machine Learning Toolbox. After installation, the library can be loaded using the following command:
```
>> load mhsampler
```
To use the Metropolis-Hastings algorithm, the following parameters need to be specified:
***Target distribution:** The distribution to be sampled.
***Proposal distribution:** The distribution used to generate new candidate samples.
***Initial state:** The initial state of the algorithm.
***Number of iterations:** The number of iterations the algorithm will run.
**2.1.2 Principles and Implementation of the Algorithm**
The Metropolis-Hastings algorithm is a Markov Chain Monte Carlo (MCMC) method used to generate samples from the target distribution. The algorithm proceeds as follows:
1. **Initialization:** Start from the initial state.
2. **Generate candidate samples:** Use the proposal distribution to generate a new candidate sample.
3. **Compute acceptance probability:** Calculate the probability that the candidate sample will be accepted.
4. **Accept or reject:** If the acceptance probability is greater than a random number, accept the candidate sample; otherwise, reject it.
5. **Update state:** If the candidate sample is accepted, update the algorithm's state.
6. **Repeat:** Repeat steps 2-5 until the desired number of iterations is reached.
### 2.2 Importance Sampling Algorithm Library
**2.2.1 Installation and Usage**
The importance sampling algorithm library in MATLAB can be installed via the Statistics and Machine Learning Toolbox. After installation, the library can be loaded using the following command:
```
>> load importance
```
To use the importance sampling algorithm, the following parameters need to be specified:
***Target distribution:** The distribution to be sampled.
***Importance distribution:** The distribution used to generate samples.
***Weight function:** The function used to calculate sample weights.
***Number of samples:** The number of samples to be generated.
**2.2.2 Principles and Implementation of the Algorithm**
The importance sampling algorithm is a Monte Carlo algorithm used to generate weighted samples from the target distribution. The algorithm proceeds as follows:
1. **Initialization:** Generate samples from the importance distribution.
2. **Compute weights:** Calculate the weight for each sample.
3. **Resampling:** Resample the samples based on their weights.
4. **Generate weighted samples:** Use the resampled samples to generate weighted samples.
### 2.3 Markov Chain Monte Carlo Algorithm Library
**2.3.1 Installation and Usage**
The Markov Chain Monte Carlo (MCMC) algorithm library in MATLAB can be installed via the Statistics and Machine Learning Toolbox. After installation, the library can be loaded using the following command:
```
>> load mcmc
```
To use the MCMC algorithm, the following parameters need to be specified:
***Target distribution:** The distribution to be sampled.
***Transition kernel:** The transition probability used to generate new candidate samples.
***Initial state:** The initial state of the algorithm.
***Number of iterations:** The number of iterations the algorithm will run.
**2.3.2 Principles and Implementation of the Algorithm**
The MCMC algorithm is a Monte Carlo algorithm used to generate samples from the target distribution. The algorithm proceeds as follows:
1. **Initialization:** Start from the initial state.
2. **Generate candidate samples:** Use the transition kernel to generate a new candidate sample.
3. **Compute acceptance probability:** Calculate the probability that the candidate sample will be accepted.
4. **Accept or reject:** If the acceptance probability is greater than a random number, accept the candidate sample; otherwise, reject it.
5. **Update state:** If the candidate sample is accepted, update the algorithm's state.
6. **Repeat:** Repeat steps 2-5 until the desired number of iterations is reached.
# 3. Practical Applications of Monte Carlo Simulation in MATLAB
### 3.1 Random Number Generation and Distribution Fitting
#### 3.1.1 Common Random Number Generators
MATLAB provides various random number generators to produce random numbers following different distributions. The most commonly used random number genera
0
0
相关推荐
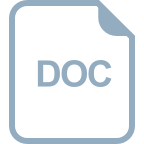
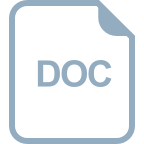
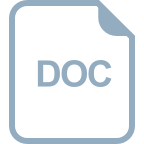
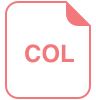
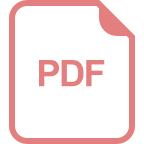
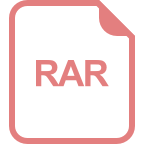
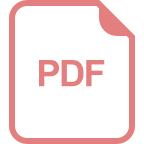
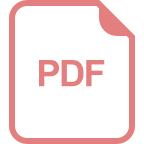
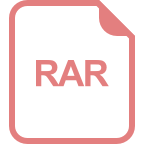