Mastering Monte Carlo Simulation: A Financial Application Guide in MATLAB
发布时间: 2024-09-15 09:57:43 阅读量: 38 订阅数: 28 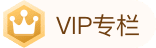
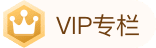
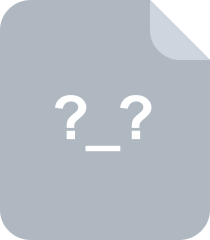
Mastering JavaScript A Complete Programming Guide Including jQuery...
# Mastering Monte Carlo Simulation: A Financial Application Guide in MATLAB
## 1. Foundations of Monte Carlo Simulation
### 1.1 Overview of Monte Carlo Simulation
Monte Carlo simulation is a probabilistic numerical technique used to solve complex problems involving random variables. It approximates solutions by generating a large number of random samples and calculating the results for each sample.
### 1.2 Random Number Generation and Probability Distributions
Random number generation is the foundation of Monte Carlo simulation. MATLAB offers a range of functions to generate random numbers from various distributions, such as normal, uniform, and exponential distributions. These distributions can be used to model real-world random events.
## 2. Monte Carlo Simulation in MATLAB
### 2.1 Random Number Generation in MATLAB
MATLAB provides a suite of functions for generating random numbers, including:
```
rand: Generates uniformly distributed random numbers.
randn: Generates normally distributed random numbers.
randperm: Generates a random permutation.
```
### 2.2 Common Probability Distributions and MATLAB Functions
MATLAB also offers various probability distribution functions for generating random numbers from specific distributions. Some common distributions and their MATLAB functions include:
| Distribution | MATLAB Function |
|---|---|
| Normal Distribution | normrnd |
| Log-Normal Distribution | lognrnd |
| Exponential Distribution | exprnd |
| Poisson Distribution | poissrnd |
### 2.3 MATLAB Implementation of Monte Carlo Simulation
Implementing Monte Carlo simulation in MATLAB involves the following steps:
1. Define the probability distribution of the random variables.
2. Generate random samples.
3. Calculate the objective function.
4. Repeat steps 2 and 3 until a sufficient number of samples is obtained.
5. Analyze the results and compute statistics.
The following code snippet demonstrates an example implementation of Monte Carlo simulation in MATLAB:
```
% Define normally distributed random variable
mu = 0; % Mean
sigma = 1; % Standard deviation
% Generate random samples
n = 10000; % Number of samples
X = normrnd(mu, sigma, n, 1);
% Calculate the objective function
Y = X.^2;
% Analyze the results
mean_Y = mean(Y); % Sample mean
std_Y = std(Y); % Sample standard deviation
```
**Code Logic Analysis:**
* The `normrnd` function generates normally distributed random samples, where `mu` and `sigma` parameters specify the mean and standard deviation.
* The `n` variable specifies the number of samples.
* `X.^2` computes the square of the random samples, serving as the objective function.
* The `mean` and `std` functions calculate the sample mean and standard deviation.
## 3. Applications of Monte Carlo Simulation in Finance
### 3.1 Challenges in Financial Modeling
Financial modeling is a critical task in the financial industry, involving the prediction and evaluation of the future performance of financial assets. However, financial markets are inherently highly uncertain, posing significant challenges for financial modeling.
***Uncertainty:** Financial markets are influenced by various factors, including economic conditions, political events, and natural disasters. The unpredictability of these factors makes accurate forecasting of future performance difficult.
***Complexity:** Financial instruments and markets are becoming increasingly complex, making modeling and analysis more challenging. For instance, derivatives and structured products have nonlinear and interrelated features, increasing the complexity of modeling.
***Computational Intensity:** Financial models often require extensive computations, especially when simulating a large number of scenarios. This can result in long computation times and high resource consumption.
### 3.2 Advantages of Monte Carlo Simulation in Finance
Monte Carlo simulation tackles the challenges in financial modeling by simulating a large number of random scenarios. It offers the following advantages:
***Handling Uncertainty:** Monte Carlo simulation can capture the randomness and uncertainty of financial markets by generating a large number of random samples. This allows it to evaluate the potential performance of assets under different scenarios.
***Handling Complexity:** Monte Carlo simulation can handle the nonlinear relationships of complex financial instruments and markets. It allows modelers to simulate interactions between different variables and consider tail risks.
***Parallelization:** Monte Carlo simulation can be parallelized, significantly reducing computation time. By running simulations simultaneously on multiple processors, results can be obtained more quickly.
### 3.3 Applications of Monte Carlo Simulation in Finance
Monte Carlo simulation has a wide range of applications in finance, including:
***Risk Management:** Assessing the risk of a portfolio, including Value at Risk (VaR) and Expected Shortfall (ES).
***Portfolio Optimization:** Optimizing the asset allocation of a portfolio to maximize returns and minimize risk.
***Pricing Financial Derivatives:** Pricing complex financial derivatives, such as options, swaps, and credit default swaps (CDS).
***Credit Risk Assessment:** Assessing the probability of default and the amount of loss for borrowers.
***Market Risk Analysis:** Simulating the impact of market fluctuations on financial assets to evaluate potential losses.
#### Code Example: Monte Carlo Simulation for Pricing European Call Options
```matlab
% Parameters
S0 = 100; % Current price of the underlying asset
K = 105; % Strike price
r = 0.05; % Risk-free interest rate
sigma = 0.2; % Volatility
T = 1; % Time to maturity
% Monte Carlo Simulation
N = 10000; % Number of simulations
dt = T / N; % Time step
% Simulate random paths
S = zeros(N, N);
for i = 1:N
for j = 1:N
dW = sqrt(dt) * randn;
S(i, j) = S0 * exp((r - sigma^2 / 2) * dt + sigma * dW);
end
end
% Calculate option price
C = max(S(:, end) - K, 0);
option_price = exp(-r * T) * mean(C);
% Output result
fprintf('European call option price: %.4f\n', option_price);
```
#### Code Logic Analysis
This code simulates the random paths of a European call option and calculates the option price.
***Parameters:** The code defines the option parameters, including the current price of the underlying asset, strike price, risk-free interest rate, volatility, and time to maturity.
***Monte Carlo Simulation:** The code uses normally distributed random numbers to simulate the random paths of the underlying asset.
***Calculate Option Price:** The code computes the payoff of the option at maturity, then discounts it back to present value to obtain the option price.
## 4. Practical Tips for Monte Carlo Simulation
### 4.1 Variance Reduction Techniques
In Monte Carlo simulation, variance is a key factor affecting the accuracy and efficiency of the simulation. Higher variance leads to greater fluctuations in simulation results, requiring more simulation runs to obtain reliable results. Therefore, reducing variance is crucial for improving simulation efficiency.
#### Antithetic Sampling
Antithetic sampling is a technique that reduces variance by transforming the distribution of a random variable. The basic principle is to convert the original distribution into a uniform distribution, generate random numbers from the uniform distribution, and then map them back to the original distribution using the inverse function.
```
% Define the probability density function of the original distribution
pdf = @(x) exp(-x.^2 / 2) / sqrt(2 * pi);
% Generate uniform distribution random numbers
u = rand(1, N);
% Apply the inverse function mapping to the original distribution
x = sqrt(-2 * log(u)) * sign(u - 0.5);
```
#### Control Variates Method
The control variates method is a technique that reduces variance by introducing an auxiliary variable that is highly correlated with the target variable. The selection of the auxiliary variable should meet the following conditions:
* It should have a high correlation with the target variable.
* It should have a small variance.
```
% Define the distribution of the target variable and the auxiliary variable
mu_x = 0;
sigma_x = 1;
mu_y = 1;
sigma_y = 0.5;
rho = 0.8;
% Generate random numbers for the target variable and the auxiliary variable
x = normrnd(mu_x, sigma_x, 1, N);
y = normrnd(mu_y, sigma_y, 1, N);
% Calculate the control variates method estimate
E_x_est = mean(x - rho * (x - mu_x) / (sigma_x * sigma_y) * (y - mu_y));
```
### 4.2 Parallelization Methods for Increased Efficiency
Parallelization is an effective method to improve the efficiency of Monte Carlo simulation, especially for large-scale simulation tasks. MATLAB provides the Parallel Computing Toolbox, which can easily parallelize simulation tasks to multi-core processors or compute clusters.
#### Parallel for Loops
Parallel for loops allow for the parallelization of for loops across multiple worker processes. Each worker process is responsible for executing a portion of the loop, significantly speeding up computation.
```
% Define a parallel for loop
parfor i = 1:N
% Execute simulation task
result(i) = simulate(params);
end
```
#### Parallel Pool
Parallel pool is a more advanced parallelization method that allows creating a set of worker processes and using them to execute tasks. Parallel pools offer more control and flexibility but are more complex to set up and manage.
```
% Create a parallel pool
pool = parpool(num_workers);
% Assign tasks to the parallel pool
spmd
% Execute simulation task
result = simulate(params);
end
% Stop the parallel pool
delete(pool);
```
### 4.3 Validation and Verification of Monte Carlo Simulations
Validation and verification are key steps to ensure the accuracy and reliability of Monte Carlo simulation results. Validation refers to checking whether the simulation implementation is correct, while verification refers to checking whether the simulation results match expectations.
#### Validation
Validation involves checking whether the simulation implementation matches its expected behavior. This can be done through the following methods:
* Check if the random number generator produces numbers that conform to the expected distribution.
* Check if the simulation function correctly computes the target variable.
* Check if the parallelization method correctly parallelizes the simulation task.
#### Verification
Verification involves comparing simulation results with known results or results from other simulation methods. This can be done through the following methods:
* Compare with analytical solutions or other numerical methods.
* Rerun the simulation using different random number seeds and check for consistency.
* Use different simulation parameters and check if the results match expectations.
## 5. Expanding Applications of Monte Carlo Simulation
The applications of Monte Carlo simulation in finance extend far beyond the cases mentioned above; it is also widely used in risk management, portfolio optimization, and pricing financial derivatives.
### 5.1 Applications of Monte Carlo Simulation in Risk Management
Monte Carlo simulation plays a critical role in risk management. By simulating various possible scenarios, risk managers can assess potential risk exposures and take measures to mitigate these risks.
For example, Monte Carlo simulation can be used for:
- **Credit Risk Assessment:** Simulate the likelihood of borrower default and estimate the resulting losses.
- **Market Risk Assessment:** Simulate asset price fluctuations and assess the resulting portfolio losses.
- **Operational Risk Assessment:** Simulate the possibility of operational failures or fraud and assess the resulting financial impact.
### 5.2 Applications of Monte Carlo Simulation in Portfolio Optimization
Monte Carlo simulation also plays a significant role in portfolio optimization. By simulating various portfolio scenarios, portfolio managers can optimize the risk and return of portfolios.
For example, Monte Carlo simulation can be used for:
- **Portfolio Construction:** Simulate the potential returns and risks of different asset allocations and select the optimal portfolio.
- **Risk Management:** Simulate the performance of a portfolio under different market conditions and determine the best risk management strategy.
- **Portfolio Rebalancing:** Simulate the performance of a portfolio at different time points and determine the best rebalancing strategy.
### 5.3 Applications of Monte Carlo Simulation in Pricing Financial Derivatives
Monte Carlo simulation is also crucial in pricing financial derivatives. By simulating the price paths of the underlying assets of derivatives, pricing models can estimate the fair value of the derivatives.
For example, Monte Carlo simulation can be used for:
- **Option Pricing:** Simulate the price paths of the underlying asset and estimate the fair value of options.
- **Swap Pricing:** Simulate interest rate paths and estimate the fair value of swaps.
- **Credit Derivatives Pricing:** Simulate the likelihood of borrower default and estimate the fair value of credit derivatives.
0
0
相关推荐







