10 Practical Tips for Monte Carlo Simulation in MATLAB
发布时间: 2024-09-15 09:53:31 阅读量: 24 订阅数: 21 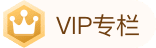
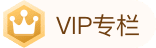
# Decoding 10 Practical Tips for Monte Carlo Simulation in MATLAB
## 1. Introduction to Monte Carlo Simulation
Monte Carlo simulation is a numerical technique based on probability and randomness used to solve complex problems. It approximates the expected value or integral of a target function by generating a large number of random samples and conducting statistical analysis on these samples. The advantage of Monte Carlo simulation lies in its ability to handle high-dimensional, nonlinear problems that traditional methods find difficult to solve, without the need for simplification or linearization of the problem.
In MATLAB, Monte Carlo simulation can be implemented using various built-in functions such as `rand` and `randn` for generating random numbers; `integral` for computing integrals; and `montecarlo` for performing Monte Carlo sampling.
## 2. Implementation of Monte Carlo Simulation in MATLAB
### 2.1 Random Number Generation in MATLAB
MATLAB offers several methods for generating random numbers, including:
- `rand`: Generates uniformly distributed random numbers between 0 and 1.
- `randn`: Generates normally distributed random numbers with a mean of 0 and a standard deviation of 1.
- `randsample`: Randomly samples a specified number of elements from a given range.
```matlab
% Generates 10 uniformly distributed random numbers between 0 and 1
rand_numbers = rand(1, 10);
% Generates 10 normally distributed random numbers with mean 0 and standard deviation 1
normal_numbers = randn(1, 10);
% Randomly samples 5 numbers from the range 1 to 100
sample_numbers = randsample(1:100, 5);
```
### 2.2 Implementation of Monte Carlo Integration
Monte Carlo integration is a method that estimates the integral value by random sampling. In MATLAB, the `integral` function can be used to perform Monte Carlo integration:
```matlab
% Defines the integrand function
f = @(x) sin(x);
% The interval of integration
a = 0;
b = pi;
% Number of samples
N = 10000;
% Randomly generates sample points
x = a + (b - a) * rand(N, 1);
% Computes the integral value
integral_value = (b - a) / N * sum(f(x));
```
### 2.3 Implementation of Monte Carlo Sampling
Monte Carlo sampling is a method that generates samples of random variables through random sampling. In MATLAB, the `mvnrnd` function can be used to generate samples from a multivariate normal distribution:
```matlab
% Defines the mean vector
mu = [0, 0];
% Defines the covariance matrix
Sigma = [1, 0; 0, 1];
% Number of samples
N = 1000;
% Generates random samples
samples = mvnrnd(mu, Sigma, N);
```
## 3. Practical Tips for Monte Carlo Simulation
### 3.1 Tips for Improving Sampling Efficiency
#### 3.1.1 Importance Sampling
Importance sampling is a technique that improves sampling efficiency by modifying the sampling distribution. It achieves this by concentrating the sampling distribution around the peak regions of the target distribution.
```matlab
% Original sampling distribution
f = @(x) exp(-x.^2);
x = randn(10000, 1);
% Importance sampling distribution
g = @(x) exp(-(x-3).^2);
y = randn(10000, 1) + 3;
% Sample result comparison
figure;
histogram(x, 50, 'Normalization', 'pdf');
hold on;
histogram(y, 50, 'Normalization', 'pdf');
legend('Original Sampling', 'Importance Sampling');
```
#### Logical Analysis:
* `f` defines the original sampling distribution, which is a normal distribution.
* `g` defines the importance sampling distribution, which is a normal distribution centered at 3.
* `x` and `y` sample from the original and importance distributions, respectively.
* The histogram displays the sampling results. It can be seen that the importance sampling distribution is concentrated in the peak regions of the target distribution, improving sampling efficiency.
#### 3.1.2 Stratified Sampling
Stratified sampling is a technique that divides the sampling space into multiple subspaces and then samples within each subspace. It can improve sampling efficiency, especially when the target distribution has multiple peaks.
```matlab
% Defines the sampling space
intervals = [0, 1; 1, 2; 2, 3];
% Samples within each subspace
num_samples = 1000;
x = zeros(num_samples, 3);
for i = 1:3
x(:, i) = rand(num_samples, 1) * (intervals(i, 2) - intervals(i, 1)) + intervals(i, 1);
end
% Visualizes the sampling results
figure;
scatter3(x(:, 1), x(:, 2), x(:, 3));
xlabel('Subspace 1');
ylabel('Subspace 2');
zlabel('Subspace 3');
```
#### Logical Analysis:
* `intervals` defines three subspaces of the sampling space.
* `x` is the sampled data from each subspace.
* The scatter plot visualizes the sampling results, showing that the sampling distribution uniformly covers the entire sampling space.
### 3.2 Tips for Reducing Variance
#### 3.2.1 Control Variable Method
The control variable method is a technique that reduces variance by introducing a control variable. The control variable is correlated with the target variable, but its distribution is known.
```matlab
% Target variable
f = @(x) exp(-x.^2);
% Control variable
g = @(x) x;
% Sampling
num_samples = 10000;
x = randn(num_samples, 1);
y = randn(num_samples, 1);
% Calculate expected values
E_f = mean(f(x));
E_g = mean(g(x));
cov_fg = cov(f(x), g(x));
% Calculate expected value using control variable method
E_f_cv = E_f - cov_fg(1, 2) / cov_fg(2, 2) * (E_g - E_f);
```
#### Logical Analysis:
* `f` and `g` define the target and control variables, respectively.
* `x` and `y` are sampled data from the target and control variables.
* `E_f` and `E_g` are the expected values of the target and control variables.
* `cov_fg` is the covariance between the target and control variables.
* `E_f_cv` is the expected value of the target variable calculated using the control variable method.
#### 3.2.2 Antithetic Variable Method
The antithetic variable method is a technique that reduces variance by reversing the sampling from the target distribution to a known distribution. It can be used for computing conditional expectations.
```matlab
% Target distribution
f = @(x) exp(-x.^2);
% Known distribution
g = @(x) normcdf(x);
% Sampling
num_samples = 10000;
u = rand(num_samples, 1);
% Calculate conditional expectation
E_f_given_u = mean(f(norminv(u)));
```
#### Logical Analysis:
* `f` and `g` define the target and known distributions, respectively.
* `u` is the sampled data from the known distribution.
* `E_f_given_u` is the conditional expected value of the target distribution given the known distribution.
## 4. Applications of Monte Carlo Simulation in MATLAB
### 4.1 Financial Modeling
Monte Carlo simulation is widely used in financial modeling for simulating the price trends of financial assets and for risk assessment.
#### Stock Price Simulation
```matlab
% Defines parameters for the stock price random walk model
mu = 0.05; % Average return rate
sigma = 0.2; % Volatility
T = 1; % Simulation time length (years)
N = 1000; % Number of simulations
% Generates stock price random walk paths
S0 = 100; % Initial stock price
S = zeros(N, T+1);
S(:, 1) = S0;
for i = 2:T+1
S(:, i) = S(:, i-1) .* exp((mu - 0.5*sigma^2)*dt + sigma*sqrt(dt)*randn(N, 1));
end
% Plots the stock price paths
figure;
plot(S);
xlabel('Time (years)');
ylabel('Stock Price');
title('Stock Price Random Walk Simulation');
```
#### Logical Analysis:
* The code simulates the stock price random walk model, where `mu` is the average return rate, `sigma` is the volatility, `T` is the simulation time length, and `N` is the number of simulations.
* `S0` is the initial stock price, and `S` stores the stock prices for all simulation paths.
* For each time step `dt`, the stock price is updated using the formula: `S(t+1) = S(t) * exp((mu - 0.5*sigma^2)*dt + sigma*sqrt(dt)*randn)`, where `randn` generates normally distributed random numbers.
* Finally, the code plots the stock price paths.
### 4.2 Risk Assessment
Monte Carlo simulation is also used for risk assessment, such as calculating the value-at-risk (VaR) of a portfolio.
#### VaR Calculation
```matlab
% Defines the return distribution of a portfolio
mu = [0.05, 0.03, 0.02]; % Average returns of assets
sigma = [0.1, 0.05, 0.03]; % Volatilities of assets
corr = [1, 0.5, 0.3; % Correlation matrix between assets
0.5, 1, 0.2;
0.3, 0.2, 1];
% Defines the confidence level
alpha = 0.05;
% Calculates VaR using Monte Carlo simulation
N = 10000; % Number of simulations
VaR = zeros(N, 1);
for i = 1:N
% Generates a random vector of asset returns
r = mvnrnd(mu, corr, N);
% Calculates the portfolio return
portfolio_return = r * weights;
% Calculates the portfolio's VaR
VaR(i) = quantile(portfolio_return, alpha);
end
% Outputs the VaR value
disp(['The portfolio's VaR is: ' num2str(mean(VaR))]);
```
#### Logical Analysis:
* The code calculates the portfolio's VaR, where `mu` is the average returns of assets, `sigma` is the volatilities of assets, `corr` is the correlation matrix between assets, and `alpha` is the confidence level.
* `N` is the number of simulations, and `VaR` stores the VaR values for all simulation paths.
* For each simulation path, the code generates a random vector of asset returns, calculates the portfolio's return, and computes the VaR.
* Finally, the code outputs the average VaR value of the portfolio.
### 4.3 Physical Modeling
Monte Carlo simulation is also used in physical modeling, such as simulating particle motion or solving partial differential equations.
#### Particle Motion Simulation
```matlab
% Defines the physical parameters for particle motion
mass = 1; % Particle mass
velocity = [1, 2, 3]; % Particle initial velocity
time = 10; % Simulation time length
% Defines the gravitational acceleration
g = 9.81;
% Simulates particle motion using Monte Carlo simulation
N = 1000; % Number of simulations
positions = zeros(N, 3, time+1);
for i = 1:N
% Initializes particle position
positions(i, :, 1) = [0, 0, 0];
% Simulates particle motion
for t = 2:time+1
% Calculates particle acceleration
a = [-g, 0, 0];
% Updates particle velocity and position
velocity = velocity + a * dt;
positions(i, :, t) = positions(i, :, t-1) + velocity * dt;
end
end
% Plots the particle motion trajectory
figure;
plot3(positions(:, 1, :), positions(:, 2, :), positions(:, 3, :));
xlabel('x');
ylabel('y');
zlabel('z');
title('Particle Motion Simulation');
```
#### Logical Analysis:
* The code simulates the motion of particles, where `mass` is the particle mass, `velocity` is the particle initial velocity, and `time` is the simulation time length.
* `g` is the gravitational acceleration, `N` is the number of simulations, and `positions` stores the particle positions for all simulation paths.
* For each simulation path, the code initializes particle positions, calculates particle acceleration, updates particle velocity and position, and stores the particle positions.
* Finally, the code plots the particle motion trajectory.
## 5. Limitations and Considerations of Monte Carlo Simulation
Monte Carlo simulation is a powerful tool, but it does have limitations and considerations:
### Limitations
***High computational cost:** Monte Carlo simulation requires a large number of samples, which can lead to high computational costs, especially when dealing with complex models.
***Limited accuracy:** The accuracy of Monte Carlo simulation is influenced by the number of samples. Increasing the number of samples can improve accuracy, but it also increases computational costs.
***Sensitive to inputs:** Monte Carlo simulation is sensitive to input distributions and model parameters. If the inputs are inaccurate or the model is inappropriate, the simulation results may be inaccurate.
### Considerations
***Choosing the right random number generator:** MATLAB offers various random number generators, and choosing the appropriate one is crucial for ensuring the accuracy of the simulation.
***Optimizing sampling strategies:** Techniques such as importance sampling and stratified sampling can improve sampling efficiency and thus reduce computational costs.
***Controlling variance:** Techniques such as the control variable method and the antithetic variable method can reduce variance, thus improving the accuracy of the simulation.
***Verifying simulation results:** It is crucial to verify the accuracy of Monte Carlo simulation results before using them for decision-making. This can be done using analytical methods or other simulation techniques.
***Being cautious in interpreting results:** Monte Carlo simulation results are probabilistic, so caution should be taken when interpreting results. The limitations of the simulation should be considered, and overinterpretation of results should be avoided.
0
0
相关推荐
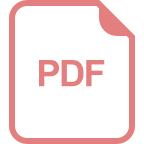
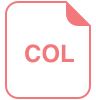
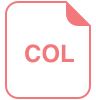
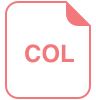
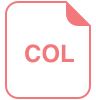
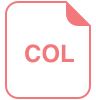
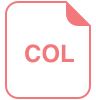
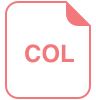