Parameter Estimation and System Identification in MATLAB Signal Processing
发布时间: 2024-09-14 11:24:47 阅读量: 34 订阅数: 37 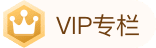
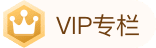
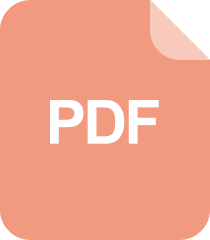
Fundamentals of statistical signal processing-estimation theory

# 1. MATLAB Signal Processing Algorithms Tutorial
In the world of digital signal processing, MATLAB serves as a powerful computational and visualization tool, offering engineers and researchers a platform for algorithm development, data analysis, data visualization, and simulation design. This chapter aims to provide a basic introduction to MATLAB in signal processing for both beginners and experienced engineers. We will start with the representation and classification of signals, then move on to learn about signal transformations, and finally introduce some fundamental signal processing techniques. The content of this chapter will not only help readers understand the basic concepts of signal processing but also enable them to grasp the preliminary application of MATLAB in this field.
## 1.1 Basic Concepts of Signals
A signal is a carrier of information and can be either continuous or discrete. In MATLAB, our focus is primarily on digital signals, which are obtained through a process of sampling and quantization. Signals can be deterministic, such as a sine wave, or random, like white noise.
## 1.2 Signal Representation in MATLAB
In MATLAB, signals can be represented using vectors or matrices, where each element corresponds to a sampling point. MATLAB provides a series of functions for signal creation, editing, and manipulation, such as the `sin()` function for creating sine signals and the `rand()` function for generating random noise signals.
```matlab
t = 0:0.001:1; % Create a time vector
f = 5; % Set the frequency to 5Hz
sineSignal = sin(2*pi*f*t); % Create a sine signal
```
## 1.3 Common Signal Processing Techniques
The goal of signal processing techniques is to extract useful information from signals, including filtering, Fourier transforms, and wavelet transforms. MATLAB provides a suite of functions through the Signal Processing Toolbox to perform these operations.
```matlab
% Use Fourier transform for spectral analysis
spectrum = fft(sineSignal);
```
By the end of this chapter, readers should be proficient in using MATLAB for basic signal processing operations and lay a solid foundation for further advanced studies in parameter estimation and system identification. In subsequent chapters, we will gradually explore these advanced topics and focus on how to leverage MATLAB to accomplish complex signal processing tasks.
# 2. Chapter 2: Theory and Methods of Parameter Estimation
## 2.1 Basic Concepts of Parameter Estimation
Parameter estimation is a core concept in statistics, involving the inference of population parameters using observed data under certain assumptions of probability distributions. The accuracy of parameter estimation directly affects the performance of a model and is a crucial part of data analysis.
### 2.1.1 Definition of Parameter Estimation
Parameter estimation can generally be divided into two types: point estimation and interval estimation. Point estimation uses a statistic (e.g., sample mean) to estimate a population parameter (e.g., population mean). Interval estimation provides a range within which the unknown population parameter is expected to fall with a certain probability.
### 2.1.2 Objectives and Significance of Parameter Estimation
The goal of parameter estimation is to infer the characteristics of the whole population as accurately as possible through limited sample data, which has broad implications in practical applications. For instance, in the field of engineering, parameter estimation can help us estimate the reliability of systems; in finance, it can be used to assess risks; in biomedicine, it can be applied to disease prediction and the evaluation of treatment outcomes.
## 2.2 Main Methods of Parameter Estimation
There are numerous methods of parameter estimation, each with its own characteristics and areas of application. This chapter will introduce three common methods of parameter estimation: least squares, maximum likelihood estimation, and Bayesian estimation.
### 2.2.1 Least Squares Method
The least squares method is a mathematical optimization technique that seeks the best functional fit to data by minimizing the sum of squared errors. In the least squares method, the objective is to find the estimated values of parameters that minimize the sum of squared differences between observed data and model predictions.
#### Specific Implementation Steps:
1. Define the objective function, typically the sum of squared errors.
2. Take partial derivatives of the objective function with respect to unknown parameters and set them equal to zero.
3. Solve the equation system to obtain the estimated values of the parameters.
#### Example Code:
```matlab
% Assume there is a set of data points (x_data, y_data) and a model function model_func
% model_func's parameters are what we need to estimate
x_data = [...]; % Independent variable data
y_data = [...]; % Dependent variable data
model_func = @(p, x) p(1)*exp(p(2)*x); % Model function, p is the parameter vector
% Use MATLAB's fminsearch function for minimization calculation
initial_params = [1, -1]; % Initial guess values for parameters
options = optimset('TolFun', 1e-6, 'MaxFunEvals', 10000, 'MaxIter', 10000); % Set optimization parameters
best_params = fminsearch(@(p) sum((y_data - model_func(p, x_data)).^2), initial_params, options);
% Output the estimated parameter values
disp(best_params);
```
### 2.2.2 Maximum Likelihood Estimation
Maximum likelihood estimation is a parameter estimation method based on probability models. Its core idea is to choose parameter values that maximize the probability of observing the given data.
#### Implementation Steps:
1. Define the likelihood function, which is the probability of observing the data given the parameters.
2. Take the logarithm of the likelihood function to obtain the log-likelihood function.
3. Take derivatives of the log-likelihood function with respect to the parameters and set them equal to zero.
4. Solve the equation to obtain the estimated values of the parameters.
#### Example Code:
```matlab
% Assume we have a set of data y_data and a probability density function of a normal distribution
n = length(y_data); % Number of data points
mu = sum(y_data)/n; % Estimate of the mean
sigma_squared = sum((y_data - mu).^2)/n; % Estimate of the variance
% Output the parameter values estimated by maximum likelihood
disp(mu);
disp(sigma_squared);
```
### 2.2.3 Bayesian Estimation
Bayesian estimation is a parameter estimation method based on Bayes' theorem. It takes into account prior knowledge of the parameters and combines observed data to calculate the posterior distribution of the parameters.
#### Implementation Steps:
1. Set the prior distribution of the parameters.
2. Calculate the posterior distribution based on observed data and prior distribution.
3. Analyze the posterior distribution to obtain the estimated values of the parameters.
#### Example Code:
```matlab
% Assume the prior distribution of the parameter theta is a beta distribution, and the observed data y follows a binomial distribution
alpha_prior = 2; % Alpha parameter of the beta distribution
beta_prior = 2; % Beta parameter of the beta distribution
y = [1, 0, 1, 1, 0, 1, 0, 0]; % Observed data
% Use MATLAB's betafit function for Bayesian estimation
theta_posterior = betafit(y + alpha_prior, length(y) + beta_prior);
% Output the estimated values of the parameters from the posterior distribution
disp(theta_posterior);
```
## 2.3 Performance Analysis of Parameter Estimation
To evaluate the quality of parameter estimation methods, consistency and efficiency analyses are typically conducted.
### 2.3.1 Consistency Analysis
Consistency analysis examines whether the estimator converges to the true parameter value as the sample size increases. If an estimator is unbiased and its variance tends to zero as the sample size increases, the estimator is said to be asymptotically consistent.
### 2.3.2 Efficiency Analysis
Efficiency analysis mainly compares the variances of different estimators. Generally, the smaller the variance, the higher the efficiency of the estimator. The Cramer-Rao inequality provides a theoretical lower bound for evaluating the efficiency of parameter estimation.
#### Example Analysis:
- In practical applications, parameter estimation can be performed with different sample sizes, and the bias and variance trends of the estimators can be analyzed as the sample size changes to evaluate the consistency and efficiency of parameter estimation.
- Comprehensive indicators such as Mean Squared Error (MSE) can also be used for evaluation, which considers the expected squared deviation of the estimated value from the true value, reflecting both the accuracy and precision of the estimation.
The theory and methods of parameter estimation are essential tools in signal processing and data analysis. The methods and analytical approaches introduced in this chapter provide a foundation for in-depth understanding and application of parameter estimation. In subsequent chapters, we will further explore how to implement these parameter estimation methods using MATLAB and deepen our understanding of the theory through practical cases.
# 3. Theory and Practice of System Identification
System identification is a key step in understanding and modeling dynamic systems, involving the estimation of system parameters from input and output data and the establishment of these parameters in mathematical models. This chapter will explore the basic framework and methods of system identification and deepen understanding through example analysis.
## 3.1 Basic Framework of System Identification
### 3.1.1 Definition and Purpose of System Identification
System identification is an interdisciplinary field involving mathematics, statistics, and computer science. It primarily studies how to use observed data to establish or improve a system's mathematical model. The purpose of system identification is to build a model that accurately describes the behavior of a system using observed data, thereby providing a basis for system analysis, control, prediction, etc.
### 3.1.2 Selection and Cla***
***mon system models include:
- **Discrete-time and continuous-time models:** Depending on time attributes, systems can be modeled as discrete or continuous.
- **Linear models and nonlinear models:** Linear models are simple and easy to analyze, while nonlinear models can more accurately describe the complex systems of the real world.
- **Black-box models, gray-box models, and white-box models:** These three types of models correspond t
0
0
相关推荐
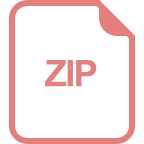
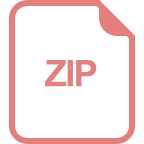
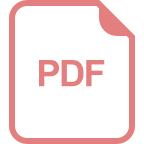
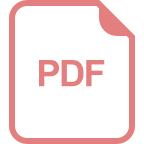
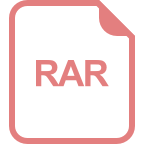
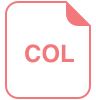
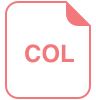
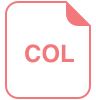