MATLAB Signal Enhancement Techniques: Strategies for Improving Signal Clarity
发布时间: 2024-09-14 10:53:15 阅读量: 8 订阅数: 17 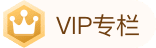
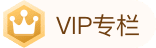
# 1. An Overview of Signal Enhancement Technology
Signal enhancement technology is indispensable in fields such as communications, radar, biomedicine, and audio. Its purpose is to extract useful information from a noisy background. Research into signal enhancement technology not only improves the intelligibility of signals but also enhances the accuracy of subsequent processing and analysis, providing more reliable signal support for various applications.
## 1.1 The Background of Signal Enhancement Needs
In practical applications, signals are often accompanied by noise, which interferes with the detection, analysis, and identification of the signal. For example, background noise affects the accuracy of speech recognition; in medical imaging, noise affects the clarity of the image. Therefore, the demand for signal enhancement is widespread across various industries.
## 1.2 The Key Points of Signal Enhancement Technology
The core of signal enhancement technology lies in distinguishing between useful signals and noise and applying appropriate processing methods to highlight signal characteristics and suppress noise. This involves complex algorithms, such as filtering technology, frequency domain processing, wavelet transforms, etc., which play their respective roles in different application scenarios.
## 1.3 The Development Trends of Signal Enhancement
With the advancement of computing power and algorithm improvements, signal enhancement technology is also evolving. Future research directions may focus on deep learning, artificial intelligence, and big data analytics, aiming for more intelligent and efficient signal processing results.
# 2. MATLAB Basics and Signal Processing Toolbox
## 2.1 Introduction to MATLAB and Its Applications in Signal Processing
### 2.1.1 The Features and Advantages of MATLAB Software
MATLAB (an abbreviation for Matrix Laboratory) is an advanced numerical computing and visualization environment that integrates computing, visualization, and programming functions, making it particularly suitable for engineering computation and algorithm development. The features of MATLAB lie in its powerful matrix computation capabilities and rich function libraries, which give it a wide application in signal processing, control design, statistical analysis, and more. The main advantages of MATLAB are reflected in the following aspects:
- **Matrix and Array Operations**: MATLAB's name contains "matrix laboratory," indicating its strong built-in support for matrix operations. From simple vector and matrix operations to complex data analysis, MATLAB can provide efficient algorithm implementations.
- **Programming Environment**: MATLAB provides an interactive programming environment that can execute commands instantly and has rich visualization tools, making code debugging and result analysis more convenient.
- **Toolboxes**: MATLAB offers a series of professional toolboxes that provide specific functions and tools for different fields. The signal processing toolbox is one of them, providing a powerful set of functions for signal analysis, filter design, etc.
### 2.1.2 A Brief Overview of Signal Processing Toolbox Functions
MATLAB's signal processing toolbox is a powerful package that provides users with a wide range of signal processing functions and applications, including signal analysis, filtering, estimation, correction, etc. The functions included in the toolbox can be summarized in the following main aspects:
- **Signal Analysis**: Provides a series of functions for signal Fourier analysis, Hilbert transform, spectral analysis, etc.
- **Filter Design and Application**: Contains functions for designing different types of filters and implementing these filters to process signals.
- **Signal Transformation**: Supports various signal transformation methods, such as wavelet transform, discrete cosine transform (DCT), and Hadamard transform.
- **Statistical Signal Processing**: Implements signal statistical analysis, such as autocorrelation, cross-correlation, and power spectral density estimation.
- **Time-Frequency Analysis**: Provides methods such as short-time Fourier transform (STFT) and wavelet time-frequency analysis.
With these functions, the signal processing toolbox provides a comprehensive set of tools for engineers and researchers to conduct experimental design, algorithm development, and system simulation.
### 2.1.3 Examples of Using the Signal Processing Toolbox
To demonstrate the practical application of the MATLAB signal processing toolbox, we can illustrate how to use the toolbox for signal filtering through a simple example.
Suppose we have a signal with noise that needs to be processed with a low-pass filter to remove high-frequency noise. Here are the steps to use MATLAB's signal processing toolbox for filtering:
1. **Define the Signal and Noise**: First, we need to define the original signal and the noise to be added.
2. **Design a Low-Pass Filter**: Design an appropriate low-pass filter based on the characteristics of the signal and the noise frequency.
3. **Apply the Filter**: Use the designed filter to process the noisy signal.
4. **Display the Results**: Compare the signal before and after filtering and analyze the filtering effect.
Code Example:
```matlab
% Define the original signal and noise
t = 0:0.001:1;
original_signal = sin(2*pi*10*t) + 0.5*randn(size(t));
noisy_signal = original_signal + 0.5*randn(size(t));
% Design a low-pass filter
Fs = 1000; % Sampling frequency
Fc = 30; % Cutoff frequency
[b, a] = butter(4, Fc/(Fs/2)); % 4th order Butterworth filter
% Apply the filter
filtered_signal = filter(b, a, noisy_signal);
% Display results
subplot(3, 1, 1);
plot(t, original_signal);
title('Original Signal');
subplot(3, 1, 2);
plot(t, noisy_signal);
title('Noisy Signal');
subplot(3, 1, 3);
plot(t, filtered_signal);
title('Filtered Signal');
```
Through the above code, we can visually see the changes in the signal before and after filtering, which helps to understand the role of the signal processing toolbox in practical applications.
## 2.2 The Theoretical Basis of Signal Enhancement in MATLAB
### 2.2.1 Basic Concepts of Signals and Noise
In signal processing, the signal represents the information we need to transmit or analyze. The signal can be either continuous-time or discrete-time. Noise generally refers to unwanted, random, or intentionally added information that affects the quality of the signal, making signal identification and extraction more difficult. Noise is usually random and can come from various sources, such as electronic equipment, transmission media, and environmental interference.
### 2.2.2 The Purpose and Methods of Signal Enhancement
The purpose of signal enhancement is to improve the quality of the signal, making it clearer during transmission or processing, thus facilitating subsequent analysis and recognition. This typically includes denoising the signal, improving signal contrast, reducing distortion, etc. Signal enhancement methods are varied, and some of the most common methods include:
- **Filtering**: Reducing noise components by designing specific filters.
- **Estimation**: Using statistical methods to estimate the value of the signal, such as Kalman filtering.
- **Transform Domain Processing**: Signal processing in the frequency or wavelet domain.
- **Learning Methods**: Using machine learning and other methods to enhance the signal.
### 2.2.3 Introduction to Common Signal Enhancement Algorithms
Signal enhancement algorithms are the key to achieving signal enhancement. Here are some common signal enhancement algorithms:
- **Mean Filtering**: Smoothing the signal's local area using the mean.
- **Median Filtering**: Replacing the central pixel value with the median of the local area to remove sharp noise.
- **Adaptive Filter**: Dynamically adjusting the filter parameters based on the local statistical characteristics of the signal.
- **Wavelet Threshold Denoising**: Performing threshold processing in the wavelet domain to achieve the purpose of denoising.
Each algorithm has its unique characteristics and applicable scenarios. In practical applications, it is necessary to choose the appropriate signal enhancement algorithm based on the characteristics of the signal and the type of noise.
## 2.3 Configuration and Usage of the MATLAB Programming Environment
### 2.3.1 MATLAB Workspace and Variables
The MATLAB workspace is the area where variables, functions, and command history are stored. Variables and commands entered by the user in the MATLAB command window are saved in the workspace, which the user can call and manipulate at any time.
Variables are the basic units of calculation in MATLAB; they can be scalars, vectors, matrices, or higher-dimensional data structures. In MATLAB, variable naming rules follow these principles:
- Variable names consist of letters, numbers, and underscores.
- The first character of a variable name cannot be a number.
- Variable names cannot be the same as MATLAB's built-in function names.
- Variable names are case-sensitive.
### 2.3.2 Programming and Debugging Tips
MATLAB provides two main programming structures: scripts and functions. A script is a collection of commands that can be executed in the command window. A function is a block of code that includes input parameters and return values.
Effectively using debugging tools during the programming process is very important. MATLAB has built-in various debugging tools to help developers quickly locate and correct errors in the code:
- **Breakpoints**: Set breakpoints at specific lines in the code. When the program runs to a breakpoint, it automatically pauses.
- **Step Execution**: Execute the code line by line to observe changes in variables.
- **Data Probes**: Real-time monitoring of variable value changes.
### 2.3.3 Performance Optimization and Code Optimization Strategies
When performing signal processing or complex numerical calculations, optimizing code performance is key to improving efficiency. Here are some strategies for performance optimization and code optimization:
- **Vectorization**: Avoid using loops as much as possible and use vectorized operations to improve execution efficiency.
- **Preallocate Memory**: Allocate arrays of sufficient size before the loop to avoid dynamically adjusting array sizes within the loop.
- **Leverage Built-in Functions**: MATLAB provides many efficient built-in functions. Reasonably utilizing these functions can improve the execution speed of the code.
- **Parallel Computing**: For tasks that can be processed in parallel, MATLAB's Parallel Computing Toolbox can be used to accelerate computation.
By employing these strategies, the running efficiency of MATLAB code can be significantly improved, which is particularly important for scenarios involving large data sets and complex algorithms.
# 3. MATLAB Implementation of Signal Enhancement
## 3.1 Filter-Based Signal Enhancement Techniques
### 3.1.1 Principles and Types of Filter Design
In the field of signal processing, filters are electronic devices or software algorithms used to selectively allow or suppress signals within certain frequency ranges. The purpose of a filter is to allow the desired signal frequency components to pass through while minimizing unwanted noise or interference. Depending on their frequency response, filters can be divided into four basic types: low-pass, high-pass, band-pass, and band-reject.
A low-pass filter (LPF) allows low-frequency signals to pass through and suppresses high-frequency signals, while a high-pass filter (HPF) does the opposite, allowing high-frequency signals to
0
0
相关推荐
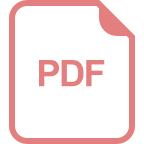
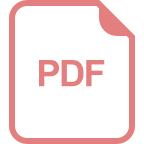
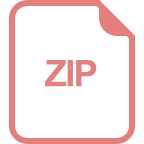





