【In-depth Understanding of MATLAB Spectrum Analysis】: The Mysteries of FFT and IFFT
发布时间: 2024-09-14 10:50:18 阅读量: 35 订阅数: 25 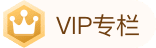
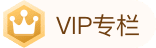
# 1. MATLAB Signal Processing Algorithm Tutorial
Spectral analysis is a core concept in digital signal processing, allowing us to understand the essential characteristics of signals from a frequency domain perspective. Within the MATLAB environment, spectral analysis becomes more intuitive and efficient, thanks to MATLAB's powerful numerical computing capabilities and an extensive library of built-in functions.
## 1.1 Purpose and Significance of Spectral Analysis
The goal of spectral analysis is to extract frequency components from complex time-domain signals to identify their frequency domain characteristics. This technology is widely used in fields such as audio processing, communication systems, and biomedical signal analysis. Through spectral analysis, engineers and scientists can better understand the composition of signals, thereby performing effective signal processing and analysis.
## 1.2 MATLAB's Role in Spectral Analysis
MATLAB provides a range of tools and functions for spectral analysis, making the process from signal acquisition, processing, to visualization simple and efficient. The spectral analysis capabilities of MATLAB support not only basic Fast Fourier Transform (FFT) but also advanced techniques such as window functions and signal filtering, enabling users to quickly transition from theory to practice.
## 1.3 Basic Steps of Spectral Analysis
The basic steps of conducting MATLAB spectral analysis include signal acquisition, preprocessing (such as filtering), FFT transformation, visualization of the frequency spectrum, and analysis and interpretation of the results. This process requires not only proficiency in using MATLAB tools but also a deep understanding of signal processing theory.
```matlab
% MATLAB code example: Simple FFT Analysis
% Let's assume we have a simple sine wave signal
Fs = 1000; % Sampling frequency
t = 0:1/Fs:1-1/Fs; % Time vector
f = 5; % Signal frequency
signal = sin(2*pi*f*t); % Generate sine wave signal
% Perform FFT transformation
Y = fft(signal);
L = length(signal);
P2 = abs(Y/L);
P1 = P2(1:L/2+1);
P1(2:end-1) = 2*P1(2:end-1);
% Define the frequency domain f
f = Fs*(0:(L/2))/L;
% Plot the one-sided amplitude spectrum
figure;
plot(f, P1);
title('Single-Sided Amplitude Spectrum of S(t)');
xlabel('f (Hz)');
ylabel('|P1(f)|');
```
With the above code, we demonstrate how to use MATLAB to perform spectral analysis on a simple sine wave signal. This is just the beginning; MATLAB's powerful analytical capabilities can support more complex signal processing and analysis tasks. As readers gain deeper understanding and practice in spectral analysis, they can explore more advanced features provided by MATLAB to optimize the analysis process.
# 2. Theory and Implementation of the Fast Fourier Transform (FFT)
## 2.1 Basic Principles of the FFT Algorithm
### 2.1.1 Concept of the Discrete Fourier Transform (DFT)
The Discrete Fourier Transform (DFT) is a mathematical method for converting discrete signals in the time domain to the frequency domain. DFT provides a way to observe the frequency components of a signal, working by representing time-domain signals as a linear combination of complex exponential functions. This process can be seen as a sampling and reconstruction process, converting time-domain sampling points into frequency-domain components through the superposition of sine and cosine functions.
In mathematical terms, for a complex number sequence \(x[n]\) of length N, its DFT is defined as:
\[ X[k] = \sum_{n=0}^{N-1} x[n] \cdot e^{-j \frac{2\pi}{N}nk} \]
Here, \(X[k]\) is the frequency component of the complex number sequence \(x[n]\) at frequency \(k\), \(j\) is the imaginary unit, and \(e\) is the base of the natural logarithm.
### 2.1.2 Mathematical Derivation of the FFT Algorithm
The Fast Fourier Transform (FFT) is an efficient algorithm for calculating DFT, proposed by James W. Cooley and John W. Tukey in 1965. FFT significantly reduces the computational complexity of DFT, from the original algorithm's \(O(N^2)\) time complexity to \(O(N \log N)\). This improvement makes large-scale spectral analysis practical.
The core idea of the FFT algorithm is to decompose the original DFT problem into smaller DFT problems and construct the solution of the original problem using the results of these smaller problems. Decomposition typically utilizes a structure called a "butterfly operation." In the recursive divide-and-conquer process, the original DFT of length N is decomposed into two DFTs of length N/2, and then these DFTs are decomposed into four DFTs of length N/4, and so on, until decomposed into the simplest DFT of length 1.
## 2.2 Application of FFT in MATLAB
### 2.2.1 Usage of MATLAB's Built-in FFT Function
MATLAB provides a powerful built-in function `fft` for efficiently computing the fast Fourier transform of signals. Using the `fft` function is straightforward; simply input the signal data to obtain the corresponding frequency domain representation.
For example, for a signal vector x of length N, calculating its FFT requires only one line of code:
```matlab
X = fft(x);
```
The `fft` function returns a complex vector, which includes the magnitude and phase information of the signal's components at different frequencies. To view the signal's amplitude spectrum, the `abs` function can be used to take the modulus; to view the signal's phase spectrum, the `angle` function can be used to take the phase angle.
### 2.2.2 Strategies for Optimizing FFT Performance
In practical applications, FFT performance can be optimized through various strategies. First, the signal length N is often chosen to be a power of 2 for the best performance of the FFT algorithm. MATLAB automatically detects during the execution of `fft` whether the signal length is optimized, and if not, it performs appropriate padding (zero-padding) or truncation.
Another performance optimization strategy is parallel computing. With the prevalence of multi-core processors, MATLAB also provides multi-threading support, allowing multiple data blocks to be processed in parallel during the calculation of FFT. This can be achieved by using the `parfor` loop, ensuring the use of the parallel version of `fft`.
## 2.3 Case Studies of FFT Analysis
### 2.3.1 Spectral Analysis of Audio Signals
Performing spectral analysis on audio signals using FFT is a fundamental operation in audio processing. The following is a simple case study demonstrating how to use MATLAB's `fft` function to analyze the spectrum of an audio signal.
```matlab
% Read audio file
[x, Fs] = audioread('example.wav'); % x is audio data, Fs is sampling frequency
% Compute FFT
X = fft(x);
% Compute frequency vector
N = length(x);
f = (0:N-1)*(Fs/N);
% Plot amplitude spectrum
X_mag = abs(X);
X_mag = X_mag(1:N/2+1);
f = f(1:N/2+1);
figure;
plot(f, X_mag);
title('Audio Signal Spectrum');
xlabel('Frequency (Hz)');
ylabel('Magnitude');
```
In this example, we first read an audio file and then compute its FFT to obtain the frequency components of the signal. Finally, we plot the signal's amplitude spectrum and visually display the frequency distribution of the audio signal through a graph.
### 2.3.2 Spectral Analysis of Power System Signals
In power systems, FFT is widely used to analyze the spectral characteristics of voltage and current signals. This is crucial for detecting harmonic distortions in the grid and monitoring the stability of the power system. The following is a simple case study showing how to use MATLAB to perform FFT analysis of power system signals.
```matlab
% Read power system signal data
load power_data.mat; % Assuming the data file contains voltage or current signals
% Compute FFT
X = fft(signal);
% Compute frequency vector
Fs = sampleRate; % Assuming the sampling frequency is known
N = length(signal);
f = (0:N-1)*(Fs/N);
% Plot amplitude spectrum
X_mag = abs(X);
X_mag = X_mag(1:N/2+1);
f = f(1:N/2+1);
figure;
plot(f, X_mag);
title('Power System Signal Spectrum');
xlabel('Frequency (Hz)');
ylabel('Magnitude');
```
In this example, we load power system signal data and compute its FFT to obtain the frequency components. By plotting the signal's amplitude spectrum, we can observe the frequency components in the power system, such as the fundamental wave and harmonics.
The above cases demonstrate the application of FFT in different fields, not limited to signal processing but also including audio analysis, power system monitoring, etc. Understanding the principle of FFT and its implementation in MATLAB lays a solid foundation for in-depth analysis in these fields.
# 3. Principles and Applications of the Inverse Fast Fourier Transform (IFFT)
## 3.1 Basic Concepts of the IFFT Algorithm
### 3.1.1 Relationship Between IFFT and FFT
The Inverse Fast Fourier Transform (IFFT) is the inverse process of the Fast Fourier Transform (FFT). If FFT is used to convert a time-domain signal into the frequency domain, IFFT is used to convert a frequency-domain signal back into the time domain. Understanding the mathematical relationship between the two is crucial, as they play a core role in signal processing, image pro
0
0
相关推荐
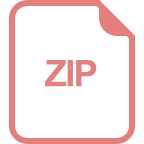
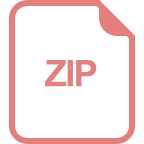
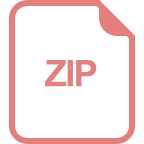
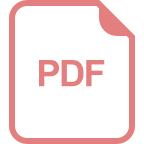
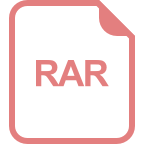
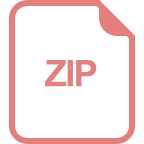
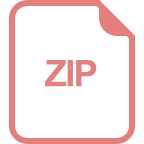
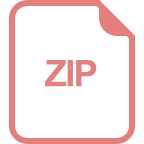
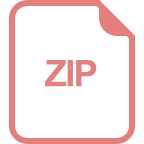