【MATLAB Signal Processing in Practice】: Case Studies from Theory to Application
发布时间: 2024-09-14 11:14:25 阅读量: 44 订阅数: 47 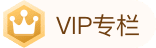
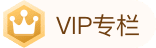
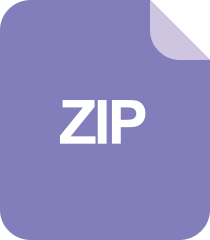
《COMSOL顺层钻孔瓦斯抽采实践案例分析与技术探讨》,COMSOL模拟技术在顺层钻孔瓦斯抽采案例中的应用研究与实践,comsol顺层钻孔瓦斯抽采案例 ,comsol;顺层钻孔;瓦斯抽采;案例,COM
# [MATLAB Signal Processing in Action]: Case Analysis from Theory to Application
In today's engineering and scientific research fields, MATLAB has become one of the indispensable tools, especially in the field of signal processing, where MATLAB offers powerful computing and graphical capabilities. This chapter will guide you into the world of MATLAB signal processing, from theoretical foundations to practical applications, from entry-level to advanced techniques, demonstrating how to solve complex signal processing problems using MATLAB.
## 1.1 The Importance of Signal Processing
Signal processing is a core component of information science and engineering. Its primary task is to improve the availability and accuracy of data by extracting useful information, filtering out noise, and enhancing signal quality. The functions provided by MATLAB in the field of signal processing allow engineers and researchers to focus more on problem-solving rather than tedious computational processes.
## 1.2 The Advantages of MATLAB in Signal Processing
MATLAB is widely popular in the field of signal processing due to its unique set of advantages: First, MATLAB has a rich and efficient built-in function library, which can greatly simplify the complexity of signal processing; second, MATLAB's visualization capabilities allow users to visually observe the effects of signal processing; finally, MATLAB's powerful programming capabilities enable users to develop customized solutions.
## 1.3 Exploring MATLAB Signal Processing Capabilities
The MATLAB Signal Processing Toolbox integrates a large number of pre-designed functions, covering everything from basic signal operations to complex signal analysis and processing techniques. Subsequent sections of this chapter will systematically introduce the features of these toolboxes and demonstrate their practical applications through examples.
By studying this chapter, you will gain a preliminary understanding of MATLAB's role and characteristics in signal processing, laying a solid foundation for further in-depth learning.
# 2. Basic Theories of MATLAB Signal Processing
## 2.1 Basics of Digital Signal Processing
### 2.1.1 Classification of Signals and Systems
In digital signal processing, signals can be classified into continuous-time signals and discrete-time signals. Continuous-time signals are continuously changing and are commonly used in analog systems, while discrete-time signals consist of values at discrete points in time, and are the主角 of digital systems. The classification of systems is also based on the type of signals they process, which can be either continuous-time systems or discrete-time systems. Additionally, there are classifications such as linear systems versus nonlinear systems, and time-invariant systems versus time-varying systems.
```matlab
% MATLAB code example: creation of a discrete-time signal
n = 0:10; % Create a vector from 0 to 10 representing discrete time points
x = sin(2*pi*0.1*n); % Create a discrete sine wave signal
stem(n, x); % Use stem function to graphically represent the signal
```
In this example, we first create a discrete-time sequence `n`, then generate a discrete sine wave signal `x`. We use the `stem` function to graphically represent the signal, allowing us to visually see the values of the signal at different time points.
### 2.1.2 Sampling Theorem and Frequency Domain Analysis
According to Nyquist's Theorem, to accurately recover a continuous signal from a sampled signal, the sampling frequency should be at least twice the highest frequency of the signal. This is one of the foundational theories of digital signal processing, known as the Nyquist Sampling Theorem. In frequency domain analysis, we use the Fourier Transform to convert signals from the time domain to the frequency domain to analyze the frequency components of the signal.
```matlab
% MATLAB code example: perform a Fourier Transform and plot the frequency spectrum
X = fft(x); % Perform a Fast Fourier Transform on signal x
n = length(x); % Signal length
f = (0:n-1)*(1/n); % Generate a frequency vector
plot(f, abs(X)/n); % Plot the normalized frequency spectrum
```
In the above code, we use the `fft` function to perform a Fast Fourier Transform on signal `x`, obtaining the frequency domain representation `X`. We then calculate the corresponding frequency vector `f` and plot the normalized frequency spectrum of the signal, allowing us to see where the signal's energy is concentrated in terms of frequency.
## 2.2 Fourier Transform and Its Applications
### 2.2.1 Fourier Series and Fourier Transform
The Fourier Series is used for periodic signals, representing periodic signals as an infinite sum of sines and cosines of different frequencies. The Fourier Transform, on the other hand, is used for aperiodic signals, decomposing them into continuous frequency components. Both are based on the principle of decomposing complex signals into simple sines and cosines, a core concept in signal processing.
### 2.2.2 Fast Fourier Transform (FFT) Algorithm
The Fast Fourier Transform (FFT) is an efficient algorithm for implementing the Discrete Fourier Transform (DFT). The FFT greatly reduces the computational complexity and is an indispensable tool in digital signal processing. With the FFT, it is possible to complete large-scale frequency spectrum analysis in a shorter time.
```matlab
% MATLAB code example: Fast Fourier Transform (FFT)
Y = fft(x); % Perform a Fast Fourier Transform on signal x
N = 2^n; % Ensure the length of x is a power of 2
f = (0:N-1)*(Fs/N); % Calculate the frequency vector
plot(f, abs(Y)/N); % Plot the normalized frequency spectrum
```
In this code snippet, we first perform a Fast Fourier Transform on signal `x` to obtain `Y`, then calculate the corresponding frequency vector `f`. By plotting the normalized amplitude of `Y`, we can observe the distribution of the signal in the frequency domain.
## 2.3 Filter Design Principles
### 2.3.1 Types and Characteristics of Filters
Filters are core components in signal processing, used to selectively retain or remove certain components of a signal based on frequency. Filters can be classified as low-pass, high-pass, band-pass, and band-stop, among others. Their characteristics are typically defined by their frequency response, including amplitude and phase responses.
### 2.3.2 Analog and Digital Filter Design
Analog filters are designed based on the principles of continuous-time circuits, while digital filters are based on the theory of discrete-time systems. In MATLAB, the design of digital filters can be accomplished using the built-in Filter Design Toolbox, or with specialized functions and methods.
```matlab
% MATLAB code example: design a simple digital low-pass filter
% Use butter function to design a low-pass filter
[N, Wn] = buttord(0.1, 0.3, 3, 60); % Calculate the filter order and cutoff frequency
[b, a] = butter(N, Wn); % Design the filter based on order and cutoff frequency
freqz(b, a); % Plot the filter's frequency response
```
The above code uses the `butter` function to design a Butterworth low-pass filter. We first use the `buttord` function to calculate the minimum order and cutoff frequency of the filter to meet the given passband and stopband ripple requirements. Then, using the `butter` function, we actually design the filter and use the `freqz` function to plot the filter's frequency response.
## 2.4 Summary
In this chapter, we introduced the basic theories of MATLAB signal processing, including the classification of digital signal processing, the sampling theorem and frequency domain analysis, the Fourier Transform and its applications in signal processing, and filter design principles. Understanding these basic theories is crucial for in-depth study of MATLAB signal processing. In the next chapter, we will explore the practical applications of these theories.
# 3. Practical Techniques in MATLAB Signal Processing
## 3.1 Signal Generation and Manipulation in MATLAB
### 3.1.1 Creation and Synthesis of Signals
In the MATLAB environment, creating and synthesizing signals is the first step in signal processing practice. Signals can be simple sine waves, square waves, or more complex signals such as human speech or biomedical signals. With the functions provided by MATLAB, engineers can easily generate these signals and perform further analysis and processing.
One of the most basic functions for signal generation is the `sin` function, used to create sine wave signals. The example is as follows:
```matlab
fs = 1000; % Sampling frequency
t = 0:1/fs:1-1/fs; % Time vector
f = 5; % Signal frequency
signal = sin(2*pi*f*t); % Generate a 5Hz sine wave signal
```
This code first defines the sampling frequency `fs`, then creates a time vector `t`, and uses the sine function to generate a signal with a frequency of 5 Hertz. This is just a simple example of signal creation. In practical applications, signals are often complex and diverse. For example, a voice signal can be read using the `audioread` function to access audio data stored in a file:
```matlab
[signal, fs] = audioread('example.wav'); % Read audio data from a .wav file
```
Next, ***mon functions used for synthesizing signals include `sum`, `conv`, etc.
For instance, to synthesize two sine wave signals with different frequencies, the following code can be used:
```matlab
% Generate two sine wave signals with different frequencies
signal1 = sin(2*pi*5*t);
signal2 = sin(2*pi*20*t);
% Synthesize these two signals
composite_signal = signal1 + signal2;
```
Signal synthesis technology is very useful in the creation of test signals, signal simulation, and other scenarios.
### 3.1.2 Time and Frequency Domain Operations on Signals
In MATLAB, signals can undergo both time domain and frequency domain operations. Time domain operations typically include signal amplification, cropping, translation, and scaling. These operations help to improve the shape of the signal, such as
0
0
相关推荐





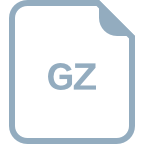
