[Advanced MATLAB Signal Processing]: Multirate Signal Processing Techniques
发布时间: 2024-09-14 11:27:31 阅读量: 52 订阅数: 47 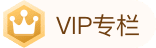
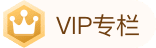
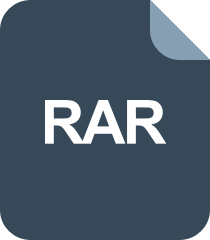
Multirate Filtering for Digital Signal Processing: MATLAB Applications
# Advanced MATLAB Signal Processing: Multirate Signal Processing Techniques
Multirate signal processing is a core technology in the field of digital signal processing, allowing the conversion of digital signals between different rates without compromising signal quality or introducing unnecessary noise. This chapter introduces the basic concepts and theories of multirate signal processing, laying the foundation for subsequent chapters that delve into sampling rate conversion, system design and optimization, and MATLAB implementation.
## 1.1 The Purpose and Applications of Multirate Processing
The core purpose of multirate signal processing is to convert signals between different sampling rates to meet the requirements of various processing modules or transmission media. This is particularly common in communication systems, audio and video processing, and data storage. For instance, to reduce storage needs or bandwidth usage, the sampling rate is often decreased; conversely, for signal analysis or high-quality reconstruction, the sampling rate may need to be increased.
## 1.2 Rate Conversion in Digital Signal Processing
In digital signal processing, sampling rate conversion means changing the signal's sampling frequency. This process often involves interpolation and decimation operations, which can be viewed as interpolation and downsampling in discrete-time signal processing. These operations enable signal compression or expansion without affecting the signal's key information content.
## 1.3 Advantages of Multirate Signal Processing
Compared to traditional single-rate signal processing methods, multirate processing has significant advantages. These advantages include, but are not limited to: enhancing the flexibility of signal processing systems, reducing the amount of data stored and transmitted, lowering the computational complexity and cost of the system. Additionally, multirate technology can reduce aliasing and mirror effects in signal processing through filter design.
In the next chapter, we will delve into the theory of sampling rate conversion, including its basic concepts and the key role it plays in multirate signal processing. We will use theoretical analysis and practical examples to help readers better understand and master the core technology of multirate signal processing.
# 2. Sampling Rate Conversion Theory
## 2.1 Basic Concepts of Sampling Rate Conversion
### 2.1.1 Sampling Theorem and Multirate Processing
The sampling theorem, also known as the Nyquist theorem, is a fundamental theory in signal processing. It explains how to correctly sample continuous signals to reconstruct the original signal. The Nyquist theorem states that to avoid aliasing, the sampling frequency must be at least twice the highest frequency of the signal.
In multirate signal processing, sampling rate conversion is a core concept. This technology allows us to change the sampling rate of a signal, which is very important in digital audio processing, image processing, and communication systems. For example, to reduce data transmission bandwidth or storage requirements, we may need to decrease the sampling rate; whereas to meet specific hardware requirements or improve signal quality, we may need to increase the sampling rate.
### 2.1.2 Sampling Rate Conversion Factor and Filter Design
The sampling rate conversion factor is defined as the ratio of the original sampling rate to the target sampling rate. It describes the degree to which the sampling rate needs to be increased or decreased. This factor can be fractional, requiring not only upsampling (increasing sampling points) or downsampling (decreasing sampling points) but also interpolation (adding new sampling points) or decimation (deleting existing sampling points).
Filter design is a critical aspect of sampling rate conversion. Ideally, we need a low-pass filter to ensure that the signal frequency does not exceed half the Nyquist frequency. However, actual filters are often non-ideal and will allow some frequencies above this to pass through, which can cause aliasing. Therefore, filter design must尽量 reduce aliasing and closely approximate the characteristics of the ideal filter.
## 2.2 Design of Lowpass Interpolation Filters
### 2.2.1 The Difference Between Ideal and Real Filters
An ideal low-pass filter can completely block signal components above the cutoff frequency while allowing low-frequency components to pass through completely. However, real filters cannot achieve this ideal frequency response; they usually have a transition band where frequency components are gradually attenuated to a complete stop.
Actual filter design requires a balance between performance and implementation complexity. A common type of real filter is the Finite Impulse Response (FIR) filter, which has linear phase characteristics and can improve performance by increasing the filter order. Another type is the Infinite Impulse Response (IIR) filter, which can provide higher performance but may introduce phase distortion.
### 2.2.2 Filter Design Methods and Performance Evaluation
Designing a good filter requires considering various factors, including the desired amount of attenuation, transition band width, and implementation complexity. Filter design methods typically include window functions and least squares methods.
Performance evaluation usually focuses on the filter's frequency response, including passband ripple, stopband attenuation, and phase response. In multirate processing, the filter's performance has a significant impact on the system's overall performance. Therefore, the design process requires frequent evaluation of filter performance and adjustments to meet application requirements.
## 2.3 Principles and Applications of Polyphase Filters
### 2.3.1 Introduction to Polyphase Filter Structures
Polyphase filters are an efficient structure in sampling rate conversion, which improves efficiency by decomposing a standard filter into several smaller filters. The advantage of this approach is that it reduces the use of multipliers, thereby lowering computational complexity.
During downsampling, polyphase filters can be used to separate the filtered signal, retaining only the signal components within the desired frequency range. During upsampling, the polyphase structure is used for interpolation and signal reconstruction, which usually involves signal interpolation and corresponding filtering processes.
### 2.3.2 The Role of Polyphase Filters in Sampling Rate Conversion
An important application of polyphase filters is in multirate filter banks. It allows for more flexible frequency division, which is crucial for applications such as subband coding and digital receivers.
In practical applications, polyphase filters can leverage their structural characteristics to reduce computational effort. For example, in a multirate processing system, polyphase filters can provide more efficient upsampling and downsampling operations by changing the input order of the subfilters and the output sequence of the subfilters.
# 3. MATLAB Implementation of Multirate Signal Processing
## 3.1 The Basis of MATLAB Applications in Signal Processing
### 3.1.1 Introduction to the MATLAB Signal Processing Toolbox
MATLAB, as a high-performance mathematical computing and visualization software, possesses powerful signal processing capabilities, primarily due to its included Signal Processing Toolbox. This toolbox provides users with a wide range of functions, from signal generation, filtering, transformation to spectral analysis, virtually all common signal processing tasks can be quickly implemented through the functions in the toolbox. Especially for multirate signal processing, MATLAB provides specialized functions and tools that can help users easily design and implement complex signal processing systems.
### 3.1.2 Setting Up the MATLAB Programming Environment
Before engaging in multirate signal processing, it is essential to ensure that the MATLAB programming environment is correctly set up. This includes installing the appropriate MATLAB version and related toolboxes. Generally, at a minimum, the Signal Processing Toolbox needs to be installed. For multirate signal processing, the Image Processing Toolbox is also required to handle image data. Once installed, a basic understanding of MATLAB's interface is necessary, familiarizing oneself with the Command Window, Editor, Workspace, and Path Management. Furthermore, for multirate signal processing, mastering how to use MATLAB's Simulink tool will be very useful because Simulink provides an intuitive drag-and-drop interface for users to easily build and test complex signal processing workflows.
## 3.2 MATLAB Implementation of Multirate Signal Processing
### 3.2.1 MATLAB Signal Generation and Analysis
Generating signals in MATLAB is very straightforward. For example, to create a simple sine wave signal, the following code can be used:
```matlab
Fs = 1000; % Sampling frequency
t = 0:1/Fs:1-1/Fs; % Time vector
f = 5; % Signal frequency
A = 1; % Signal amplitude
y = A*sin(2*pi*f*t); % Sine wave signal
```
For complex signals, such as audio or images, MATLAB's built-in functions can be used to import and process. For example, use `audioread` and `imread` to read audio and image files, respectively.
Signal analysis is an important part of signal processing. MATLAB provides a wide range of functions to analyze various attributes of signals, including spectral analysis, time-frequency analysis, etc. For example, the `fft` function can be used to compute the Fast Fourier Transform (FFT) of a signal:
```matlab
Y = fft(y);
P2 = abs(Y/N); % Two-sided spectrum
P1 = P2(1:N/2+1); % One-sided spectrum
P1(2:end-1) = 2*P1(2:end-1);
f = Fs*(0:(N/2))/N;
```
### 3.2.2 Using and Programming Sampling Rate Conversion Functions
MATLAB provides various functions for sampling rate conversion, the most commonly used including `resample`, `decimate`, and `intfilt`, among others. For example, to reduce the sampling rate of a signal from Fs to Fs/4, the `decimate` function can be used:
```matlab
y_decimated = decimate(y, 4);
```
For more complex sampling rate conversions, the `resample` function can be used. This function allows users to specify a new sampling rate and the bandwidth of an anti-aliasing filter, enabling more flexible signal processing.
```matlab
y_resampled = resample(y, 1, 4);
```
When using these functions for sampling rate conversion,
0
0
相关推荐







