Filter Design in MATLAB: Theory and Practice
发布时间: 2024-09-14 10:47:56 阅读量: 8 订阅数: 17 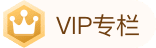
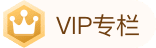
# Chapter 1: Fundamentals of MATLAB Filter Design
In digital signal processing, filter design is a fundamental and core task. MATLAB, as a powerful mathematical computation and engineering simulation software, offers a wealth of tools and functions for filter design, enabling engineers and researchers to easily achieve complex design requirements. This chapter will lay the foundation for MATLAB filter design, covering all the important links from conceptual understanding to concrete implementation.
## 1.1 The Role and Importance of Filters
Filters can allow or block certain parts of a signal to pass through based on specific frequency characteristics. In signal processing, filters are used for extracting useful signals, suppressing interference or noise, and performing spectral analysis. They play a key role in many fields such as communication, radar, audio processing, and medical imaging.
## 1.2 The Role of MATLAB in Filter Design
MATLAB provides various built-in functions and tools for designing and analyzing filters. These tools are suitable not only for teaching and theoretical research but also for practical engineering applications. With MATLAB, designers can quickly perform algorithm validation, performance evaluation, and achieve efficient code writing.
## 1.3 Understanding the Basic Steps of Filter Design
Generally, filter design includes several key steps: determining filter specifications, selecting an appropriate filter type, calculating filter coefficients, and implementing the filter design. In the MATLAB environment, these steps can be accomplished by writing scripts or using interactive tools. In subsequent chapters, we will gradually elaborate on these contents and provide detailed guidance on how to use MATLAB's powerful features to complete each step of the design work.
# Chapter 2: Filter Design Theory
### 2.1 Basic Concepts of Digital Filters
Digital filters are an important part of digital signal processing, capable of altering a signal'***pared to analog filters, digital filters offer higher stability and accuracy and can achieve complex filtering characteristics that are difficult for analog filters.
#### 2.1.1 Classification and Function of Filters
Filters can be classified into four basic types based on their frequency response characteristics: low-pass, high-pass, band-pass, and band-stop. A low-pass filter allows signals below the cutoff frequency to pass through; a high-pass filter allows signals above the cutoff frequency; a band-pass filter allows signals within a certain frequency range to pass through, while a band-stop filter does the opposite, blocking signals within that frequency range.
In digital signal processing, filters are also commonly divided into two main categories: Infinite Impulse Response (IIR) and Finite Impulse Response (FIR). The impulse response of an IIR filter will continue indefinitely, and their design is often based on analog filter theory, such as Butterworth or Chebyshev. The impulse response of an FIR filter will completely disappear after a finite amount of time, and it has strict linear phase characteristics, making it ideal for precise signal processing.
#### 2.1.2 Performance Indicators of Filters
Filter design performance indicators include the amplitude response of the passband and stopband, transition band width, phase distortion, group delay, stability, and computational complexity. The amplitude response describes the gain of the filter in the passband and stopband, while the transition band width describes the rate of change between the passband and stopband. Ideally, we want the transition band to be as narrow as possible to improve signal processing selectivity. However, in actual design, trade-offs need to be made among different performance indicators.
Phase distortion may be a critical factor for certain signal processing applications, especially in audio and image processing. For example, in audio processing, phase distortion can affect the waveform of sound waves, thereby affecting sound quality. Group delay is an important measure of phase distortion, describing the delay difference of different frequency signals passing through the filter.
### 2.2 Mathematical Principles of Filter Design
#### 2.2.1 Frequency Response and Z-Transform
One of the keys to filter design is understanding frequency response, that is, how the filter responds to signals of different frequencies. In the Z-domain, the frequency response of a filter is described by the magnitude of the Z-transform. The Z-transform is a generalization of the Fourier transform for discrete-time signals and is used for frequency domain analysis of these signals.
For a digital filter, its frequency response H(e^jω) is defined as:
```math
H(e^{j\omega}) = \sum_{n=-\infty}^{\infty} h[n]e^{-j\omega n}
```
Where `h[n]` is the impulse response of the filter, `ω` is the angular frequency, with values ranging from 0 to π corresponding to frequencies from 0 to half the sampling frequency. The magnitude of the Z-transform `|H(e^{j\omega})|` gives the amplitude response of the filter, while its argument `∠H(e^{j\omega})` gives the phase response of the filter.
#### 2.2.2 Overview of Filter Design Methods
There are many methods for filter design, including but not limited to the window function method, frequency sampling method, and optimization design method. Each method has its characteristics and applicable scenarios.
The window function method controls the amplitude response of the filter by choosing an appropriate window function. The frequency sampling method directly samples the ideal frequency response in the frequency domain and then obtains the time-domain impulse response through the inverse Z-transform. The optimization design method obtains filter coefficients by designing a target function and optimizing it, such as the least squares method and Chebyshev approximation.
### 2.3 Window Function Method in Filter Design
#### 2.3.1 Types and Characteristics of Window Functions
The window function method is a common approach for designing FIR filters. It defines an ideal filter'***mon window functions include the rectangular window, Hamming window, Hanning window, and Blackman window.
The choice of window function affects the performance indicators of the filter, such as transition band width and side-lobe level. For example, the rectangular window has the highest side-lobe level, while the Blackman window has the lowest but with a larger transition band width. Choosing the appropriate window function is crucial for designing a satisfactory filter.
#### 2.3.2 Steps of Designing Filters with the Window Function Method
Designing an FIR filter typically includes the following steps:
1. Determine the filter's performance indicators, such as cutoff frequency, stopband attenuation, and passband ripple.
2. Based on the performance indicators, calculate the length of the ideal filter's impulse response and the shape of the required window function.
3. Truncate the ideal filter's impulse response with the selected window function to obtain the actual filter coefficients.
4. Implement the filter design using the obtained filter coefficients and perform frequency response analysis and verification.
5. If necessary, iteratively optimize the filter coefficients.
### 2.4 Frequency Sampling Method in Filter Design
#### 2.4.1 Principles of the Frequency Sampling Method
The frequency sampling method is a straightforward approach to filter design. It directly samples the ideal frequency response of the filter in the frequency domain and then obtains the FIR filter's time-domain coefficients through an inverse transform. This method is particularly suitable for applications with special requirements for phase characteristics because it can provide precise linear phase characteristics.
The key to the frequency sampling method lies in how to choose the values of the sampling points to approximate the ideal frequency response. The choice of sampling points needs to consider the filter's performance indicators, such as the ripple requirements in the passband and stopband.
#### 2.4.2 Application Example of the Frequency Sampling Method
Suppose we design a low-pass FIR filter with a cutoff frequency of `ωc` and a sampling rate of `ωs`. The design steps are as follows:
1. Determine the filter's performance indicators, such as cutoff frequency and stopband attenuation.
2. Select appropriate frequency sampling points `k`, ranging from `0` to `N-1`, where `N` is the length of the filter.
3. For each sampling point `k`, set its corresponding ideal frequency response `Hd[k]`.
4. Apply the inverse Discrete Fourier Transform (IDFT) algorithm to convert frequency domain coefficients to time domain coefficients `h[n]`.
```math
h[n] = \frac{1}{N} \sum_{k=0}^{N-1} Hd[k] e^{j\frac{2\pi}{N}kn}
```
5. Apply the obtained filter coefficients for signal processing and perform performance evaluation.
Filters designed using the frequency sampling method can ensure precise linear phase characteristics, but due to the limitations between the number of frequency sampling points and the length of the time-domain filter, this method may require longer filter coefficients to meet design requirements.
# Chapter 3: Filter Design Tools in MATLAB
In this cha
0
0
相关推荐
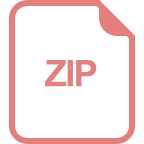
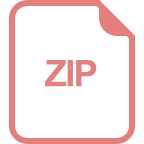
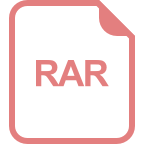
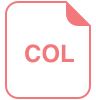
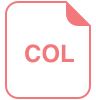
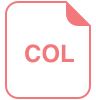
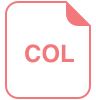

