Detailed Application of Window Functions in MATLAB Signal Processing
发布时间: 2024-09-14 10:59:19 阅读量: 36 订阅数: 30 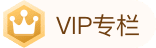
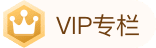
# 1. Signal Processing and Window Function Fundamentals
In digital signal processing, signals are typically represented through discrete samples. These samples are temporally finite, whereas the actual physical signals might be infinite. To accurately extract information from these finite samples, we need to preprocess the signal appropriately, ensuring its stability and controllability. The **window function** plays a crucial role in this process.
## 1.1 Window Functions in Signal Processing
Within the realm of signal processing, window functions are primarily used to reduce spectral leakage caused by signal truncation. Spectral leakage refers to the phenomenon where frequency components of a signal "leak" into other frequencies where they should not appear in the frequency domain, which can obscure the results of signal spectrum analysis. By applying window functions, we can smooth the edges of the signal, reducing this leakage phenomenon and obtaining more accurate spectral information.
## 1.2 Basic Concepts of Window Functions
Window functions can be seen as a type of weighting function that multiplies the signal to adjust the contribution of different parts of the signal. In the time domain, window functions typically start from zero, increase to a maximum value, and then decrease back to zero. This shape, reminiscent of a window, ensures that the start and end of the signal gradually decay to zero, thereby reducing spectral leakage. Different window functions have different characteristics, such as the Hanning window, Hamming window, Blackman window, etc., and they have varying resolution and side lobe attenuation properties in the frequency domain, making them suitable for different signal processing scenarios.
## 1.3 The Importance of Window Functions
The significance of window functions in signal processing cannot be understated. Besides reducing spectral leakage, they can also be used to improve the speed of signal processing and the accuracy of signal detection. Proper selection and application of window functions can significantly enhance the performance of digital signal processing systems.
In summary, as a foundational concept in the field of signal processing, window functions have a profound impact on the entire digital signal processing system. This chapter will introduce the basic theories and applications of window functions to lay a solid foundation for more in-depth discussions and practices in subsequent chapters.
# 2. Theoretical Knowledge of Window Functions
## 2.1 The Role of Window Functions in Signal Processing
### 2.1.1 Signal Spectral Leakage Problem and Solutions
In signal processing, when we截取 an infinitely long signal to obtain a finite length signal, the abrupt changes at the signal's edges due to the截断operation will cause side lobes to appear in the frequency domain, resulting in spectral leakage. This leakage can interfere with our analysis of the signal spectrum, making it difficult to accurately distinguish between different frequency components of the signal.
To address this issue, the concept of window functions was introduced. Window functions effectively reduce spectral leakage by weighting the window to truncate the original signal, making the signal's spectrum more concentrated and thus improving the accuracy of signal analysis. Different window functions have different frequency domain characteristics, and by reasonably selecting the window function, one can specifically suppress side lobes, control leakage, and obtain a clearer spectrum.
### 2.1.2 Classification and Characteristics of Window Functions
Window functions can be classified into various types based on their shape, mathematical expressions, and frequency domain characteristics, with common window functions including the rectangular window, Hanning window, Hamming window, and Blackman window. Each window function has unique characteristics in both the time and frequency domains.
- Rectangular window: In the time domain, the rectangular window is equivalent to not applying any weighting and simply truncating the signal. Its frequency domain characteristic is that the main lobe width is narrowest, but the side lobe attenuation is very low, resulting in the most severe leakage.
- Hanning and Hamming windows: These two window functions smooth the signal in the time domain through a cosine function, reducing the impact of time-domain abrupt changes on the frequency domain. Their frequency domain main lobe width is wider than that of the rectangular window, but the side lobe attenuation is much greater, thereby reducing leakage.
- Blackman window: This is a window function with a narrower main lobe and better side lobe attenuation, but due to the wider main lobe, it can lead to a decrease in frequency domain resolution.
### 2.2 Mathematical Models and Design of Window Functions
#### 2.2.1 Time and Frequency Domain Characteristics of Window Functions
The characteristics of window functions in the time and frequency domains must be considered when designing and applying window functions. Time domain characteristics describe the shape of the window function in the time domain, while frequency domain characteristics reflect the impact of the window function on the signal spectrum. Frequency domain characteristics are usually obtained through Fourier transforms.
#### 2.2.2 Mathematical Expressions of Common Window Functions
Each window function has its corresponding mathematical expression, which precisely describes its variation规律in the time domain. For example, the Hamming window can be represented as:
```
w(n) = 0.54 - 0.46 * cos(2πn / N), n = 0, 1, ..., N-1
```
where `w(n)` is the value of the window function at the nth sampling point, and `N` is the window length.
#### 2.2.3 Theoretical Basis for Selection of Window Functions
The choice of window functions typically depends on specific application requirements and signal characteristics. If higher frequency resolution is needed, a window function with a narrower main lobe should be chosen; if smaller side lobe leakage is required, a window function with greater side lobe attenuation should be selected.
## 2.3 Performance Evaluation Indicators for Window Functions
### 2.3.1 Frequency Domain Resolution and Main Lobe Width
Frequency domain resolution refers to the ability of a window function to distinguish between two adjacent frequency components in the frequency domain, while the main lobe width refers to the width of the window function's spectrum main lobe. Both are directly related to the accuracy of spectral analysis.
### 2.3.2 Side Lobe Attenuation and Out-of-Band Leakage
Side lobe attenuation refers to the decibel drop of the side lobes relative to the main lobe peak, and higher attenuation can reduce the interference of out-of-band signals. Out-of-band leakage refers to the energy distribution of the window function in the frequency domain outside the main lobe, describing the severity of the leakage.
### 2.3.3 Computational Complexity and Implementation Efficiency
The computational complexity and implementation efficiency of window functions affect the design of the entire signal processing system. Simple window functions usually have lower computational complexity and are easy to implement, while more complex window functions may bring higher computational loads, which needs to be balanced in practical applications.
When selecting window functions, these performance indicators need to be comprehensively considered to meet specific application requirements. For example, in radar signal processing, higher side lobe attenuation might be needed to reduce noise interference, while in audio analysis, greater attention might be paid to frequency resolution and main lobe width.
Through the introduction of this chapter, we can see the importance of window functions in signal processing and how to choose appropriate window functions according to different needs. The next chapter will continue to explore the practical application of window functions in MATLAB, diving into the operational level and demonstrating how to apply window functions in signal analysis and processing.
# 3. Practical Application of MATLAB Window Functions
## 3.1 MATLAB Implementation of Window Functions
### 3.1.1 Usage of Window Function Generation Functions
MATLAB provides various window function generation functions, such as `rectwin`, `hamming`, `hann`, `blackman`, `kaiser`, etc., corresponding to different types of window functions. These functions can generate a window vector of a given length, which can then be applied to signal processing.
For example, to generate a 256-point Hamming window, the following MATLAB code can be used:
```matlab
N = 256; % Window length
window = hamming(N); % Generate Hamming window
```
After executing the above code, the `window` variable contains the Hamming window data with a length of 256. Each window function has its specific mathematical expression, and the window vector generated by the `hamming` function is based on the calculation of `0.54 - 0.46*cos(2*pi*(0:N-1)'/N)`.
### 3.1.2 Customization and Tuning of Window Function Parameters
In practical applications, depending on different signal processing needs, it may be necessary to customize the parameters of the window function. MATLAB also supports the design of custom window functions by adjusting the parameters of the window function to meet specific performance requirements.
For example, to design a Kaiser window with custom parameters, the `kaiser` function can be used and its beta value (β) specified:
```matlab
N = 256; % Window length
beta = 6; % Beta value
window = kaiser(N, beta); % Generate Kaiser window
```
By changing the `beta` value, the degree of side lobe attenuation can be adjusted, thereby affecting the spectral leakage and side lobe levels. Adjusting these parameters needs to be considered in conjunction with specific signal processing problems, such as enhancing the main lobe in some cases to obtain higher frequency resolution, while in other cases, reducing side lobes to lower noise interference.
## 3.2 Application of Window Functions in Signal Analysi
0
0
相关推荐
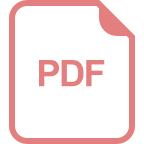
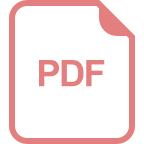
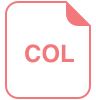
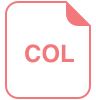
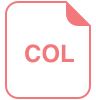
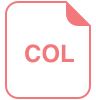
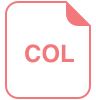
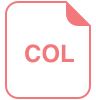