cannot import name 'Dense' from 'keras.models' (F:\pythonProject2\lib\site-packages\keras\models.py)
时间: 2023-09-06 12:14:13 浏览: 52
This error occurs when the code is trying to import the Dense layer from keras.models, but it cannot find it.
There could be several reasons for this issue:
1. The version of Keras being used is outdated and does not have the Dense layer. Try updating Keras to the latest version.
2. There might be a naming conflict in the code. Check if any other modules or packages are using the name Dense.
3. It is possible that the installation of Keras is corrupted. Try reinstalling Keras.
4. Try importing the Dense layer directly from the keras.layers module instead of keras.models.
Example:
```
from keras.layers import Dense
```
This should resolve the issue.
相关问题
cannot import name 'Dense' from 'keras.models'
This error suggests that the `Dense` module could not be imported from the `keras.models` library. This could occur due to a few reasons:
1. The `keras` library is not installed: Ensure that `keras` is installed on your system. You can install it using pip: `pip install keras`.
2. The version of `keras` is outdated: If you already have `keras` installed, the issue could be that the version is outdated. Try updating it to the latest version: `pip install keras --upgrade`.
3. Incorrect import statement: Ensure that you are importing `Dense` from the correct module. The correct import statement should be: `from keras.layers import Dense`.
4. Conflicting packages: There may be conflicting packages or versions of packages on your system. Try uninstalling and reinstalling `keras` to ensure a clean installation.
D:\Python37\python.exe D:/PycharmProjects/TensorFlow/Load-forecasting/Electric-Power-Hourly-Load-Forecasting-using-Recurrent-Neural-Networks-master/load_forecasting.py Traceback (most recent call last): File "D:/PycharmProjects/TensorFlow/Load-forecasting/Electric-Power-Hourly-Load-Forecasting-using-Recurrent-Neural-Networks-master/load_forecasting.py", line 5, in <module> from keras.layers.core import Dense, Activation, Dropout File "D:\Python37\lib\site-packages\keras\__init__.py", line 21, in <module> from keras import models File "D:\Python37\lib\site-packages\keras\models\__init__.py", line 18, in <module> from keras.engine.functional import Functional File "D:\Python37\lib\site-packages\keras\engine\functional.py", line 26, in <module> from keras import backend File "D:\Python37\lib\site-packages\keras\backend.py", line 32, in <module> from keras import backend_config File "D:\Python37\lib\site-packages\keras\backend_config.py", line 33, in <module> @tf.__internal__.dispatch.add_dispatch_support AttributeError: module 'tensorflow.compat.v2' has no attribute '__internal__' 进程已结束,退出代码为 1
根据您提供的错误信息,看起来您在运行一个脚本时遇到了模块导入错误。脚本中的 `load_forecasting.py` 文件无法导入 `keras` 模块的一些组件。
这个问题通常是由版本不兼容或依赖项问题引起的。在最新版本的 TensorFlow 中,`keras` 被整合到 TensorFlow 的核心中,而不再是单独的模块。由于这种变化,可能会导致一些旧脚本在导入 `keras` 组件时出现错误。
为了解决这个问题,您可以尝试以下方法:
1. 更新依赖项:
- 确保您的 TensorFlow 和 Keras 版本是最新的。您可以使用 `pip` 命令更新它们,例如 `pip install --upgrade tensorflow keras`。
- 更新其他相关依赖项,如 NumPy、Pandas 等。
2. 修改导入语句:
- 如果您的 TensorFlow 版本是 2.x,可以尝试将导入语句修改为 `from tensorflow.keras.layers import Dense, Activation, Dropout`。
- 如果您使用的是旧版本的 TensorFlow(1.x),则修改导入语句为 `from keras.layers.core import Dense, Activation, Dropout`。
请根据上述建议检查和调试您的代码,以解决模块导入错误。如果问题仍然存在,请提供更多关于脚本、项目结构和依赖项的信息,我将尽力提供更多帮助。
相关推荐
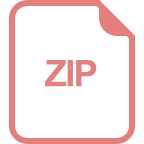
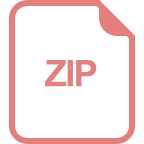
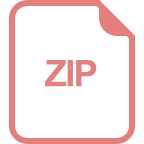













