from sklearn.metrics import roc_curve,auc,confusion_matrix import pandas as pd import statsmodels.api as sm import matplotlib.pyplot as plt import numpy as np w=pd.read_csv("D:/pythonProject/venv/Trans.csv")#加载文件 w['intercept']=1.0#设定截距 x_c=w.columns[[4,0,1,2]];y_c=w.columns[3] X=w[x_c];y=w[y_c] X=np.array(X);y=np.array(y) zid=np.ones(len(y));zid[:int(len(y)*0.2)]=0 x_x=X[zid==1,:];y_x=y[zid==1] x_t=X[zid==0,:];y_t=y[zid==0] result=sm.Logit(y_x,x_x).fit() print(result.summary) y_p= result.predict(x_t) fpr,tpr, thresholds = roc_curve(y_t,y_p)# roc_auc=auc(fpr,tpr)#算auc的函数 print("Area under the ROC curve : %f" % roc_auc)#算出auc #画图 plt.plot(fpr,tpr,label='ROC')#画基本表 plt.xlabel('FPR')#x轴为fpr,假真率 plt.ylabel('TPR')#y轴是tpr,真真率 #得出结果 print(thresholds[np.argmin(np.abs(tpr-(1-fpr)))])#算最优阈值 print(np.sum(y_t!=1*(y_p>0.3421))/len(y_t))#算误判率 plt.show()#画图
时间: 2024-03-04 11:49:01 浏览: 116
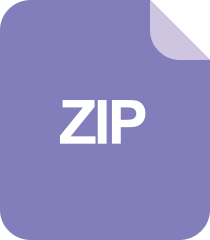
metrics-sdks-node:[只读]适用于Node.js的ReadMe Metrics SDK的镜像
这段代码是一个用于二分类问题的 logistic 回归模型,并使用 ROC 曲线和 AUC 值来评估模型性能的例子。具体解释如下:
1. 加载数据:
```python
w=pd.read_csv("D:/pythonProject/venv/Trans.csv")#加载文件
w['intercept']=1.0#设定截距
x_c=w.columns[[4,0,1,2]];y_c=w.columns[3]
X=w[x_c];y=w[y_c]
X=np.array(X);y=np.array(y)
zid=np.ones(len(y));zid[:int(len(y)*0.2)]=0
x_x=X[zid==1,:];y_x=y[zid==1]
x_t=X[zid==0,:];y_t=y[zid==0]
```
这段代码中,首先读取了一个 CSV 文件,然后根据数据的特点对数据进行了一些预处理,如添加了一个截距项,然后将数据集分成了训练集和测试集,比例为 8:2。
2. 训练模型:
```python
result=sm.Logit(y_x,x_x).fit()
print(result.summary)
```
这段代码中,使用了 statsmodels 库的 Logit 函数来训练逻辑回归模型,并输出了模型的摘要信息。
3. 预测测试集结果并计算 ROC 曲线和 AUC 值:
```python
y_p= result.predict(x_t)
fpr,tpr, thresholds = roc_curve(y_t,y_p)# roc_auc=auc(fpr,tpr)#算auc的函数
print("Area under the ROC curve : %f" % roc_auc)#算出auc
```
这段代码中,首先使用训练好的模型对测试集进行了预测,得到了每个样本属于正类的概率。然后使用 sklearn 库的 roc_curve 函数计算了 ROC 曲线上的 FPR 和 TPR,并使用 auc 函数计算了 ROC 曲线下的面积 AUC。
4. 输出结果并画图:
```python
print(thresholds[np.argmin(np.abs(tpr-(1-fpr)))])#算最优阈值
print(np.sum(y_t!=1*(y_p>0.3421))/len(y_t))#算误判率
plt.show()#画图
```
这段代码中,首先计算了最优阈值和误判率。最优阈值是使得 FPR 和 TPR 的差值最小的阈值,误判率是分类错误的样本数占总样本数的比例。然后使用 matplotlib 库画出了 ROC 曲线的图像。
阅读全文
相关推荐
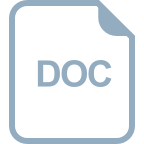
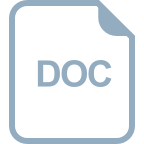








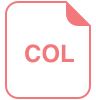






