notimplementederror: formatting_info=true not yet implemented
时间: 2023-04-27 18:06:25 浏览: 77
"NotImplementedError: formatting_info=true not yet implemented" 的意思是“尚未实现 formatting_info=true”。这个错误通常出现在使用 Python 中的 xlrd 库时,当尝试读取 Excel 文件的格式信息时会出现这个错误。这是因为 xlrd 库目前还不支持读取 Excel 文件的格式信息。如果需要读取格式信息,可以考虑使用其他库或工具来实现。
相关问题
xlutils.copy(xlrd.open_workbook('old.xls', formatting_info=True)).save(''new.xls')报错descriptor 'decode' for 'bytes' objects doesn't apply to a 'NoneType' object
这个错误的原因是你使用了`formatting_info=True`参数,但是你的`old.xls`文件中没有任何格式信息,所以`xlrd.open_workbook`返回了一个空的`workbook`对象,而空对象没有任何解码方法,因此会出现这个错误。
你可以通过以下两种方式解决这个问题:
1. 将`formatting_info=True`参数移除,这样`xlrd.open_workbook`函数将不会读取格式信息,而只会读取单元格的内容和公式。
2. 确保你的`old.xls`文件包含格式信息。你可以通过在Excel中手动设置一些格式,然后保存文件,并使用`formatting_info=True`参数重新读取文件来测试是否成功。如果成功,那么你可以在代码中使用相同的格式来生成新的Excel文件。
优化代码 def fault_classification_wrapper(vin, main_path, data_path, log_path, done_path): start_time = time.time() isc_path = os.path.join(done_path, vin, 'isc_cal_result', f'{vin}_report.xlsx') if not os.path.exists(isc_path): print('No isc detection input!') else: isc_input = isc_produce_alarm(isc_path, vin) ica_path = os.path.join(done_path, vin, 'ica_cal_result', f'ica_detection_alarm_{vin}.csv') if not os.path.exists(ica_path): print('No ica detection input!') else: ica_input = ica_produce_alarm(ica_path) soh_path = os.path.join(done_path, vin, 'SOH_cal_result', f'{vin}_sohAno.csv') if not os.path.exists(soh_path): print('No soh detection input!') else: soh_input = soh_produce_alarm(soh_path, vin) alarm_df = pd.concat([isc_input, ica_input, soh_input]) alarm_df.reset_index(drop=True, inplace=True) alarm_df['alarm_cell'] = alarm_df['alarm_cell'].apply(lambda _: str(_)) print(vin) module = AutoAnalysisMain(alarm_df, main_path, data_path, done_path) module.analysis_process() flags = os.O_WRONLY | os.O_CREAT modes = stat.S_IWUSR | stat.S_IRUSR with os.fdopen(os.open(os.path.join(log_path, 'log.txt'), flags, modes), 'w') as txt_file: for k, v in module.output.items(): txt_file.write(k + ':' + str(v)) txt_file.write('\n') for x, y in module.output_sub.items(): txt_file.write(x + ':' + str(y)) txt_file.write('\n\n') fc_result_path = os.path.join(done_path, vin, 'fc_result') if not os.path.exists(fc_result_path): os.makedirs(fc_result_path) pd.DataFrame(module.output).to_csv( os.path.join(fc_result_path, 'main_structure.csv')) df2 = pd.DataFrame() for subs in module.output_sub.keys(): sub_s = pd.Series(module.output_sub[subs]) df2 = df2.append(sub_s, ignore_index=True) df2.to_csv(os.path.join(fc_result_path, 'sub_structure.csv')) end_time = time.time() print("time cost of fault classification:", float(end_time - start_time) * 1000.0, "ms") return
Here are some suggestions to optimize the code:
1. Use list comprehension to simplify the code:
```
alarm_df = pd.concat([isc_input, ica_input, soh_input]).reset_index(drop=True)
alarm_df['alarm_cell'] = alarm_df['alarm_cell'].apply(str)
```
2. Use context manager to simplify file operation:
```
with open(os.path.join(log_path, 'log.txt'), 'w') as txt_file:
for k, v in module.output.items():
txt_file.write(f"{k}:{v}\n")
for x, y in module.output_sub.items():
txt_file.write(f"{x}:{y}\n\n")
```
3. Use `Pathlib` to simplify path operation:
```
fc_result_path = Path(done_path) / vin / 'fc_result'
fc_result_path.mkdir(parents=True, exist_ok=True)
pd.DataFrame(module.output).to_csv(fc_result_path / 'main_structure.csv')
pd.DataFrame(module.output_sub).to_csv(fc_result_path / 'sub_structure.csv')
```
4. Use f-string to simplify string formatting:
```
print(f"time cost of fault classification: {(end_time - start_time) * 1000.0} ms")
```
Here's the optimized code:
```
def fault_classification_wrapper(vin, main_path, data_path, log_path, done_path):
start_time = time.time()
isc_path = Path(done_path) / vin / 'isc_cal_result' / f'{vin}_report.xlsx'
if not isc_path.exists():
print('No isc detection input!')
isc_input = pd.DataFrame()
else:
isc_input = isc_produce_alarm(isc_path, vin)
ica_path = Path(done_path) / vin / 'ica_cal_result' / f'ica_detection_alarm_{vin}.csv'
if not ica_path.exists():
print('No ica detection input!')
ica_input = pd.DataFrame()
else:
ica_input = ica_produce_alarm(ica_path)
soh_path = Path(done_path) / vin / 'SOH_cal_result' / f'{vin}_sohAno.csv'
if not soh_path.exists():
print('No soh detection input!')
soh_input = pd.DataFrame()
else:
soh_input = soh_produce_alarm(soh_path, vin)
alarm_df = pd.concat([isc_input, ica_input, soh_input]).reset_index(drop=True)
alarm_df['alarm_cell'] = alarm_df['alarm_cell'].apply(str)
print(vin)
module = AutoAnalysisMain(alarm_df, main_path, data_path, done_path)
module.analysis_process()
with open(Path(log_path) / 'log.txt', 'w') as txt_file:
for k, v in module.output.items():
txt_file.write(f"{k}:{v}\n")
for x, y in module.output_sub.items():
txt_file.write(f"{x}:{y}\n\n")
fc_result_path = Path(done_path) / vin / 'fc_result'
fc_result_path.mkdir(parents=True, exist_ok=True)
pd.DataFrame(module.output).to_csv(fc_result_path / 'main_structure.csv')
pd.DataFrame(module.output_sub).to_csv(fc_result_path / 'sub_structure.csv')
end_time = time.time()
print(f"time cost of fault classification: {(end_time - start_time) * 1000.0} ms")
return
```
相关推荐
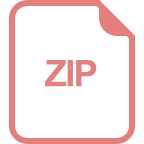
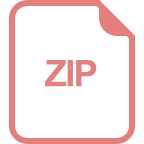
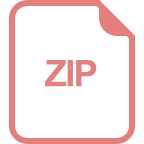













