We derive analytic bounds on the noise invariance of majority vote classifiers operating on compressed inputs. Specifically, starting from recent bounds on the true risk of majority vote classifiers, we extend the applicability of PAC-Bayesian theory to quantify the resilience of majority votes to input noise stemming from compression. The derived bounds are intuitive in binary classification settings, where they can be measured as expressions of voter differentials and voter pair agreement. By combining measures of input distortion with analytic guarantees on noise invariance, we prescribe rate-efficient machines to compress inputs without affecting subsequent classification. Our validation shows how bounding noise invariance can inform the compression stage for any majority vote classifier such that worst-case implications of bad input reconstructions are known, and inputs can be compressed to the minimum amount of information needed prior to inference.翻译
时间: 2024-03-07 21:52:31 浏览: 18
我们推导出多数投票分类器在处理压缩输入时的噪声不变性的解析界限。具体来说,从最近关于多数投票分类器真实风险的界限出发,我们扩展了PAC-Bayesian理论的适用性,以量化多数投票对来自压缩的输入噪声的抵抗能力。这些导出的界限在二元分类设置中具有直观性,可以通过选民差异和选民对一致性的表达式来衡量。通过将输入失真度量与噪声不变性的解析保证相结合,我们可以指定有效率的机器来压缩输入,而不影响后续的分类。我们的验证表明,通过界定噪声不变性,可以为任何多数投票分类器的压缩阶段提供信息,以便在知道恶劣输入重构的最坏情况下,将输入压缩到推理所需的最小信息量。
相关问题
warning: in the working copy of 'var_derive/imss/imssvar.py', LF will be replaced by CRLF the next time Git touches it
这个警告是在使用Windows平台进行git add操作时出现的。它的意思是在下一次Git操作时,LF(换行符)将被CRLF(回车换行符)替换。这个警告是由于Git的自动换行符转换功能引起的。在Windows系统上,Git可以自动将行结束符CRLF转换为LF,而在签出代码时将LF转换为CRLF。你可以通过设置core.autocrlf来控制这个功能。如果你想在签出代码时将LF转换为CRLF,可以将core.autocrlf设置为true。你可以使用以下命令进行设置:$ git config --global core.autocrlf true。如果你在Linux或Mac系统上使用LF作为行结束符,并且不希望Git在签出文件时进行自动转换,可以将core.autocrlf设置为input。这样,在提交时,Git会将CRLF转换为LF,而在签出时不进行转换。你可以使用以下命令进行设置:$ git config --global core.autocrlf input。\[1\]\[2\]\[3\]
#### 引用[.reference_title]
- *1* [warning: in the working copy of ‘package-lock.json‘, LF will be replaced by CRLF the next time Git](https://blog.csdn.net/qq_43842093/article/details/128471953)[target="_blank" data-report-click={"spm":"1018.2226.3001.9630","extra":{"utm_source":"vip_chatgpt_common_search_pc_result","utm_medium":"distribute.pc_search_result.none-task-cask-2~all~insert_cask~default-1-null.142^v91^koosearch_v1,239^v3^insert_chatgpt"}} ] [.reference_item]
- *2* *3* [[git] warning: LF will be replaced by CRLF | fatal: CRLF would be replaced by LF](https://blog.csdn.net/feng88724/article/details/11600375)[target="_blank" data-report-click={"spm":"1018.2226.3001.9630","extra":{"utm_source":"vip_chatgpt_common_search_pc_result","utm_medium":"distribute.pc_search_result.none-task-cask-2~all~insert_cask~default-1-null.142^v91^koosearch_v1,239^v3^insert_chatgpt"}} ] [.reference_item]
[ .reference_list ]
this type must derive from the 'modulerules' type defined by unreal build to
这个类型必须派生自Unreal Build Tool定义的'modulerules'类型。Unreal Build Tool(UBT)是虚幻引擎的一个构建工具,它用于编译、打包和构建游戏项目。UBT定义了许多类型和规则,用于描述和管理构建过程中的模块、插件和资源等。
'modulerules'是其中一个类型,它用于定义模块的构建规则和属性。派生自'modulerules'类型意味着这个新类型继承了'modulerules'的特性和行为,并且可以基于这个基类进行扩展和定制。
通过从'modulerules'类型派生出新的类型,开发人员可以根据特定需求定义自己的构建规则,并使用UBT的功能进行构建过程中的自定义操作。这样可以提高游戏项目的构建效率和灵活性,满足开发团队的特定需求。
派生自'modulerules'类型的新类型可以包含各种定义和配置,例如编译选项、依赖关系、插件使用、资源管理等。通过定义和修改这些属性,开发人员可以定制构建过程,优化编译速度和资源使用,以及解决特定的构建问题。
总之,派生自'modulerules'类型是在Unreal Build Tool中自定义构建规则和描述模块属性的一种方式,它可以帮助开发人员更好地管理和控制游戏项目的构建过程。
相关推荐
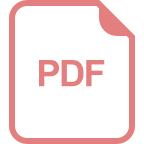
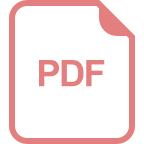
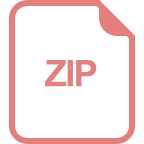












