for i, (x, label) in enumerate(self.train_loader): x = x.to(self.device) label = label.to(self.device) outputs = self.net(x)#得到新模型的输出 target_curr = label pre_ce = outputs.clone() pre_ce = pre_ce[:, self.strat_num:self.end_num] loss = torch.nn.functional.cross_entropy(pre_ce, target_curr)#新模型和目标结果的交叉熵 loss_distill = 0 if self.distillation: with torch.no_grad(): outputs_old = self.old_model(x) t_one_hot = outputs_old[:0:self.strat_num] loss_distill = F.binary_cross_entropy(F.softmax(outputs[:0:self.strat_num] / 2.0, dim=1), F.softmax(t_one_hot, dim=1)) loss = loss + 10 * loss_distill逐行解释
时间: 2024-02-10 15:15:32 浏览: 27
这段代码是一个使用知识蒸馏技术训练神经网络的实现示例,以下是逐行解释:
- `for i, (x, label) in enumerate(self.train_loader):`
- 对训练数据进行迭代,`x`是输入数据,`label`是对应的标签。
- `x = x.to(self.device)`
- 将输入数据移动到指定的设备上(如GPU)。
- `label = label.to(self.device)`
- 将标签数据移动到指定的设备上(如GPU)。
- `outputs = self.net(x)`
- 将输入数据通过新模型进行前向计算,得到输出结果。
- `target_curr = label`
- 将当前模型的输出结果作为目标结果。
- `pre_ce = outputs.clone()`
- 对当前模型的输出结果进行深拷贝,以便后续计算知识蒸馏损失。
- `pre_ce = pre_ce[:, self.strat_num:self.end_num]`
- 从当前模型的输出结果中截取出需要计算损失的部分。
- `loss = torch.nn.functional.cross_entropy(pre_ce, target_curr)`
- 计算当前模型的输出结果和目标结果之间的交叉熵损失。
- `loss_distill = 0`
- 初始化知识蒸馏损失为0。
- `if self.distillation:`
- 如果启用了知识蒸馏,则进行下列操作。
- `with torch.no_grad():`
- 关闭梯度计算。
- `outputs_old = self.old_model(x)`
- 将输入数据通过旧模型进行前向计算,得到输出结果。
- `t_one_hot = outputs_old[:0:self.strat_num]`
- 从旧模型的输出结果中截取出需要计算知识蒸馏损失的部分,并将其转化为独热编码。
- `loss_distill = F.binary_cross_entropy(F.softmax(outputs[:0:self.strat_num] / 2.0, dim=1), F.softmax(t_one_hot, dim=1))`
- 计算新模型和旧模型的输出结果之间的知识蒸馏损失,主要包括两部分:一是将新模型的输出结果和旧模型的输出结果进行softmax操作,并将结果进行二元交叉熵计算;二是将旧模型的输出结果进行softmax操作,再将其转化为独热编码。其中,`F`是PyTorch中的函数库。
- `loss = loss + 10 * loss_distill`
- 将交叉熵损失和知识蒸馏损失进行加权求和,其中10是超参数,可根据实际情况进行调整。最终得到总损失函数,用于进行反向传播更新模型参数。
相关推荐
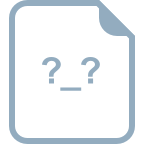















