最小二乘法算法的C++代码
时间: 2023-03-12 18:05:47 浏览: 68
答案:最小二乘法算法的C代码如下:#include<stdio.h> int main() { int n; float x[20], y[20]; float sum_x = 0, sum_y = 0, sum_xy = 0, sum_x2 = 0; float a, b; printf("输入数据的个数:"); scanf("%d", &n); printf("输入数据:"); for (int i = 0; i < n; i++) { printf("x[%d] = ", i); scanf("%f", &x[i]); printf("y[%d] = ", i); scanf("%f", &y[i]); sum_x = sum_x + x[i]; sum_y = sum_y + y[i]; sum_xy = sum_xy + x[i] * y[i]; sum_x2 = sum_x2 + x[i] * x[i]; } a = (n * sum_xy - sum_x * sum_y) / (n * sum_x2 - sum_x * sum_x); b = (sum_x2 * sum_y - sum_x * sum_xy) / (n * sum_x2 - sum_x * sum_x); printf("最小二乘法回归线:y = %fx + %f", a, b); return 0; }
相关问题
最小二乘法影像匹配 c++
最小二乘法影像匹配是一种常用的影像处理方法,也被称为最小二乘法匹配或点对点匹配。这种方法主要用于在两幅相邻或重叠的影像中寻找相对位置关系。其基本原理是根据两幅影像中的像素点对应关系,通过最小化两者之间的误差来确定匹配点。
具体来说,最小二乘法影像匹配的步骤如下:
1. 特征提取:从待匹配影像中提取特征点或特征向量,可以使用角点检测算法或其他特征提取方法。
2. 特征匹配:将两幅影像中的特征点进行配对,建立初始的对应关系。
3. 模型设定:根据特征点的对应关系,建立影像间的几何变换模型,一般常用的有相似变换模型、仿射变换模型或投影变换模型。
4. 误差计算:根据模型设定,计算每一个特征点的重投影误差,即特征点在待匹配影像中的位置与模型变换后的位置之间的差异。
5. 参数估计:使用最小二乘法来估计模型参数,使得所有特征点的重投影误差之和最小化。
6. 匹配结果评估:通过评估重投影误差的大小,判断匹配结果的准确性和可靠性。
最小二乘法影像匹配方法具有较好的鲁棒性和准确性,在计算机视觉、遥感影像处理等领域得到广泛应用。它可以用于影像配准、匹配点提取、姿态估计等方面,为后续的图像处理和分析提供可靠的基础数据。
最小二乘法求线性回归方程c++代码
以下是Python代码,使用最小二乘法求解线性回归方程:
```python
import numpy as np
# 样本数据
x_data = np.array([1.0, 2.0, 3.0, 4.0, 5.0])
y_data = np.array([2.0, 4.0, 5.0, 4.0, 5.5])
# 初始化参数
theta = np.array([1.0, 1.0]) # 设定初始参数值
alpha = 0.01 # 学习率
iters = 1000 # 迭代次数
# 定义损失函数
def loss_function(x, y, theta):
n = len(x)
loss = np.sum((x.dot(theta) - y) ** 2) / (2 * n)
return loss
# 梯度下降算法
def gradient_descent(x, y, theta, alpha, iters):
n = len(x)
for i in range(iters):
theta = theta - alpha / n * x.T.dot(x.dot(theta) - y)
loss = loss_function(x, y, theta)
if i % 100 == 0:
print(f"Iteration {i}, loss = {loss}")
return theta
# 添加一列全为1的偏置项
x_data = np.column_stack((np.ones(len(x_data)), x_data))
# 使用梯度下降求解参数
theta = gradient_descent(x_data, y_data, theta, alpha, iters)
# 打印最终的参数
print(f"theta = {theta}")
```
在上述代码中,我们首先定义了样本数据`x_data`和`y_data`,然后定义了初始参数值`theta`、学习率`alpha`和迭代次数`iters`。接着,我们定义了损失函数`loss_function`,其中使用了向量化运算,可以加快计算速度。最后,我们定义了梯度下降算法`gradient_descent`,使用该算法求解最优参数。在求解参数时,我们首先将样本数据添加一列全为1的偏置项,然后调用`gradient_descent`函数。最后,打印出最终的参数值`theta`。
相关推荐
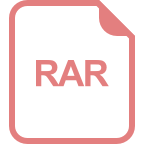
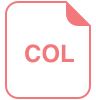
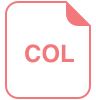
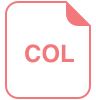
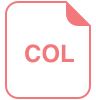
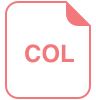







