from_logits=True
时间: 2023-09-30 13:05:33 浏览: 42
from_logits=True通常是指在使用交叉熵损失函数时,将模型输出的原始值(logits)作为输入,而不是经过softmax转换后的概率值。在深度学习中,交叉熵损失函数通常用于多分类任务中,其计算公式如下:
$$
loss = -\frac{1}{N} \sum_{i=1}^{N} \sum_{j=1}^{K} y_{i,j} \log(p_{i,j})
$$
其中,$N$为样本数,$K$为类别数,$y_{i,j}$表示第$i$个样本的第$j$个类别是否为正确标签(0或1),$p_{i,j}$表示第$i$个样本的第$j$个类别的预测概率。在实际实现中,我们可以将模型的输出先经过softmax函数将其转换为概率值,然后再将概率值作为损失函数的输入进行计算。也可以将模型输出的原始值作为输入,将from_logits参数设置为True,让交叉熵函数内部进行softmax转换。这种方法可以提高数值稳定性,避免softmax函数输出值过小或者过大,从而导致计算精度下降。因此,当模型输出的是原始值而不是概率值时,通常将from_logits参数设置为True。
相关问题
按照以下要求补充代码:The final Dense layer should use a 'linear' activation. This is effectively no activation. The model.compile statement will indicate this by including from_logits=True. loss=tf.keras.losses.SparseCategoricalCrossentropy(from_logits=True) This does not impact the form of the target. In the case of SparseCategorialCrossentropy, the target is the expected digit, 0-9.
要按照要求修改代码,可以将第三个Dense的激活函数改为线性激活函数('linear' activation)。同时,在model.compile语句中from_logits=True参数,以指示使用性激活函数。的代码如下所:
```python
tf.random.set_seed1234) # 为了结果的一致性
model = Sequential(
[
tf.keras.layers.Dense(units=25, activation="relu"),
tf.keras.layers.Dense(units=15, activation="relu"),
tf.keras.layers.Dense(units=10, activation="linear") # 修改激活函数为线性激活函数
], name="my_model"
)
model.compile(optimizer=tf.keras.optimizers.Adam(),
loss=tf.keras.losses.SparseCategoricalCrossentropy(from_logits=True), # 添加from_logits=True参数
metrics=['accuracy'])
```
这样修改后的模型使用线性激活函数作为最后一个Dense层的激活函数,并且通过设置from_logits=True来指示SparseCategoricalCrossentropy损失函数。这种设置不会影响目标的形式,目标仍然是期望的数字0-9。
import tensorflow as tf from tensorflow.keras import datasets, layers, models, optimizers from tensorflow.keras.preprocessing import image_dataset_from_directory import matplotlib.pyplot as plt # 定义数据集路径 data_dir = r'F:\Pycham\project\data\FMD' # 定义图像大小和批处理大小 image_size = (224, 224) batch_size = 32 # 从目录中加载训练数据集 train_ds = image_dataset_from_directory( data_dir, validation_split=0.2, subset="training", seed=123, image_size=image_size, batch_size=batch_size) # 从目录中加载验证数据集 val_ds = image_dataset_from_directory( data_dir, validation_split=0.2, subset="validation", seed=123, image_size=image_size, batch_size=batch_size) # 构建卷积神经网络模型 model = models.Sequential() model.add(layers.experimental.preprocessing.Rescaling(1./255, input_shape=(image_size[0], image_size[1], 3))) model.add(layers.Conv2D(32, (3, 3), activation='selu')) model.add(layers.MaxPooling2D((2, 2))) model.add(layers.Conv2D(64, (3, 3), activation='selu')) model.add(layers.MaxPooling2D((2, 2))) model.add(layers.Conv2D(64, (3, 3), activation='selu')) model.add(layers.Conv2D(128, (3, 3), activation='selu')) model.add(layers.MaxPooling2D((2, 2))) # 添加全连接层 model.add(layers.Flatten()) model.add(layers.Dense(128, activation='selu')) model.add(layers.Dropout(0.5)) model.add(layers.Dense(64, activation='selu')) model.add(layers.Dense(10)) # 编译模型,使用 SGD 优化器和 Categorical Crossentropy 损失函数 model.compile(optimizer=optimizers.SGD(learning_rate=0.01, momentum=0.9), loss=tf.keras.losses.SparseCategoricalCrossentropy(from_logits=True), metrics=['accuracy']) # 训练模型,共训练 20 轮 history = model.fit(train_ds, epochs=5, validation_data=val_ds) # 绘制训练过程中的准确率和损失曲线 plt.plot(history.history['accuracy'], label='accuracy') plt.plot(history.history['val_accuracy'], label = 'val_accuracy') plt.xlabel('Epoch') plt.ylabel('Accuracy') plt.ylim([0.5, 1]) plt.legend(loc='lower right') plt.show() # 在测试集上评估模型准确率 test_loss, test_acc = model.evaluate(val_ds) print(f'测试准确率: {test_acc}')上述代码得出的准确率仅为0.5,请你通过修改学习率等方式修改代码,假设数据集路径为F:\Pycham\project\data\FMD
如果准确率较低,可以通过以下方式尝试提高准确率:
1. 调整学习率:学习率是一个重要的超参数,可以尝试减小或增大学习率,以找到一个更好的值。例如,尝试将学习率从0.01调整为0.005或0.02。
2. 增加训练轮数:训练模型的轮数越多,模型就有越多的机会学习数据集中的模式。尝试增加训练轮数,以提高模型准确率。
3. 调整网络结构:可以尝试增加或减少卷积层、全连接层或dropout层等,以找到更好的网络结构。例如,增加卷积层或全连接层的数量,或者尝试使用其他激活函数。
4. 数据增强:数据增强是一种有效的方式,可以增加数据集的多样性,并提高模型的泛化能力。可以尝试使用不同的数据增强技术,例如旋转、平移、缩放、翻转等。
以下是修改后的代码,调整了学习率和训练轮数,并增加了数据增强:
```python
import tensorflow as tf
from tensorflow.keras import datasets, layers, models, optimizers
from tensorflow.keras.preprocessing.image import ImageDataGenerator
import matplotlib.pyplot as plt
# 定义数据集路径和图像大小
data_dir = r'F:\Pycham\project\data\FMD'
image_size = (224, 224)
batch_size = 32
# 使用数据增强
train_datagen = ImageDataGenerator(rotation_range=10,
width_shift_range=0.1,
height_shift_range=0.1,
zoom_range=0.1,
horizontal_flip=True,
rescale=1./255)
# 从目录中加载训练数据集
train_ds = train_datagen.flow_from_directory(data_dir,
target_size=image_size,
batch_size=batch_size,
class_mode='categorical')
# 从目录中加载验证数据集
val_ds = image_dataset_from_directory(data_dir,
validation_split=0.2,
subset="validation",
seed=123,
image_size=image_size,
batch_size=batch_size)
# 构建卷积神经网络模型
model = models.Sequential()
model.add(layers.experimental.preprocessing.Rescaling(1./255, input_shape=(image_size[0], image_size[1], 3)))
model.add(layers.Conv2D(32, (3, 3), activation='selu'))
model.add(layers.MaxPooling2D((2, 2)))
model.add(layers.Conv2D(64, (3, 3), activation='selu'))
model.add(layers.MaxPooling2D((2, 2)))
model.add(layers.Conv2D(64, (3, 3), activation='selu'))
model.add(layers.Conv2D(128, (3, 3), activation='selu'))
model.add(layers.MaxPooling2D((2, 2)))
# 添加全连接层
model.add(layers.Flatten())
model.add(layers.Dense(128, activation='selu'))
model.add(layers.Dropout(0.5))
model.add(layers.Dense(64, activation='selu'))
model.add(layers.Dense(train_ds.num_classes))
# 编译模型,使用 Adam 优化器和 Categorical Crossentropy 损失函数
model.compile(optimizer=optimizers.Adam(learning_rate=0.001),
loss=tf.keras.losses.CategoricalCrossentropy(from_logits=True),
metrics=['accuracy'])
# 训练模型,共训练 20 轮
history = model.fit(train_ds, epochs=20, validation_data=val_ds)
# 绘制训练过程中的准确率和损失曲线
plt.plot(history.history['accuracy'], label='accuracy')
plt.plot(history.history['val_accuracy'], label = 'val_accuracy')
plt.xlabel('Epoch')
plt.ylabel('Accuracy')
plt.ylim([0.5, 1])
plt.legend(loc='lower right')
plt.show()
# 在测试集上评估模型准确率
test_loss, test_acc = model.evaluate(val_ds)
print(f'测试准确率: {test_acc}')
```
在代码中,使用了ImageDataGenerator进行数据增强,并将Adam优化器用于模型的编译。另外,将训练轮数增加到了20轮。
相关推荐
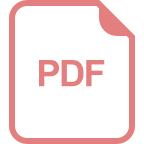
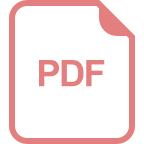
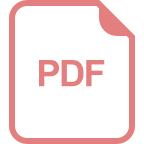













