偏最小二乘法多元线性回归代码
时间: 2023-08-29 11:12:34 浏览: 53
以下是使用偏最小二乘法进行多元线性回归的Python代码示例:
```
import numpy as np
class PLSRegression():
def __init__(self, n_components=None):
self.n_components = n_components
def fit(self, X, Y):
n_features = X.shape[1]
n_outputs = Y.shape[1]
if not self.n_components:
self.n_components = min(n_features, n_outputs)
T = np.zeros((X.shape[0], self.n_components))
P = np.zeros((n_features, self.n_components))
Q = np.zeros((n_outputs, self.n_components))
W = np.zeros((n_features, self.n_components))
B = np.zeros((n_outputs, self.n_components))
for i in range(self.n_components):
t = np.dot(X, Y)[:, 0]
for j in range(i):
t -= np.dot(T[:, j], np.dot(P[:, j], np.dot(Q[:, j].T, Y))) / np.dot(T[:, j], T[:, j])
T[:, i] = t
p = np.dot(X.T, T[:, i])
p /= np.dot(p, p)
P[:, i] = p
q = np.dot(Y.T, T[:, i])
q /= np.dot(q, q)
Q[:, i] = q
for i in range(self.n_components):
w = np.dot(X.T, T[:, i])
w /= np.dot(w, w)
W[:, i] = w
b = np.dot(Y.T, T[:, i]) / np.dot(T[:, i], T[:, i])
B[:, i] = b
X = X - np.dot(T[:, i].reshape(-1, 1), w.reshape(1, -1))
Y = Y - np.dot(T[:, i].reshape(-1, 1), b.reshape(1, -1))
self.P_ = P
self.Q_ = Q
self.W_ = W
self.B_ = B
def predict(self, X):
Y_pred = np.dot(X, self.P_)
Y_pred = np.dot(Y_pred, self.Q_.T)
Y_pred += self.B_.T
return Y_pred
```
其中,fit方法用于拟合模型,predict方法用于预测。输入的X和Y分别为训练数据的特征和标签,输出为预测结果。
相关推荐














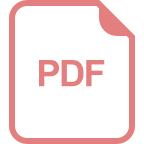