使用python实现逻辑回归函数
时间: 2023-10-31 13:08:44 浏览: 67
Sure, no problem! Here is an implementation of the logistic regression function in Python:
```
import numpy as np
def sigmoid(z):
return 1 / (1 + np.exp(-z))
class LogisticRegression:
def __init__(self, lr=0.01, num_iter=100000, fit_intercept=True, verbose=False):
self.lr = lr
self.num_iter = num_iter
self.fit_intercept = fit_intercept
self.verbose = verbose
def add_intercept(self, X):
intercept = np.ones((X.shape[0], 1))
return np.concatenate((intercept, X), axis=1)
def fit(self, X, y):
if self.fit_intercept:
X = self.add_intercept(X)
# initialize weights
self.theta = np.zeros(X.shape[1])
# gradient descent
for i in range(self.num_iter):
z = np.dot(X, self.theta)
h = sigmoid(z)
gradient = np.dot(X.T, (h - y)) / y.size
self.theta -= self.lr * gradient
if self.verbose and i % 10000 == 0:
z = np.dot(X, self.theta)
h = sigmoid(z)
print(f'loss: {self._loss(h, y)} \t')
def predict_prob(self, X):
if self.fit_intercept:
X = self.add_intercept(X)
return sigmoid(np.dot(X, self.theta))
def predict(self, X, threshold=0.5):
return self.predict_prob(X) >= threshold
def _loss(self, h, y):
return (-y * np.log(h) - (1 - y) * np.log(1 - h)).mean()
```
This implementation includes a `sigmoid` function, which is used to calculate the probability of the target variable being 1, given the input features. The `LogisticRegression` class fits the logistic regression model using gradient descent, and includes methods for predicting probabilities and binary classifications. If `fit_intercept` is set to `True`, the model will also fit an intercept term to the data.
阅读全文
相关推荐
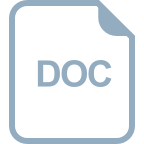
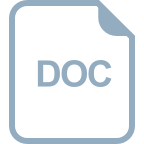
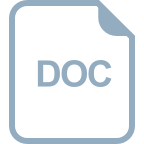
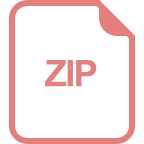
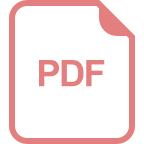
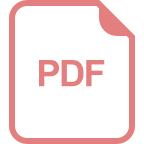
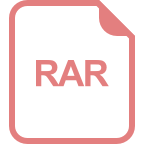
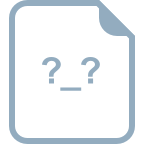
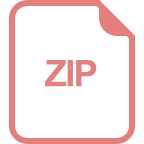
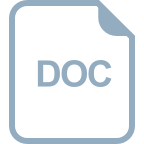
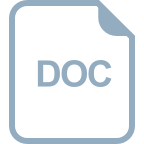
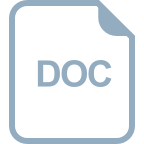
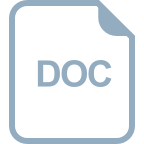
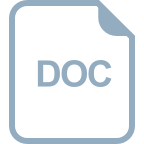
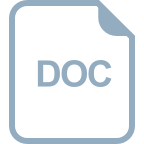
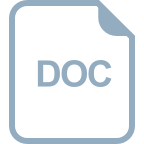
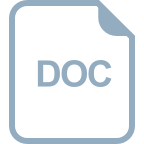
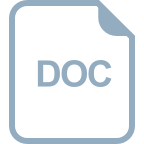
