编写一个python代码,基于3D UNet,输入标签得到剂量预测
时间: 2024-10-05 11:04:59 浏览: 37
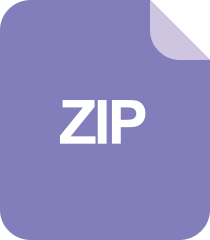
基于Unet实现的心脏分割任务python源码+模型(高分项目).zip

要在Python中编写一个基于3D UNet的图像分割模型来预测剂量,首先你需要安装一些必要的库,比如`torch`, `pytorch-ignite`, `numpy`, 和`torchvision`(对于数据处理)。以下是构建基本框架的一个例子,假设你已经有了训练数据集和相应的标签:
```python
import torch
import torch.nn as nn
from torch.utils.data import DataLoader
from torchvision.transforms import Compose, ToTensor
import numpy as np
from unet_3d import UNet3D
# 设定超参数
batch_size = 8
num_workers = 4
learning_rate = 0.001
num_epochs = 100
# 加载并预处理数据
data_transforms = Compose([ToTensor()])
dataset = YourDataset(root_path, transform=data_transforms)
dataloader = DataLoader(dataset, batch_size=batch_size, shuffle=True, num_workers=num_workers)
# 定义3D UNet模型
class MyUNet(nn.Module):
def __init__(self):
super(MyUNet, self).__init__()
self.unet = UNet3D(input_channels=1, output_channels=1) # 输入通道数为1(灰度图像),输出通道数也为1(单通道预测)
model = MyUNet()
# 损失函数和优化器
criterion = nn.MSELoss() # 使用均方误差作为损失函数
optimizer = torch.optim.Adam(model.parameters(), lr=learning_rate)
for epoch in range(num_epochs):
for i, (inputs, targets) in enumerate(dataloader):
inputs = inputs.type(torch.float32).cuda() if torch.cuda.is_available() else inputs
targets = targets.type(torch.float32).cuda() if torch.cuda.is_available() else targets
optimizer.zero_grad()
outputs = model(inputs)
loss = criterion(outputs, targets)
loss.backward()
optimizer.step()
print(f"Epoch [{epoch+1}/{num_epochs}], Step [{i+1}/{len(dataloader)}], Loss: {loss.item():.4f}")
# 预测阶段
# 假设我们有一个新的未标记图像
new_data = ... # 根据实际加载新图像
prediction = model(new_data.unsqueeze(0)) # 将数据添加维度以便模型接受
prediction = prediction.squeeze().detach().cpu().numpy()
```
请注意,这个代码片段是一个简化的版本,实际使用时需要根据你的数据集(例如 `.nii.gz` 或者 `.h5` 文件格式)调整数据读取部分,并且可能需要进行数据增强和验证等步骤。同时,`YourDataset` 应该是你自定义的包含输入图像和标签的类。
阅读全文
相关推荐
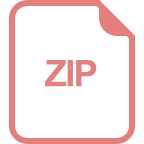
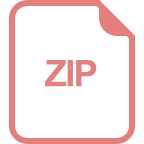
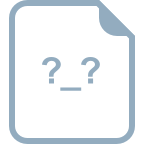
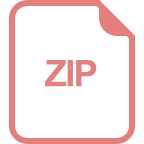







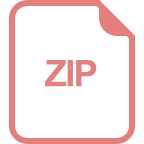
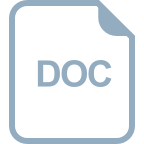



