Matrix Decomposition Methods in Numerical Linear Algebra
发布时间: 2024-09-14 23:07:44 阅读量: 29 订阅数: 15 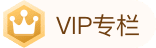
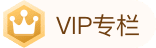
# 1. Introduction
## 1.1 A Brief Introduction to Linear Algebra
Linear algebra stands as a pivotal branch of mathematics, playing a significant role across various disciplines. It investigates the properties of vector spaces and linear mappings and serves as one of the foundational tools in modern mathematics and scientific fields.
The fundamental concepts of linear algebra encompass vectors, matrices, systems of linear equations, and linear transformations. Vectors represent both magnitude and direction of physical quantities mathematically, matrices are rectangular arrays arranged with elements in a specific order, systems of linear equations consist of multiple linear equations, and linear transformations refer to mappings from one vector space to another.
## 1.2 The Importance and Applications of Matrices
Matrices find extensive applications in a multitude of domains. In physics, matrices are used to describe transformations, rotations, scaling, and other movements of objects, aiding in the calculation of motion trajectories and deformations for particles and rigid bodies in space. In economics, matrices represent the input-output relationships of economic systems, facilitating studies in economic growth and resource allocation. In computer science, matrices are pivotal in image processing, machine learning, and data compression, among other areas.
## 1.3 Background and Significance of Matrix Factorization Methods
Matrix factorization involves expressing a complex matrix as a product of simpler matrices, enhancing our comprehension of matrix properties and applications. Matrix factorization methods are crucial in numerical linear algebra, with implications for solving systems of linear equations, computing eigenvalues and eigenvectors, data compression, and dimensionality reduction.
This article aims to introduce commonly used matrix factorization methods in numerical linear algebra, including basic matrix factorization, eigenvalue decomposition, singular value decomposition (SVD), generalized eigenvalue decomposition, and advanced matrix factorization techniques. By learning and understanding these methods, we can better apply linear algebra knowledge to practical problems, improving computational efficiency and accuracy.
# 2. Basic Matrix Factorization Methods
### 2.1 LU Decomposition
LU decomposition is a commonly used matrix factorization technique applied to solving systems of linear equations and inverting matrices. The fundamental principle is to factorize a matrix into the product of a lower triangular matrix and an upper triangular matrix. The specific steps are as follows:
1. For an n阶 square matrix A, select an n阶 unit lower triangular matrix L and an n阶 upper triangular matrix U.
2. Transform A into an upper triangular matrix U through row operations, recording each step of the row operations to obtain matrix U.
3. Apply the recorded row operations in reverse to the identity matrix to obtain the lower triangular matrix L.
4. Factorize matrix A into the product of L and U: A = LU.
The advantages of LU decomposition include reducing the computational effort for solving systems of linear equations and inverting matrices, making it especially suitable for solving systems with identical coefficient matrices multiple times.
Here is an example code for LU decomposition using Python:
```python
import numpy as np
def lu_decomposition(A):
n = len(A)
L = np.eye(n)
U = np.copy(A)
for k in range(n-1):
for i in range(k+1, n):
factor = U[i, k] / U[k, k]
L[i, k] = factor
U[i, k:] -= factor * U[k, k:]
return L, U
# Example usage
A = np.array([[2, 3, 1],
[4, 9, 2],
[7, 8, 6]])
L, U = lu_decomposition(A)
print("Lower triangular matrix L:")
print(L)
print("Upper triangular matrix U:")
print(U)
```
**Code Explanation:**
Firstly, import the necessary library (numpy). Then, define a function named `lu_decomposition`, which takes a matrix A as input and returns the lower triangular matrix L and the upper triangular matrix U after LU decomposition. The function uses two nested loops to compute the LU decomposition, with the outer loop controlling the columns and the inner loop performing the row operations. Finally, by calling the function with the example matrix A, the result of the LU decomposition can be obtained and printed.
Running the above code will output the lower triangular matrix L and the upper triangular matrix U after LU decomposition.
### 2.2 QR Decomposition
QR decomposition involves factorizing a matrix into the product of an orthogonal matrix and an upper triangular matrix. QR decomposition is crucial in numerical computation and can be used for solving least squares problems, eigenvalue problems, and singular value decomposition. The basic principle of QR decomposition is to use the Gram-Schmidt orthogonalization process to transform the matrix column vectors into orthogonal vectors, then construct an upper triangular matrix.
Here is an example code for QR decomposition using Python:
```python
import numpy as np
def qr_decomposition(A):
Q, R = np.linalg.qr(A)
return Q, R
# Example usage
A = np.array([[2, 3, 1],
[4, 9, 2],
[7, 8, 6]])
Q, R = qr_decomposition(A)
print("Orthogonal matrix Q:")
print(Q)
print("Upper triangular matrix R:")
print(R)
```
**Code Explanation:**
In this example, we utilize the `numpy.linalg.qr` function from the numpy library to implement QR decomposition. The `qr_decomposition` function takes a matrix A as input and returns the orthogonal matrix Q and the upper triangular matrix R. By calling the function with the example matrix A, the result of the QR decomposition can be obtained and printed.
Running the above code will output the orthogonal matrix Q and the upper triangular matrix R after QR decomposition.
### 2.3 Cholesky Decomposition
Cholesky decomposition is a method for factorizing a symmetric positive-definite matrix into the product of a lower triangular matrix and its transpose. Cholesky decomposition is useful for efficiently solving systems of linear equations and generating random numbers.
Here is an example code for Cholesky decomposition using Python:
```python
import numpy as np
def cholesky_decomposition(A):
n = len(A)
L = np.zeros_like(A)
for i in range(n):
for j in range(i+1):
if i == j:
L[i, j] = np.sqrt(A[i, j] - np.sum(L[i, :j]**2))
else:
L[i, j] = (A[i, j] - np.sum(L[i, :j] * L[j, :j])) / L[j, j]
return L
# Example usage
A = np.array([[4, 12, -16],
[12, 37, -43],
[-16, -43, 98]])
L = cholesky_decomposition(A)
print("Lower triangular matrix L:")
print(L)
```
**Code Explanation:**
In this example, we use the `numpy.sqrt` function and `numpy.sum` function from the numpy library to calculate each element of the Cholesky decomposition. The `cholesky_decomposition` function takes a symmetric positive-definite matrix A as input and returns the lower triangular matrix L. By calling the function with the example matrix A, the result of the Cholesky decomposition can be obtained and printed.
Running the above code will output the lower triangular matrix L after Cholesky decomposition.
**Summary:**
This chapter introduces basic matrix factorization methods in numerical linear algebra, including LU decomposition, QR decomposition, and Cholesky decomposition. These methods are significantly applied in solving systems of linear equations, inverting matrices, and solving least squares problems. By understanding and mastering these basic matrix factorization methods, they can be better applied to numerical computation in practical problems.
# 3. Eigenvalue Decomposition Methods
This chapter will introduce eigenvalue decomposition meth
0
0
相关推荐
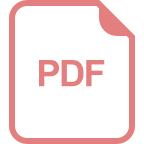
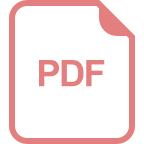
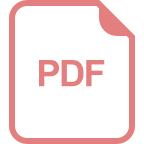
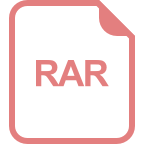
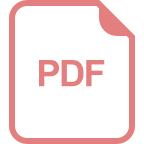
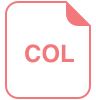
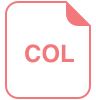
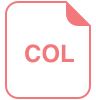
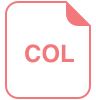