Introduction to Frequency Domain Analysis: Concepts and Basic Principles
发布时间: 2024-09-15 05:27:01 阅读量: 35 订阅数: 35 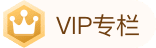
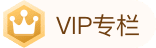
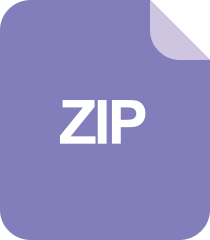
白色大气风格的旅游酒店企业网站模板.zip
# Chapter 1: Introduction to Frequency Domain Analysis
Frequency domain analysis is a signal processing method that studies the frequency components and characteristics of a signal by transforming it from the time domain to the frequency domain. In frequency domain analysis, signals are represented by amplitude and phase information at different frequencies, which helps us understand the signal's features and behavior more profoundly. This chapter will introduce the basic concepts and applications of frequency domain analysis, and explore the differences between frequency domain analysis and time domain analysis.
# Chapter 2: Basic Concepts of Fourier Transform
The Fourier transform is a significant mathematical tool in the field of signal processing. By transforming signals from the time domain to the frequency domain, it helps us better understand the characteristics and behavior of signals. In this chapter, we will introduce the basic concepts of the Fourier transform, including its definition, its relationship with the Fourier series, and the differences between the Fourier transforms of continuous and discrete signals.
### 2.1 Definition of Fourier Transform
The Fourier transform represents a function as the sum of sine and cosine functions at different frequencies. For a continuous function \(x(t)\), its Fourier transform \(X(f)\) is defined as follows:
\[
X(f) = \int_{-\infty}^{\infty} x(t) e^{-j2\pi ft} dt
\]
Here, \(f\) is the frequency, and \(j\) is the imaginary unit. The Fourier transform converts a signal from the time domain (\(t\)) to the frequency domain (\(f\)), obtaining the signal's representation in the frequency domain.
### 2.2 Relationship Between Fourier Series and Fourier Transform
The Fourier series is a frequency domain representation method for periodic signals, while the Fourier transform is for non-periodic signals. The Fourier series decomposes a periodic signal into a series of sine and cosine functions, whereas the Fourier transform represents a non-periodic signal as a continuous spectrum.
### 2.3 Differences Between Fourier Transform of Continuous Signals and Discrete Signals
In practical applications, we have both continuous and discrete signals, corresponding to the continuous Fourier transform and discrete Fourier transform. The continuous Fourier transform is a transformation of the signal over the entire time range, while the discrete Fourier transform is a transformation of the signal within a finite time and frequency range. The discrete Fourier transform is widely used in digital signal processing, such as in the design of digital filters and spectral analysis.
Through the study of this chapter, we have a preliminary understanding of the basic concepts of the Fourier transform and its importance in signal processing. In the following chapters, we will continue to explore important concepts and applications in frequency domain analysis.
# Chapter 3: Important Concepts in Frequency Domain Analysis
Frequency domain analysis involves some important concepts. Deep understanding of these concepts is crucial for frequency domain analysis. The following will detail three important concepts in frequency domain analysis.
#### 3.1 Spectral Density and Power Spectral Density
Spectral density refers to the energy distribution of a signal in the frequency domain, describing the intensity of the signal at different frequencies. Power spectral density is the square of the signal's spectral density, indicating the signal power within a unit frequency range. Spectral density and power spectral density are widely used in signal processing to analyze the frequency domain characteristics of signals.
#### 3.2 Frequency Resolution
Frequency resolution refers to the ability of a system or method to distinguish different frequency components in a signal. In frequency domain analysis, the size of the frequency resolution directly affects the accuracy of signal processing. Generally, the higher the frequency resolution, the more accurately the system can distinguish different frequency components of the signal.
#### 3.3 Phase Spectrum Analysis
Phase spectrum analysis refers to the analysis of the phase information of a signal in the frequency domain. Phase information is also crucial in signal processing, as it can affect the waveform and characteristics of the signal. Through phase spectrum analysis, we can gain a deeper understanding of the phase variation规律of the signal in the frequency domain, providing more information and basis for signal processing.
These are the important concepts in frequency domain analysis. A deep understanding of these concepts will help us better apply frequency domain analysis technology. Next, we will introduce some scenarios and cases of frequency domain analysis in practical applications.
# Chapter 4: Applications of Fourier Transform in Signal Processing
The Fourier transform plays a vital role in signal processing. Through frequency domain analysis, signals can be filtered, and spectral analysis can be performed. The following are specific applications of the Fourier transform in signal processing:
#### 4.1 Signal Filtering and Frequency Domain Analysis
In signal processing, filtering is a common operation used to remove noise from a signal or select interesting frequency components. Through the Fourier transform, we can transform a signal from the time domain to the frequency domain, filter the frequency domain signal, and then inverse transform it back to the time domain to obtain the filtered signal.
```python
import numpy as np
import matplotlib.pyplot as plt
# Generate random signal
np.random.seed(0)
time = np.linspace(0, 10, 1000)
signal = np.cos(2 * np.pi * 1 * time) + 0.5 * np.cos(2 * np.pi * 2.5 * time)
noise = 0.2 * np.random.normal(size=time.size)
noisy_signal = signal + noise
# Fourier Transform
fourier_transform = np.fft.fft(noisy_signal)
frequencies = np.fft.fftfreq(len(time))
# Frequency filtering
fourier_transform[np.abs(frequencies) > 2] = 0
# Inverse Transform
filtered_signal = np.fft.ifft(fourier_transform)
# Plot the results
plt.figure(figsize=(12, 6))
plt.subplot(211)
plt.plot(time, noisy_signal, label='Noisy Signal')
plt.legend()
plt.subplot(212)
plt.plot(time, filtered_signal, label='Filtered Signal', color='orange')
plt.legend()
plt.show()
```
With the above code, we can perform frequency domain filtering on a noisy signal, remove interference components, and obtain a clearer signal.
#### 4.2 The Role of Fourier Transform in Spectral Analysis
Spectral analysis is a common method used to study the frequency domain characteristics of signals. The Fourier transform can transform signals from the time domain to the frequency domain, helping us analyze the frequency components and power distribution of signals, thereby gaining a deeper understanding of the signal's characteristics.
```python
import numpy as np
import matplotlib.pyplot as plt
# Generate signal
fs = 1000 # Sampling frequency
t = np.linspace(0, 1, fs)
signal = np.sin(2 * np.pi * 5 * t) + 0.5 * np.sin(2 * np.pi * 20 * t)
fft_result = np.fft.fft(signal)
frequencies = np.fft.fftfreq(len(t), 1/fs)
# Plot power spectral density graph
plt.figure(figsize=(12, 6))
plt.plot(frequencies[:len(frequencies)//2], np.abs(fft_result)[:len(frequencies)//2])
plt.xlabel('Frequency (Hz)')
plt.ylabel('Power')
plt.title('Power Spectrum Density')
plt.show()
```
The above code is used to plot the power spectral density graph of a signal. By transforming the signal into the frequency domain through the Fourier transform, we can clearly display the frequency components and power distribution of the signal.
#### 4.3 Complementing Understanding of Frequency Domain Analysis
The Fourier transform provides us with a way to transform from the time domain to the frequency domain, allowing us to more comprehensively analyze the characteristics of signals. Frequency domain analysis, through processing signals in the frequency domain, helps us better understand the signal's frequency components, amplitude, phase, etc., providing strong support for signal processing and identification. In practical applications, combining the Fourier transform and related technologies can achieve efficient processing and analysis of signals.
# Chapter 5: Fast Fourier Transform (FFT) Algorithm
The Fast Fourier Transform (FFT) algorithm is an efficient method for computing the Fourier transform, widely used in signal processing, image processing, communications, and other fields. In frequency domain analysis, the FFT algorithm plays an important role, enabling the rapid calculation of signal spectra and achieving efficient frequency domain analysis.
#### 5.1 Introduction to the FFT Algorithm
The FFT algorithm was proposed by Cooley and Tukey in 1965. It decomposes a signal of length N into smaller subproblems and recursively calculates the Fourier transform using a divide-and-conquer strategy, thus achieving efficient computation of the Fourier transform. The time complexity of the FFT algorithm is O(NlogN), which is much better than the time complexity of O(N^2) for direct computation.
#### 5.2 Applications of FFT in Signal Processing
FFT is widely used in the field of signal processing. For example, in audio processing, FFT can quickly calculate the spectrum of an audio signal, thus enabling the extraction and analysis of audio frequency domain features; in the field of communications, FFT is used for frequency domain signal processing and modulation-demodulation; in image processing, FFT can be used for image frequency domain filtering and feature extraction applications.
#### 5.3 Comparison of FFT with Other Frequency Domain Analysis Methods
Compared with other frequency domain analysis methods, FFT has the advantages of efficiency and speed, ***pared with traditional Fourier transform methods, FFT can calculate the signal's frequency spectrum information more quickly, providing convenience for real-time signal processing. In practical applications, according to different needs and computational resources, an appropriate frequency domain analysis method can be selected for application.
Through a deep understanding and application of the FFT algorithm, we can better achieve signal processing, frequency domain analysis, and related research work, providing strong support for scientific research and engineering practice.
# Chapter 6: Future Development Directions of Frequency Domain Analysis
As an important signal processing method, frequency domain analysis has broad prospects for future development. The following are the future development directions of frequency domain analysis:
### 6.1 Applications of Artificial Intelligence in Frequency Domain Analysis
With the continuous development of artificial intelligence technology, its applications in frequency domain analysis will become increasingly widespread. Artificial intelligence can help optimize frequency domain analysis algorithms, improving processing efficiency and accuracy. For example, using deep learning algorithms to classify and predict frequency domain analysis results can help identify patterns and rules in complex signals.
### 6.2 Combination of Frequency Domain Analysis and Big Data
With the continuous popularization and application of big data technology, frequency domain analysis can also be combined with big data technology to better process massive data and complex signals. Utilizing the distributed computing and storage capabilities of big data platforms can achieve real-time analysis and processing of large-scale signal data, providing more possibilities for frequency domain analysis.
### 6.3 Development Prospects of Frequency Domain Analysis in Emerging Technology Fields
With the continuous progress of science and technology, the demand for frequency domain analysis in emerging technology fields is also increasing. For example, in the fields of the Internet of Things, artificial intelligence, and biomedical engineering, frequency domain analysis can help solve various practical problems, promoting technological development and innovation. In the future, frequency domain analysis will play a more important role in emerging technology fields, contributing to social progress and human well-being.
These are the future development directions of frequency domain analysis, hoping to bring new insights and opportunities for research and application in related fields.
0
0
相关推荐
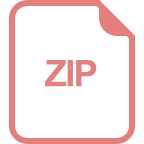
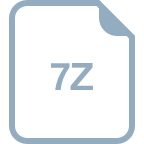
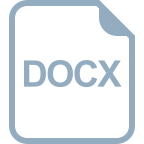
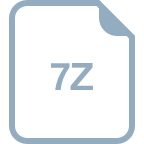