MATLAB Normal Distribution Parameter Estimation: Unveiling the Distribution Patterns Behind the Data
发布时间: 2024-09-14 15:15:56 阅读量: 26 订阅数: 29 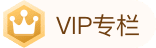
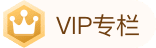
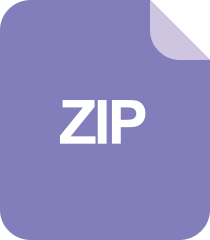
LES PHASOR PARAMETER ESTIMATION:使用LES方法估计相量参数-matlab开发
# Introduction to Normal Distribution in MATLAB
The normal distribution, also known as the Gaussian distribution, is a continuous probability distribution widely used in statistics and probability theory. It is renowned for its bell-shaped curve, characterized by two parameters: the mean and the standard deviation.
In MATLAB, the normal distribution can be generated using the `normrnd` function. This function accepts two parameters: the mean and the standard deviation. For example, the following code generates a normal distribution sample with a mean of 0 and a standard deviation of 1:
```
x = normrnd(0, 1, 1000);
```
# Theoretical Basis for Normal Distribution Parameter Estimation
The normal distribution, also known as the Gaussian distribution, is a continuous probability distribution that exists widely in nature and engineering applications. Parameter estimation for the normal distribution is a fundamental task in statistics, aiming to infer the unknown parameters of the normal distribution from sample data. This chapter will introduce the theoretical basis of normal distribution parameter estimation, including the probability density function of the normal distribution and the maximum likelihood estimation method.
### 2.1 Probability Density Function of the Normal Distribution
The probability density function of the normal distribution is:
```
f(x) = (1 / (σ√(2π))) * exp(-(1 / 2) * ((x - μ) / σ)^2)
```
Where:
* x is the random variable
* μ is the mean of the normal distribution
* σ is the standard deviation of the normal distribution
The probability density function represents the probability of the random variable taking a specific value given the mean and standard deviation. The probability density function of the normal distribution is bell-shaped, with its center at the mean and symmetrical on both sides.
### 2.2 Maximum Likelihood Estimation Method
The maximum likelihood estimation method is a parameter estimation method that selects the parameter values that maximize the sample data's likelihood function as the estimated values.
For the normal distribution, the likelihood function is:
```
L(μ, σ) = (1 / (n * (2πσ^2)^(n/2))) * exp(-(1 / 2) * Σ((x_i - μ) / σ)^2)
```
Where:
* n is the sample size
* x_i is the sample data
The maximum likelihood estimation method obtains the parameter estimates by solving the partial derivatives of the likelihood function with respect to the parameters μ and σ and setting them to zero:
```
μ_hat = (1 / n) * Σx_i
σ_hat^2 = (1 / n) * Σ((x_i - μ_hat)^2)
```
The maximum likelihood estimation method is a commonly used parameter estimation method, with the advantages that:
* It has asymptotic unbiasedness: When the sample size is large enough, the maximum likelihood estimate is unbiased.
* It has asymptotic efficiency: When the sample size is large enough, the maximum likelihood estimate is efficient, that is, it has the smallest variance.
# 3.1 Data Reading and Preprocessing
Before performing normal distribution parameter estimation, it is necessary to read and preprocess the data first. MATLAB provides various methods for reading data, such as:
```matlab
% Read data from a text file
data = load('data.txt');
% Read data from a CSV file
data = csvread('data.csv');
% Read data from an Excel file
data = xlsread('data.xlsx');
```
After reading the data, it is necessary to preprocess the data to ensure that it conforms to the assumptions of the normal distribution. Preprocessing steps include:
- **Handling missing values:** Missing values can affect the accuracy of parameter estimation. Missing values can be deleted or filled using interpolation or average values.
- **Handling outliers:** Outliers can distort parameter estimation. Outliers can be deleted or handled using Winsorization or the Tukey method.
- **Data transformation:** If the data does not conform to the normal distribution, data transformation can be performed, such as logarithmic transformation or square root transformation, to make the data closer to the normal distribution.
### 3.2 Parameter Estimation Methods
MATLAB provides various normal distribution parameter estimation methods, including:
#### 3.2.1 Maximum Likelihood Estimation Method
The maximum likelihood estimation method (MLE) is a classic parameter estimation method. MLE estimates parameters by maximizing the likelihood function. The likelihood function for the normal distribution is:
```
L(μ, σ) = (2πσ^2)^(-n/2) * exp(-Σ(x_i - μ)^2 / (2σ^2))
```
Where μ and σ are the mean and standard deviation of the normal distribution, respectively, and x_i are the data samples.
In MATLAB, the `mle` function is used for maximum likelihood estimation:
```matlab
% Estimate the parameters of the normal distribution
params = mle(data, 'distribution', 'normal');
% Get the estimated mean and standard deviation
mu = params(1);
sigma = params(2);
```
#### 3.2.2 Bayesian Estimation Method
The Bayesian estimation method is a parameter estimation method based on Bayes' theorem. The Bayesian estimation method requires specifying a prior distribution, which is the prior probability distribution of the parameters. The prior distribution for the normal distribution is typically a normal distribution or an inverse gamma distribution.
In MATLAB, the `bayesfit` function is used for Bayesian estimation:
```matlab
% Specify the prior distribution
prior = struct('mu', normrnd(0, 1), 'sigma', gamrnd(1, 1));
% Perform Bayesian estimation
params = bayesfit(data, prior, 'distribution', 'normal');
% Get the estimated mean and standard deviation
mu = params.mu;
sigma = params.sigma;
```
# 4. Applications of Normal Distribution Parameter Estimation
### 4.1 Hypothesis Testing
An important application of normal distribution parameter estimation is hypothesis testing. Hypothesis testing is a statistical method used to determine whether given data supports a particular hypothesis. In normal distribution parameter estimation, hypothesis testing can be used for the following purposes:
- **Testing whether the mean equals a specific value:** For example, a manufacturer claims that the average lifespan of its light bulbs is 1000 hours. We can use hypothesis testing to determine if this claim is supported by the data.
- **Testing whether the variance equals a specific value:** For example, a company claims that the standard deviation of its quality control process is 0.5. We can use hypothesis testing to determine if this claim is supported by the data.
The process of hypothesis testing involves the following steps:
1. **Propose a hypothesis:** Propose a hypothesis about the parameters of the normal distribution, such as the mean equals a specific value or the variance equals a specific value.
2. **Formulate an alternative hypothesis:** Propose an alternative hypothesis that contradicts the hypothesis, such as the mean does not equal a specific value or the variance does not equal a specific value.
3. **Determine the significance level:** Choose a significance level, usually 0.05, which represents the maximum probability of error we are willing to accept for the hypothesis to be true.
4. **Calculate the test statistic:** Calculate a test statistic based on the data that measures the degree of deviation of the data from the hypothesis.
5. **Determine the critical value:** Determine the critical value based on the significance level and degrees of freedom.
6. **Compare the test statistic and the critical value:** If the test statistic is greater than the critical value, then reject the hypothesis; otherwise, accept the hypothesis.
### 4.2 Confidence Interval Estimation
Confidence interval estimation is another important application of normal distribution parameter estimation. Confidence interval estimation is a statistical method used to estimate the true values of the parameters of the normal distribution. A confidence interval consists of two values, called the lower confidence limit and the upper confidence limit. The process of confidence interval estimation involves the following steps:
1. **Calculate the sample mean and sample variance:** Calculate the sample mean and sample variance from the data.
2. **Determine the confidence level:** Choose a confidence level, usually 95%, indicating that we have a 95% confidence that the true value falls within the confidence interval.
3. **Calculate the confidence interval:** Calculate the confidence interval based on the sample mean, sample variance, confidence level, and degrees of freedom.
Confidence interval estimation can be used for the following purposes:
- **Estimate the true value of the normal distribution mean:** For example, we can use confidence interval estimation to estimate the true average lifespan of light bulbs manufactured by a manufacturer.
- **Estimate the true value of the normal distribution variance:** For example, we can use confidence interval estimation to estimate the true standard deviation of a company's quality control process.
### 4.3 Parameter Sensitivity Analysis
Parameter sensitivity analysis is the third important application of normal distribution parameter estimation. Parameter sensitivity analysis is a statistical method used to determine the impact of changes in the parameters of the normal distribution on other statistics. The process of parameter sensitivity analysis involves the following steps:
1. **Select parameters:** Select the normal distribution parameters to be analyzed, such as the mean or variance.
2. **Change the parameter values:** Change the parameter values within a certain range.
3. **Calculate other statistics:** Calculate other statistics, such as confidence intervals or p-values for hypothesis tests, for each parameter value.
4. **Draw sensitivity graphs:** Draw graphs showing the relationship between parameter values and other statistics.
Parameter sensitivity analysis can be used for the following purposes:
- **Determine the impact of parameter changes on confidence intervals:** For example, we can use parameter sensitivity analysis to determine the impact of mean changes on the width of confidence intervals.
- **Determine the impact of parameter changes on hypothesis test results:** For example, we can use parameter sensitivity analysis to determine the impact of variance changes on the p-values of hypothesis tests.
# 5. Advanced Topics in Normal Distribution Parameter Estimation in MATLAB
### 5.1 Normal Mixture Models
The normal mixture model (GMM) is a probabilistic model that assumes the data is composed of a mixture of multiple normal distributions. GMM can be used to model data with multiple modes or peaks.
In MATLAB, the `fitgmdist` function can be used to fit a GMM. This function requires data and the number of mixture components as input.
```matlab
% Data
data = [***];
% Number of mixture components
K = 2;
% Fit GMM
gm = fitgmdist(data, K);
```
The parameters of the fitted GMM can be extracted from the `gm` object.
```matlab
% Means
means = gm.mu;
% Covariances
covariances = gm.Sigma;
% Mixture weights
weights = ***ponentProportion;
```
### 5.2 Nonparametric Estimation of the Normal Distribution
Nonparametric estimation methods for the normal distribution do not assume a distribution for the data. These methods are typically based on the rank or quantiles of the data.
In MATLAB, the `ksdensity` function can be used for nonparametric estimation of the normal distribution. This function requires data as input and returns the estimated probability density function.
```matlab
% Data
data = [***];
% Nonparametric estimation
[f, x] = ksdensity(data);
```
The estimated probability density function can be plotted to visualize the distribution of the data.
```matlab
plot(x, f);
```
### 5.3 Bayesian Inference for the Normal Distribution
Bayesian inference for the normal distribution is a method that uses Bayesian statistics to infer the parameters of the normal distribution. Bayesian inference requires prior distributions and a likelihood function as input.
In MATLAB, the `bayesstats` toolbox can be used for Bayesian inference of the normal distribution. This toolbox provides the `bayesfit` function, which can fit various Bayesian models for probability distributions.
```matlab
% Data
data = [***];
% Prior distribution
mu_prior = 5;
sigma_prior = 2;
% Likelihood function
likelihood = @(mu, sigma) normpdf(data, mu, sigma);
% Fit Bayesian model
model = bayesfit(data, 'Normal', 'mu_prior', mu_prior, 'sigma_prior', sigma_prior, 'likelihood', likelihood);
```
The parameters of the fitted Bayesian model can be extracted from the `model` object.
```matlab
% Posterior mean
mu_posterior = model.mu_posterior;
% Posterior standard deviation
sigma_posterior = model.sigma_posterior;
```
0
0
相关推荐
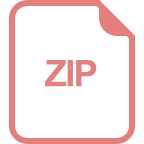
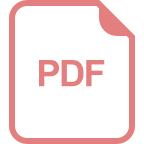
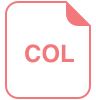
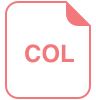
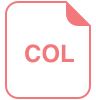
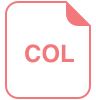
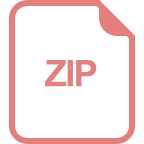
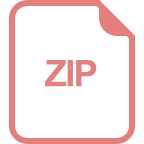