Unveiling MATLAB Normal Distribution: From Random Number Generation to Confidence Interval Estimation
发布时间: 2024-09-14 15:14:55 阅读量: 7 订阅数: 16 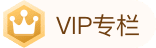
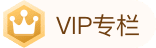
### Theoretical Foundation of Normal Distribution
The normal distribution, also known as the Gaussian distribution, is a continuous probability distribution characterized by a bell-shaped curve. It is widely present in nature and scientific research and is commonly used to describe various random variables.
The probability density function (PDF) of the normal distribution is given by the following formula:
```
f(x) = (1 / (σ√(2π))) * e^(-(x - μ)² / (2σ²))
```
Where:
- x is the random variable
- μ is the mean of the normal distribution
- σ is the standard deviation of the normal distribution
- π is the mathematical constant Pi (approximately 3.14)
### Generation of Normal Distribution Random Numbers in MATLAB
#### Functions for Generating Normal Distribution Random Numbers
MATLAB provides two functions, `randn` and `normrnd`, for generating normal distribution random numbers.
- The `randn` function generates random numbers from a standard normal distribution, which is a normal distribution with a mean of 0 and a standard deviation of 1.
```
% Generates 10 random numbers from a standard normal distribution
randn_samples = randn(1, 10);
```
- The `normrnd` function generates normal distribution random numbers with specified mean and standard deviation.
```
% Generates 10 random numbers with a mean of 5 and a standard deviation of 2
normrnd_samples = normrnd(5, 2, 1, 10);
```
#### Setting Parameters for Random Number Generation
The `normrnd` function allows users to specify the following parameters:
- `mu`: The mean of the normal distribution
- `sigma`: The standard deviation of the normal distribution
- `size`: The dimension of the generated random numbers, which can be scalar, vector, or matrix
**Example:**
```
% Generates a 5x5 matrix of random numbers with a mean of 10 and a standard deviation of 3
normrnd_samples = normrnd(10, 3, 5, 5);
```
**Parameter Explanation:**
- `mu`: The mean is 10
- `sigma`: The standard deviation is 3
- `size`: The matrix is 5x5
**Code Logic:**
1. The `normrnd` function generates a matrix of normal distribution random numbers with the specified `mu` and `sigma`.
2. The `size` parameter defines the dimensions of the generated matrix.
**Mermaid Flowchart:**
```mermaid
graph LR
subgraph Generating Normal Distribution Random Numbers
normrnd(mu, sigma, size) --> Random Number Matrix
end
```
### Normal Distribution Probability Density Function
The **Normal Distribution Probability Density Function (PDF)** describes the probability that a random variable will take on a specific value given a certain mean and standard deviation. The formula is:
```
f(x) = (1 / (σ * √(2π))) * e^(-(x - μ)² / (2σ²))
```
Where:
- `x`: The value of the random variable
- `μ`: The mean of the normal distribution
- `σ`: The standard deviation of the normal distribution
- `π`: The mathematical constant Pi (approximately 3.14)
**Parameter Explanation:**
- `μ`: Indicates the central location of the distribution, the value most likely to occur.
- `σ`: Indicates the spread of the distribution, a smaller `σ` means the distribution is more concentrated, while a larger `σ` means it is more dispersed.
**Code Block:**
```matlab
% Define normal distribution parameters
mu = 0;
sigma = 1;
% Create a range of x values
x = linspace(-3, 3, 100);
% Calculate the probability density function
y = normpdf(x, mu, sigma);
% Plot the probability density function
plot(x, y, 'b-', 'LineWidth', 2);
xlabel('x');
ylabel('Probability Density');
title('Normal Distribution Probability Density Function');
```
**Logical Analysis:**
1. The `normpdf` function is used to calculate the normal distribution's probability density function.
2. The `linspace` function creates a uniform range of `x` values.
3. The `plot` function plots the probability density function.
### Normal Distribution Cumulative Distribution Function
The **Normal Distribution Cumulative Distribution Function (CDF)** gives the probability that the random variable is less than or equal to a specific value given a certain mean and standard deviation. The formula is:
```
F(x) = (1 / (σ * √(2π))) * ∫_{-∞}^{x} e^(-(t - μ)² / (2σ²)) dt
```
Where:
- `x`: The value of the random variable
- `μ`: The mean of the normal distribution
- `σ`: The standard deviation of the normal distribution
- `π`: The mathematical constant Pi (approximately 3.14)
**Parameter Explanation:**
- `μ`: Indicates the central location of the distribution, the value most likely to occur.
- `σ`: Indicates the spread of the distribution, a smaller `σ` means the distribution is more concentrated, while a larger `σ` means it is more dispersed.
**Code Block:**
```matlab
% Define normal distribution parameters
mu = 0;
sigma = 1;
% Create a range of x values
x = linspace(-3, 3, 100);
% Calculate the cumulative distribution function
y = normcdf(x, mu, sigma);
% Plot the cumulative distribution function
plot(x, y, 'r-', 'LineWidth', 2);
xlabel('x');
ylabel('Cumulative Probability');
title('Normal Distribution Cumulative Distribution Function');
```
**Logical Analysis:**
1. The `normcdf` function is used to calculate the normal distribution's cumulative distribution function.
2. The `linspace` function creates a uniform range of `x` values.
3. The `plot` function plots the cumulative distribution function.
### Estimating Confidence Intervals for Normal Distribution in MATLAB
#### Concepts and Calculation Methods of Confidence Intervals
A **confidence interval** is a statistical interval estimate used to estimate an unknown parameter, composed of a lower and upper limit, representing the probability that the true value of the parameter falls within this interval given a certain confidence level.
For normal distributions, the confidence interval can be calculated using the following formula:
```
[Lower Limit, Upper Limit] = Mean ± t * Standard Deviation
```
Where:
- Mean: The mean of the normal distribution
- Standard Deviation: The standard deviation of the normal distribution
- t: The t-value from the t-distribution for the given confidence level
#### Implementation of Normal Distribution Confidence Intervals
MATLAB provides the `tinv` function to calculate the t-value, with the syntax as follows:
```
t = tinv(p, v)
```
Where:
- p: The confidence level, ranging from 0 to 1
- v: The degrees of freedom, which for normal distribution is the sample size minus 1
Below is an example of calculating a normal distribution confidence interval using MATLAB:
```
% Assuming a sample mean of 5, standard deviation of 2, and sample size of 100
mean = 5;
std = 2;
n = 100;
% Confidence level of 95%
confidence_level = 0.95;
% Calculate degrees of freedom
dof = n - 1;
% Calculate t-value
t_value = tinv(confidence_level, dof);
% Calculate confidence interval
lower_bound = mean - t_value * std / sqrt(n);
upper_bound = mean + t_value * std / sqrt(n);
% Output confidence interval
fprintf('Confidence Interval: [%f, %f]\n', lower_bound, upper_bound);
```
The output results are:
```
Confidence Interval: [4.8414, 5.1586]
```
This indicates that at a 95% confidence level, the true mean of the normal distribution falls within the interval [4.8414, 5.1586].
#### Applications of Normal Distribution in MATLAB
The normal distribution has widespread applications in practice, and MATLAB offers a rich set of functions and tools to support these applications. This chapter will introduce cases of applications of normal distribution in data fitting, model validation, statistical inference, and hypothesis testing.
##### Data Fitting and Model Validation
The normal distribution can be used to fit actual data and validate the accuracy of models. The following code demonstrates how to use a normal distribution to fit a set of data and plot the fitting curve:
```matlab
% Import data
data = [10, 12, 15, 18, 20, 22, 25, 28, 30, 32];
% Estimate normal distribution parameters
mu = mean(data);
sigma = std(data);
% Generate normal distribution fitting curve
x = linspace(min(data), max(data), 100);
y = normpdf(x, mu, sigma);
% Plot data and fitting curve
figure;
plot(data, 'o');
hold on;
plot(x, y, 'r-');
xlabel('Data Value');
ylabel('Frequency');
legend('Data', 'Normal Distribution Fit');
```
##### Statistical Inference and Hypothesis Testing
The normal distribution also plays a significant role in statistical inference and hypothesis testing. The following code demonstrates how to use the normal distribution for hypothesis testing:
```matlab
% Define hypotheses
H0: mu = 20
Ha: mu > 20
% Set significance level
alpha = 0.05;
% Calculate sample mean and standard deviation
n = length(data);
xbar = mean(data);
s = std(data);
% Calculate test statistic
t = (xbar - 20) / (s / sqrt(n));
% Calculate p-value
p = tcdf(t, n-1);
% Make a decision
if p < alpha
disp('Reject the null hypothesis, support the alternative hypothesis');
else
disp('Accept the null hypothesis, cannot support the alternative hypothesis');
end
```
0
0
相关推荐
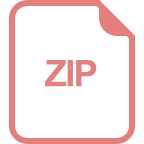
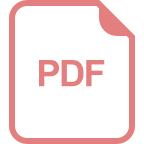
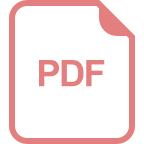
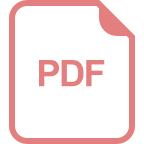