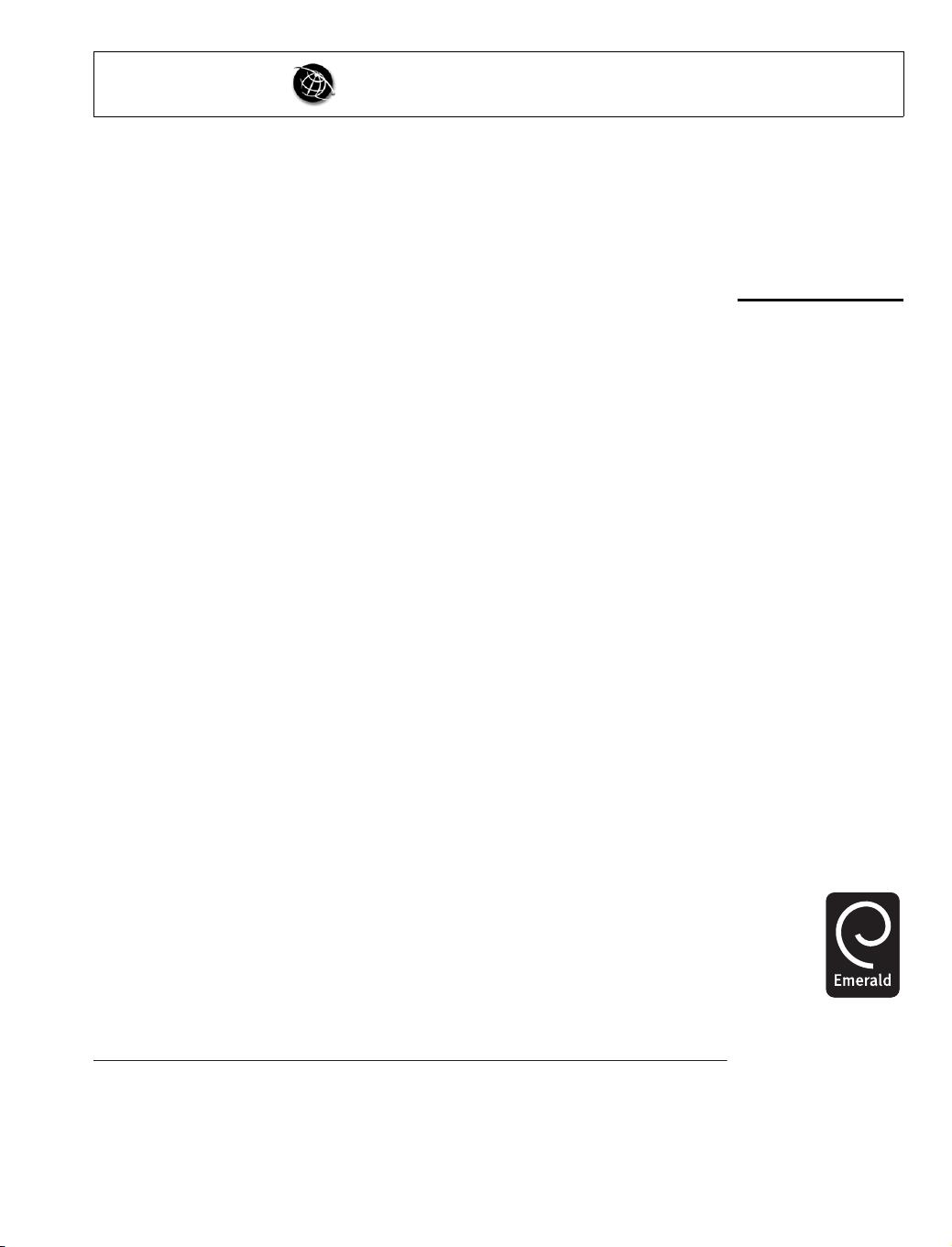
A hybrid neural genetic method
for load forecasting based
on phase space reconstruction
Wang Junguo
School of Mechanical Science and Engineering,
Southwest Jiaotong University, Chengdu, China, and
Zhou Jianzhong and Peng Bing
College of Hydropower and Information Engineering,
Huazhong University of Science and Technology, Wuhan, China
Abstract
Purpose – The purpose of this paper is to improve forecasting accuracy for short-term load series.
Design/methodology/approach – A forecasting method based on chaotic time series and optimal
diagonal recurrent neural networks (DRNN) is presented. The input of the DRNN is determined by the
embedding dimension of the reconstructed phase space, and adaptive dynamic back propagation
(DBP) algorithm is used to train the network. The connection weights of the DRNN are optimized via
modified genetic algorithms, and the best results of optimization are regarded as initial weights for the
network. The new method is applied to predict the actual short-term load according to its chaotic
characteristics, and the forecasting results also validate the feasibility.
Findings – For the chaos time series, the hybrid neural genetic method based on phase space
reconstruction can carry out the short-term prediction with the higher accuracy.
Research limitations/implications – The proposed method is not suited to medium and long-term
load forecasting.
Practical implications – The accuracy of the load forecasting is important to the economic and
secure operation of power systems; also, the neural genetic method can improve forecasting accuracy.
Originality/value – This paper will help overcome the defects of traditional neural network and
make short-term load forecasting more accurate and fast.
Keywords Neural nets, Loading (physics), Forecasting, Electric power systems, Time series analysis
Paper type Research paper
1. Introduction
Short-term load forecast (STLF) is important to daily operations of a power utility. It is
required for unit commitment, energy transfer scheduling, and load dispatch. With the
emergence of load management strategies, the short-term forecast has played a broader
role in utility operations (Lee et al., 1992; Ling et al., 2003). Since the time series of
power loads have some chaotic characteristics, Farmer and Sidorowich (1987)
proposed a state-space reconstruction method based on the geometry of the embedded
data for the discovery of a relationship between past and future points. For relevant
studies on predictions, please refer to OuYang et al. (2000), Sydow et al. (2001),
Lin (2001) and Liu et al. (2002).
The current issue and full text archive of this journal is available at
www.emeraldinsight.com/0368-492X.htm
This paper is supported by Notional Natural Science Foundation of China 50539140 and
50579022.
A method for
load forecasting
1291
Kybernetes
Vol. 39 No. 8, 2010
pp. 1291-1297
q Emerald Group Publishing Limited
0368-492X
DOI 10.1108/03684921011063574