没有合适的资源?快使用搜索试试~ 我知道了~
少样本语义分割任务中的动态原型卷积网络
Support ImageQuery ImageSupport ImageQuery Image115530用于少样本语义分割的动态原型卷积网络0刘杰1*,包彦琦2*,谢国森3,4†,熊欢4,Jan-Jakob Sonke5,Efstratios Gavves101阿姆斯特丹大学,荷兰2东北大学,中国5荷兰癌症研究所,荷兰3南京理工大学,中国4穆罕默德∙本∙扎耶德人工智能大学,阿联酋0摘要0少样本语义分割(FSS)的关键挑战是如何在episode训练场景下,为支持和查询特征及/或它们的原型之间定制一个理想的交互。大多数现有的FSS方法通过仅仅利用简单的操作(例如余弦相似度和特征连接)来实现这种支持/查询交互,用于分割查询对象。然而,这些交互方法通常不能很好地捕捉到FSS中广泛遇到的查询图像中的固有对象细节,例如,如果要分割的查询对象有孔洞和槽,准确的分割几乎总是会发生。为此,我们提出了一种动态原型卷积网络(DPCN),以完全捕捉用于准确FSS的上述固有细节。具体而言,在DPCN中,首先提出了一种动态卷积模块(DCM),用于从支持前景生成动态卷积核,然后使用这些卷积核对查询特征进行卷积操作以实现信息交互。此外,我们还为DPCN配备了一个支持激活模块(SAM)和一个特征过滤模块(FFM),用于分别生成伪掩码和过滤查询图像中的背景信息。SAM和FFM可以共同从查询特征中挖掘丰富的上下文信息。我们的DPCN在k-shotFSS设置下也是灵活高效的。在PASCAL-5i和COCO-20i上进行了大量实验证明,DPCN在1-shot和5-shot设置下都具有优越的性能。01. 引言0由于深度卷积神经网络的进步,语义分割取得了巨大的成功[9, 10,22]。然而,大多数领先的图像语义分割模型依赖于大量具有像素级注释的训练图像,这需要巨大的人力。半监督和弱监督分割方法[15, 24, 28]被提出来缓解这种费用0* 平等贡献。†通讯作者。0相似度/求和/连接0池化/聚类0...前景/背景原型0卷积核生成0动态卷积0动态卷积核0特征过滤0(a)现有的基于原型的方法0图1.(a)现有的基于原型的方法和(b)提出的DPCN之间的比较。(a)现有方法通常采用支持前景的掩码平均池化或聚类来获得多个前景/背景原型。然后,支持和查询之间的信息交互可以是简单的操作,例如余弦相似度,逐元素求和和通道连接。然而,这种范式无法分割植物的固有细节,因为这种不足的交互无法很好地解决FSS中的外观和形状变化(例如,植物中的孔洞和槽)。(b)我们的DPCN可以很好地分割植物并捕捉固有的细微细节。这得益于使用从支持前景特征生成的动态卷积核对查询特征进行动态卷积。0半监督和弱监督方法在只有少量标注样本的情况下,都会面临显著的性能下降。在这种情况下,引入了少样本语义分割(FSS)[20],它允许在只有少量标注样本的情况下对新对象类别进行密集的像素级预测。通常,大多数FSS方法采用基于episode的元学习策略[30],每个episode由支持集和查询集组成,它们共享相同的对象类别。支持集包含少量具有像素级注释的支持图像。FSS模型应该能够在支持集的指导下学习预测查询集中图像的分割掩码。学习是基于训练期间可用的带有注释的episode进行的。在测试时,模型应该能够对查询进行分割115540再次提供与感兴趣的类别相关的图像,同时提供相应的支持集,但这次查询和支持集中的感兴趣类别是新颖的,之前没有见过的。目前,最主要的FSS方法是基于原型的方法[12,25]。如图1(a)所示,基于原型的范式通常通过利用掩码平均池化和/或对支持特征进行聚类来生成多个前景和/或背景原型。这些原型应该包含支持图像中目标对象的代表性信息,因此它们与查询特征的余弦相似性、逐元素求和和特征串联的相互作用可以为查询图像中的对象产生必要的预测。然而,仅仅依靠这些有限的原型和简单的操作所实现的预测必然会丢失查询图像中的一些内在对象细节。例如,如图1(a)所示,由于植物具有孔洞和槽,这些是内在细节,分割对象无法很好地覆盖这些细节,即在这种情况下实现了有缺陷的过分分割。此外,在FSS中存在大的对象变化(例如外观和尺度)时,通常很难仅通过考虑支持信息来全面编码目标对象的充分模式,如大多数先前的基于原型的方法所示。为了解决上述挑战,我们提出了一种动态原型卷积网络(DPCN),以完全捕捉准确的FSS的内在对象细节。DPCN属于基于原型的方法,但具有几个优雅的扩展和优点。具体而言,我们首先提出了一种动态卷积模块(DCM),以实现更充分的支持和查询特征之间的交互,从而为查询对象提供更准确的预测。如图1(b)所示,我们利用三个动态核,即一个方形核和两个非对称核,从支持前景特征生成。然后,使用这些动态核在查询特征上并行进行三个卷积操作。这种交互策略简单而重要,可以全面解决大的对象变化(例如外观和尺度),并捕捉内在对象细节。直观地说,方形核能够捕捉对象的主要信息(例如图1(b)中的植物的主体);相比之下,非对称核(即大小为d×1或1×d的核)旨在捕捉微小的对象细节,例如图1(b)中的叶子。因此,配备DCM的DPCN可以以极其简单的方式更好地处理内在对象细节。此外,为了全面编码目标对象的充分模式,我们提出了一种支持激活模块(SAM)和特征过滤模块(FFM),以尽可能多地从查询图像中挖掘与对象相关的上下文信息。具体而言,SAM生成支持0使用高级支持和查询特征绘制端口激活图和初始伪查询掩码。然后将支持原型和伪查询前景特征融合,生成查询图像在FFM中的精细伪掩码。与原始伪查询掩码相比,精细掩码包含更多的对象前景上下文,同时过滤一些噪声信息。因此,来自支持和查询图像的丰富对象相关上下文信息被聚合到最终特征中,从而提高了分割性能。我们的主要贡献如下:•我们提出了一种动态原型卷积网络(DPCN),以捕捉准确的FSS的内在对象细节。据我们所知,我们是FSS领域中第一个这样做的人。•我们提出了一种新颖的动态卷积模块(DCM),以实现充分的支持-查询交互。DCM可以作为即插即用的组件,改进现有的原型学习方法。•我们提出了一种支持激活模块(SAM)和特征过滤模块(FFM),以从查询图像中挖掘目标对象的互补信息。02. 相关工作02.1. 语义分割0语义分割是一项经典的计算机视觉任务,旨在为输入图像提供逐像素的预测。最近,各种网络[14]被积极设计用于进一步改进语义分割结果。为了捕捉更多的上下文信息,提出了扩张卷积[33]、金字塔池化[40]和可变形卷积[3]等方法来扩大感受野。同时,一些模型利用注意机制[6, 27, 34,37]来捕捉语义分割中的远距离依赖关系,达到了最先进的性能。然而,这些语义分割方法在提供不充足的训练数据时仍然无法保持其初始性能。02.2. 少样本语义分割0少样本语义分割(FSS)是指在给定少量像素级标注的支持图像的情况下,学习对查询图像中的目标对象进行分割。大多数现有的FSS方法采用两分支架构,即在基础类上进行元训练,然后在不相交的新颖类上进行元测试。OSLSM[20]是第一个两分支FSS模型。接下来,PL[4]引入了原型学习范式,从支持图像生成原型来指导查询对象的分割。最近,研究界出现了许多基于原型的FSS方法,如CANet [36]、SG-One [39]、PANet [26]、PMMs[31]、PFENet [25]和ASGNet [12]。关键思想是SAMHigh-levelFeatureMid-levelFeatureSupportMaskInitialPseudo MaskRefinedPseudo MaskElement-wiseMultiplicationSumConvolution����������������Mask PredictionDynamicKernelsActivationMapsSupport Activation Module�����Feature Filtering ModuleFeatureExtractionInput���������Mean�����CCCC��������������Figure 2. Overall architecture of our proposed dynamic prototype convolution network (DPCN). Firstly, support activation module(SAM) is introduced to generate activation maps and initial pseudo mask of target object in query image using high-level support and queryfeature. Then, feature filtering module (FFM) takes input mid-level support and query feature as well as initial pseudo mask to producerefined pseudo mask, which is leveraged to filter most background information in query feature. Meanwhile, dynamic convolution module(DCM) implements three groups of dynamic convolution over query features in parallel using kernels (multiple prototypes) generatedfrom support foreground features, to propagate rich context information from support to query features. Finally, the updated features areconcatenated and fed into a decoder for the final query segmentation mask prediction.of these methods lies in generating or rearranging represen-tative prototypes using different strategies, then the inter-action between prototypes with query features can be for-mulated as a few-to-many matching problem.However,these prototype learning methods inevitably cause informa-tion loss due to limited prototypes. Therefore, graph-basedmethods have thrived recently as they try to preserve struc-tural information with many-to-many matching mechanism.For instance, PGNet [36] applies attentive graph reasoningto propagate label information from support data to querydata.SAGNN [30] constructs graph nodes using multi-scale features and performs k-step reasoning over nodes tocapture cross-scale information. Most recently, HSNet [17]proposes to tackle the FSS task from the perspective of vi-sual correspondence. It implements efficient 4D convolu-tions over multi-level feature correlation and achieves greatsuccess. Different from previous methods, we try to per-form sufficient interaction between support and query fea-tures using dynamic convolution, and mine as much com-plementary target information from both support and queryfeatures.2.3. Dynamic Convolution NetworksDynamic convolution networks aim to generate diversekernels and implement convolution over input feature withthese kernels.Many previous works have explored theeffectiveness of dynamic convolution in deep neural net-works. DFN [11] proposes a dynamic filter network wherefilters are generated dynamically conditioned on input andachieves state-of-the-art performance on video and stereoprediction task. [2] aggregates multiple parallel convolu-tion kernels dynamically based upon their attentions, and itboosts both image classification and keypoint detection ac-curacy. Dynamic convolution is also used in DMNet [8] toadaptively capture multi-scale contents for predicting pixel-level semantic labels. The core of these methods is con-structing multiple kernels from input features.Most re-cently, dynamic convolution is introduced into the few-shotobject detection task by [38], which generates various ker-nels from the object regions in support image and then im-plements convolution over query feature using these ker-nels, leading to a more representative query feature. In thispaper, we propose to generate dynamic kernels from fore-ground support feature to interact with query feature by con-volution. Instead of only using square kennels as in [38], wealso introduce asymmetric kernels to capture subtle objectdetails. Experiments in §4.3 demonstrate well the effective-ness of our method.3. Method3.1. Problem SettingWe adopt the standard FSS setting, i.e., following theepisode-based meta-learning paradigm [23]. We start fromclasses Ctr and Cts for the training set Dtr and the test setDts, respectively.The key difference between FSS andgeneral semantic segmentation task is that Ctr and Cts inFSS are disjoint, Ctr ∩ Cts = ∅. Both Dtr and Dts con-sist of thousands of randomly sampled episodes, and eachepisode (S, Q) includes a support set S, and a query set Q115550核生成器0支持图像0查询图像0权重共享0MAP扩展0卷积0解码器0动态卷积模块0MASKWindows ExtractionMatMul���������� × �� × ���� × ���� × �� × ���������� × ��× �� ������ × ��× �� ��Corr���� × �� �� × �� ��Mean(0) & Max(1)Norm��M�����Figure 3. Illustration of the support activation module (SAM).for a specific class c. For the k-shot setting, the supportset that contains k image-mask pairs can be formulated asS = {(Iis, M is)}ki=1, where Iis represents ith support imageand M is indicates corresponding binary mask. Similarly,we define the query set as Q = {(Iq, Mq)}, where Iq isthe query image and its binary mask Mq is only availablein the model training phase. In the meta-training stage, theFSS model takes as input S and Iq from a specific classc and generates a predicted maskˆMq for the query im-age. Then the model can be trained with the supervisionof a binary cross-entropy loss between Mq and ˆMq. Fi-nally, the model takes multiple randomly sampled episodes(Stsi , Qtsi )Ntsi=1 from Dts for evaluation. Next, the 1-shot set-ting is adopted to illustrate our method for simplicity.3.2. OverviewAs in Fig. 2, our dynamic prototype convolution network(DPCN) consists of three key modules, i.e., support activa-tion module (SAM), feature filtering module (FFM), anddynamic convolution module (DCM). Specifically, giventhe support and query images, Is and Iq , we use a commonbackbone with shared weights to extract both mid-level andhigh-level features. We then have the SAM whose task is togenerate an initial pseudo mask M 0pse for the target object inthe query image. After SAM, a FFM follows, which aims torefine the pseudo mask and filter out irrelevant backgroundinformation in the query feature. To incorporate relevantcontextual information, we then employ the DCM, whichlearns to generate custom kernels from support foregroundfeature and employ dynamic convolution over query fea-ture. We then feed the pseudo masks and features computedby the dynamic convolutions into a decoder to predict the fi-nal segmentation mask ˆMq for the query image. Next, wedescribe each of the aforementioned modules in detail.3.3. Support Activation ModuleInspired by PFENet [16, 25], recent FSS models [29,30] usually leverage high-level features (e.g., conv5 ofResNet50) from the support and query set to generate theprior mask indicating the rough location of the target objectin the query image. As this prior mask is usually obtainedby element-to-element or square region-based matching be-tween feature maps, a holistic context is not taken into ac-count.To counter this, with the support activation module wegenerate multiple activation maps of the target object inthe query image using holistic region-to-region matching.Specifically, as in Fig. 3, SAM takes as input the high-levelsupport feature xhs ∈ RCh×Hs×Ws, the corresponding bi-nary mask Ms ∈ RHs×Ws, as well as the high-level queryfeature xhq ∈ RCh×Hq×Wq, where Ch is the channel dimen-sion, Hs, Ws, Hq, Wq are the height and width of supportand query feature, respectively.To perform holistic matching, we first need to generateregion features Rs and Rq with a fixed window operationW sliding on the support and query features, respectively.Rs = W(xhs ⊗ Ms) ∈ Rdhdw×Ch×HsWs,Rq = W(xhq ) ∈ Rdhdw×Ch×HqWq,(1)where ⊗ stands for the Hadamard product and dh, dw arethe window height and width. In our experiments we opt forsymmetrical and asymmetrical windows, i.e., (dh, dw) ∈{(5, 1), (3, 3), (1, 5)} that are comprehensive and holisticregions, to account for possible object geometry variances.Having the region features, we proceed with matching bycomputing their cosine similarity, which generates the re-gional matching map Corr ∈ Rdhdw×HsWs×HqWq. No-tably, we utilize both square window (3,3) and asymmet-rical windows (i.e., (5,1) and (1,5)), where square windowcan introduce more contextual information on regular partof target objects like the main body of object plants, asym-metrical windows can incorporate contextual details of slen-der part (e.g., leaves of plants).We generate the final activation map Mact ∈ RHq× Wqby taking the mean value among all regions and the maxi-mal value among all support features followed by normal-ization operation. As we have three windows, we have threeactivation maps, {M iact}3i=1. In the end, we obtain the ini-tial pseudo-mask M 0pse ∈ RHq×Wq, which indicates therough location of target objects, by a mean operation.3.4. Feature Filtering ModuleAs in Fig. 2, the feature filtering module is con-structed on mid-level support and query features, i.e., xs ∈RC×H×W and xq ∈ RC×H×W where C, H, W are chan-nel, height, and width, respectively. Given xs, xq, and theinitial pseudo mask M 0pse, the feature filtering module re-fines the pseudo mask, which is used to filter out irrelevantbackground information in the query image. We first applymasked average pooling on the features from the support setto get prototype vector p ∈ RC×1×1:p = average(xs ⊗ R(Ms)),(2)where R reshapes support mask Ms to be the same shapeas xs. Then, we expand the support prototype vector p tomatch the dimensions of the feature maps, xp ∈ RC×H×W ,11556Kernel GeneratorForeground Extraction������������������������������������ × ������������1D Pooling1D PoolingS× ������������������������2 × ������������ConvConvConvS× 1 × ������������1× ������������ × ������������S× ������������ × ������������������������������������������������������������������������������������������������������������������������������������������������ℎ������������������������������������������������������������������������������������������������������������������������������������2C × ������������ ×W������������ ×WFigure 4. Illustration of kernel generator in DCM.and combine the target object information from both thesupport and query features. We refine the pseudo mask withthe help of a smaller network F composed of a 2D convo-lution layer followed by a sigmoid function,M rpse = F((xq ⊗ R(M 0pse)) ⊕ xp) ∈ RH×W ,(3)where ⊕ stands for the element-wise sum. Compared withM 0pse, M rpse gives more accurate estimation of the objectlocation in the query image. Lastly, we obtain the final fil-tered query feature that discards irrelevant background bycombining the feature xq with the prior mask :˜xq = (xq ⊗ M rpse) ⊕ xq ∈ RC×H×W .(4)3.5. Dynamic Convolution ModuleIn the previous step we obtain a foreground feature fromthe query, which is minimally affected by irrelevant back-ground. Still, the operations so far have been so that to pro-vide a rough estimate of the location of the target object.For accurate segmentation, however, much finer pixel-levelpredictions are required. In the absence of significant datato train our filters on, we introduce dynamic convolutions.We illustrate DCM in Fig. 2, and Fig. 4 depicts the detailsof the kernel generator.Dynamic convolutions rely on meta-learning to inferwhat are the optimal kernel parameters given a subset offeatures, agnostic to the unknown underlying class seman-tics. Specifically, we input the mid-level support featurexs and the corresponding mask Ms to a kernel generator,which generates dynamic kernels, i.e., one group squarekernel and two groups of asymmetrical kernels.Then,we carry out three convolution operations over the filteredquery feature ˜xq using dynamic kernels. Firstly, we extractforeground vectors Pfg from support feature:Pfg = Fe(xs ⊗ Ms) ∈ RNfg×C,(5)where Fe is the foreground extraction function without anylearnable parameters, Nfg represents the number of fore-ground vectors. Next, two consecutive 1D pooling opera-tions with kernel size S and S2 are leveraged to obtain twogroups of prototypes ps ∈ RS×C and ps2 ∈ RS2×C:ps = pools(Pfg), ps2
下载后可阅读完整内容,剩余1页未读,立即下载

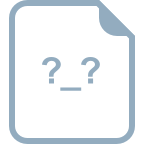
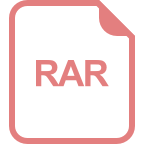
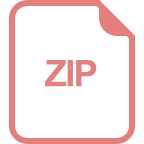
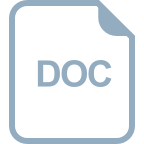
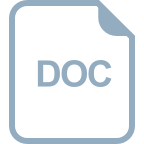
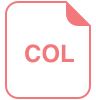
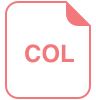
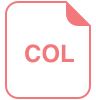
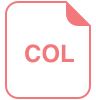
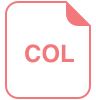
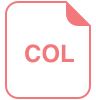
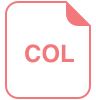
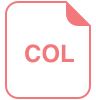
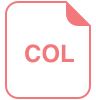
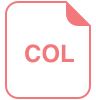


cpongm
- 粉丝: 5
- 资源: 2万+
上传资源 快速赚钱
我的内容管理 展开
我的资源 快来上传第一个资源
我的收益
登录查看自己的收益我的积分 登录查看自己的积分
我的C币 登录后查看C币余额
我的收藏
我的下载
下载帮助

最新资源
- 高清艺术文字图标资源,PNG和ICO格式免费下载
- mui框架HTML5应用界面组件使用示例教程
- Vue.js开发利器:chrome-vue-devtools插件解析
- 掌握ElectronBrowserJS:打造跨平台电子应用
- 前端导师教程:构建与部署社交证明页面
- Java多线程与线程安全在断点续传中的实现
- 免Root一键卸载安卓预装应用教程
- 易语言实现高级表格滚动条完美控制技巧
- 超声波测距尺的源码实现
- 数据可视化与交互:构建易用的数据界面
- 实现Discourse外聘回复自动标记的简易插件
- 链表的头插法与尾插法实现及长度计算
- Playwright与Typescript及Mocha集成:自动化UI测试实践指南
- 128x128像素线性工具图标下载集合
- 易语言安装包程序增强版:智能导入与重复库过滤
- 利用AJAX与Spotify API在Google地图中探索世界音乐排行榜
资源上传下载、课程学习等过程中有任何疑问或建议,欢迎提出宝贵意见哦~我们会及时处理!
点击此处反馈


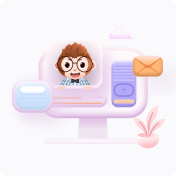
安全验证
文档复制为VIP权益,开通VIP直接复制
