现有数据集的.csv文件,写出用ARMA滤波器实现图卷积神经网络进行的卷积的python代码
时间: 2024-01-21 20:19:08 浏览: 96
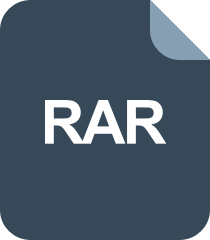
卷积神经网络python代码
首先,需要导入必要的库:numpy、pandas、scipy、networkx、torch、torch_geometric
```python
import numpy as np
import pandas as pd
from scipy.sparse import coo_matrix
import networkx as nx
import torch
from torch_geometric.data import Data
from torch_geometric.nn import ARMAConv
```
接着,读取.csv文件中的数据,并将其转换为稀疏矩阵格式:
```python
df = pd.read_csv('data.csv')
edge_index = np.array(df[['source', 'target']])
edge_index = torch.from_numpy(edge_index).T.contiguous()
edge_index, _ = torch.unique(edge_index, sorted=True, return_inverse=True)
num_nodes = edge_index.max().item() + 1
# 构建邻接矩阵
adj_matrix = coo_matrix((np.ones(edge_index.shape[1]), edge_index.numpy()), shape=(num_nodes, num_nodes))
```
然后,根据邻接矩阵构建图,并使用ARMAConv实现卷积:
```python
# 构建图
graph = nx.from_scipy_sparse_matrix(adj_matrix)
edge_attr = np.array([graph.get_edge_data(edge[0], edge[1])['weight'] for edge in graph.edges()])
edge_attr = torch.from_numpy(edge_attr).float()
# 构建Data对象
x = torch.randn(num_nodes, 16) # 假设每个节点的特征向量维度为16
data = Data(x=x, edge_index=edge_index, edge_attr=edge_attr)
# ARMAConv卷积
conv = ARMAConv(16, 32, 2, bias=True)
x = conv(x=data.x, edge_index=data.edge_index, edge_weight=data.edge_attr)
```
最后,得到卷积后的节点特征向量x。
阅读全文
相关推荐






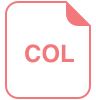
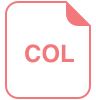
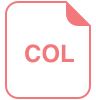
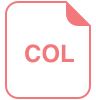
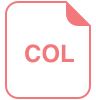
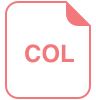
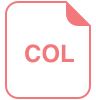
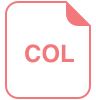
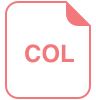