depth == CV_8U || depth == CV_32F
时间: 2024-08-13 14:04:52 浏览: 79
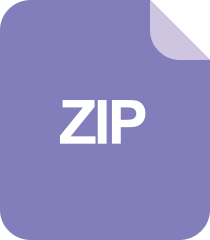
CV4.zip_Computer Vision_Photometric Stereo_depth of field_surfac
在OpenCV中,`depth`是一个表示图像像素深度的关键字,它通常用于描述像素值的存储方式和范围。`CV_8U`代表8位无符号整数(unsigned char),这是最常见的深度,用于存储灰度或彩色图像,每个像素占用1字节,值域为0到255。而`CV_32F`则代表32位单精度浮点数(float),常用于处理需要高精度数学运算的图像,如计算和分析。
`depth == CV_8U || depth == CV_32F`这个条件判断表达式意味着如果图像的深度是8位无符号整数或者32位浮点数,那么这个条件就会为真。这在处理不同类型的图像数据时非常有用,例如在读取图像、进行特定运算或转换图像格式之前,可以根据这个条件来确定后续操作的策略。
阅读全文
相关推荐
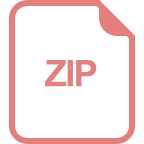
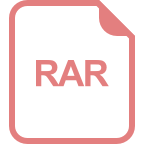
















