print(img_size.shape, batch_size.shape),帮我看看这段有什么问题
时间: 2024-03-09 08:51:02 浏览: 28
这段代码会报错,因为 shape 和 size 是 NumPy 数组的属性,而不是普通的变量和常量。因此,如果 img_size 和 batch_size 不是 NumPy 数组,就不能使用 shape 和 size 方法。
如果 img_size 和 batch_size 是普通的变量或常量,可以使用内置函数 len() 来获取它们的大小,而不是使用 shape 和 size:
```python
img_size = 224
batch_size = 32
print("img_size 的大小为:", len(img_size)) # 报错,无法获取大小
print("batch_size 的大小为:", len(batch_size)) # 报错,无法获取大小
```
正确的写法应该是将 img_size 和 batch_size 定义为 NumPy 数组,例如:
```python
import numpy as np
img_size = np.array([224, 224])
batch_size = np.array([32])
print("img_size 的大小为:", img_size.shape) # 输出 (2,)
print("batch_size 的大小为:", batch_size.shape) # 输出 (1,)
```
这样就可以使用 shape 方法来获取它们的大小了。
相关问题
下面的代码哪里有问题,帮我改一下from __future__ import print_function import numpy as np import tensorflow import keras from keras.models import Sequential from keras.layers import Dense,Dropout,Flatten from keras.layers import Conv2D,MaxPooling2D from keras import backend as K import tensorflow as tf import datetime import os np.random.seed(0) from sklearn.model_selection import train_test_split from PIL import Image import matplotlib.pyplot as plt from keras.datasets import mnist images = [] labels = [] (x_train,y_train),(x_test,y_test)=mnist.load_data() X = np.array(images) print (X.shape) y = np.array(list(map(int, labels))) print (y.shape) x_train, x_test, y_train, y_test = train_test_split(X, y, test_size=0.30, random_state=0) print (x_train.shape) print (x_test.shape) print (y_train.shape) print (y_test.shape) ############################ ########## batch_size = 20 num_classes = 4 learning_rate = 0.0001 epochs = 10 img_rows,img_cols = 32 , 32 if K.image_data_format() =='channels_first': x_train =x_train.reshape(x_train.shape[0],1,img_rows,img_cols) x_test = x_test.reshape(x_test.shape[0],1,img_rows,img_cols) input_shape = (1,img_rows,img_cols) else: x_train = x_train.reshape(x_train.shape[0],img_rows,img_cols,1) x_test = x_test.reshape(x_test.shape[0],img_rows,img_cols,1) input_shape =(img_rows,img_cols,1) x_train =x_train.astype('float32') x_test = x_test.astype('float32') x_train /= 255 x_test /= 255 print('x_train shape:',x_train.shape) print(x_train.shape[0],'train samples') print(x_test.shape[0],'test samples')
代码中存在以下问题:
1. 变量 images 和 labels 没有被赋值,而后面却使用了它们来构造 X 和 y,导致 X 和 y 的值都为空。
2. 导入的 mnist 数据集没有被使用,而是使用了未定义的 images 和 labels,需要将其替换为 mnist 数据集。
3. input_shape 的值应该是三元组 (img_rows, img_cols, 1),而代码中缺少了最后一个 1。
4. 在 if K.image_data_format() =='channels_first': 分支中,x_train 和 x_test 被改变了形状,但 y_train 和 y_test 却没有被改变,需要将其同步修改。
下面是修改后的代码:
```
from __future__ import print_function
import numpy as np
import tensorflow
import keras
from keras.models import Sequential
from keras.layers import Dense, Dropout, Flatten
from keras.layers import Conv2D, MaxPooling2D
from keras import backend as K
import tensorflow as tf
import datetime
import os
np.random.seed(0)
from sklearn.model_selection import train_test_split
from PIL import Image
import matplotlib.pyplot as plt
from keras.datasets import mnist
(x_train, y_train), (x_test, y_test) = mnist.load_data()
print(x_train.shape)
print(y_train.shape)
############################
##########
batch_size = 20
num_classes = 10
learning_rate = 0.0001
epochs = 10
img_rows, img_cols = 32, 32
if K.image_data_format() == 'channels_first':
x_train = x_train.reshape(x_train.shape[0], 1, img_rows, img_cols)
x_test = x_test.reshape(x_test.shape[0], 1, img_rows, img_cols)
input_shape = (img_rows, img_cols, 1)
else:
x_train = x_train.reshape(x_train.shape[0], img_rows, img_cols, 1)
x_test = x_test.reshape(x_test.shape[0], img_rows, img_cols, 1)
input_shape = (img_rows, img_cols, 1)
y_train = keras.utils.to_categorical(y_train, num_classes)
y_test = keras.utils.to_categorical(y_test, num_classes)
x_train = x_train.astype('float32')
x_test = x_test.astype('float32')
x_train /= 255
x_test /= 255
print('x_train shape:', x_train.shape)
print(x_train.shape[0], 'train samples')
print(x_test.shape[0], 'test samples')
print('input_shape:', input_shape)
```
img = train_generator[0] print(img[0].shape) print(img[1].shape) 什么意思
这段代码是在使用一个名为`train_generator`的生成器对象,通过调用它的`__getitem__`方法获取了一个batch的数据,并将其赋值给了一个名为`img`的变量。`train_generator[0]`表示获取生成器的第一个batch数据。
`img`是一个包含两个元素的元组,第一个元素是一个形状为`(batch_size, height, width, channels)`的numpy数组,表示batch的图像数据,而第二个元素是一个形状为`(batch_size, num_classes)`的numpy数组,表示batch的标签数据。
`print(img[0].shape)`输出的是batch的图像数据的形状,即`(batch_size, height, width, channels)`,其中`batch_size`表示batch的大小,`height`表示图像的高度,`width`表示图像的宽度,`channels`表示图像的通道数。
`print(img[1].shape)`输出的是batch的标签数据的形状,即`(batch_size, num_classes)`,其中`batch_size`表示batch的大小,`num_classes`表示分类问题中的类别数。
相关推荐
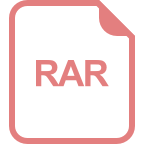













