手动完成MLP的反向传播进行训练pytorch代码
时间: 2024-02-11 12:06:14 浏览: 114
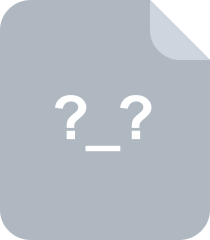
python实现线性回归的反向传播(以torch)
以下是手动完成MLP的反向传播进行训练的PyTorch代码:
```python
import torch
import torch.nn as nn
# 定义多层感知器模型
class MLP(nn.Module):
def __init__(self):
super(MLP, self).__init__()
self.layer1 = nn.Linear(2, 4) # 输入层到隐藏层
self.layer2 = nn.Linear(4, 1) # 隐藏层到输出层
self.activation = nn.Sigmoid() # 激活函数为Sigmoid
def forward(self, x):
x = self.layer1(x)
x = self.activation(x)
x = self.layer2(x)
x = self.activation(x)
return x
# 定义训练数据
x_train = torch.tensor([[0, 0], [0, 1], [1, 0], [1, 1]], dtype=torch.float32)
y_train = torch.tensor([[0], [1], [1], [0]], dtype=torch.float32)
# 初始化模型参数
model = MLP()
model.layer1.weight.data = torch.tensor([[0.1, 0.2], [0.3, 0.4], [0.5, 0.6], [0.7, 0.8]], dtype=torch.float32)
model.layer1.bias.data = torch.tensor([0.1, 0.2, 0.3, 0.4], dtype=torch.float32)
model.layer2.weight.data = torch.tensor([[0.9, 1.0, 1.1, 1.2]], dtype=torch.float32)
model.layer2.bias.data = torch.tensor([0.5], dtype=torch.float32)
# 定义损失函数和学习率
criterion = nn.BCELoss()
lr = 0.1
# 开始训练
epochs = 10000
for epoch in range(epochs):
# 前向传播
h = torch.matmul(x_train, model.layer1.weight.t()) + model.layer1.bias
h = model.activation(h)
y_pred = torch.matmul(h, model.layer2.weight.t()) + model.layer2.bias
y_pred = model.activation(y_pred)
# 计算损失和梯度
loss = criterion(y_pred, y_train)
grad_y_pred = (y_pred - y_train) / (y_pred * (1 - y_pred))
grad_layer2 = torch.matmul(grad_y_pred.t(), h)
grad_bias2 = torch.sum(grad_y_pred, dim=0)
grad_h = torch.matmul(grad_y_pred, model.layer2.weight)
grad_layer1 = torch.matmul(grad_h * h * (1 - h), x_train)
grad_bias1 = torch.sum(grad_h * h * (1 - h), dim=0)
# 更新参数
model.layer2.weight -= lr * grad_layer2
model.layer2.bias -= lr * grad_bias2
model.layer1.weight -= lr * grad_layer1
model.layer1.bias -= lr * grad_bias1
# 输出训练过程
if (epoch+1) % 1000 == 0:
print('Epoch [{}/{}], Loss: {:.4f}'.format(epoch+1, epochs, loss.item()))
# 测试模型
with torch.no_grad():
h = torch.matmul(x_train, model.layer1.weight.t()) + model.layer1.bias
h = model.activation(h)
y_pred = torch.matmul(h, model.layer2.weight.t()) + model.layer2.bias
y_pred = model.activation(y_pred)
y_pred = (y_pred > 0.5).float()
print('Predicted Values:', y_pred)
```
在这个例子中,我们定义了一个包含一个输入层、一个隐藏层和一个输出层的多层感知器。输入是一个2维的张量,输出是一个标量。我们使用Sigmoid作为激活函数,并使用二元交叉熵作为损失函数。我们手动初始化模型参数,并使用手动计算梯度的方式进行训练,每1000个epoch输出一次损失值。最后我们对模型进行测试,输出预测的结果。
阅读全文
相关推荐
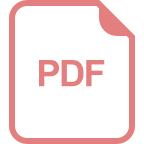
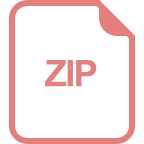
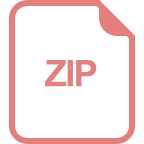
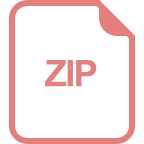
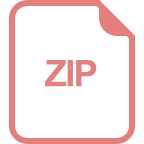
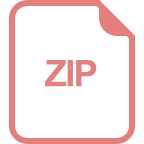
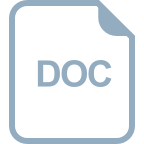
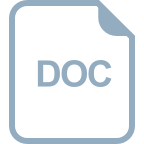
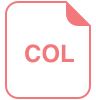
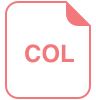
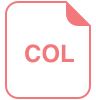
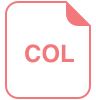
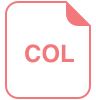



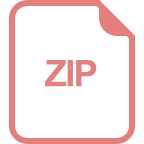